5G End-to-End Slice Embedding Based On Heterogeneous Graph Neural Network and Reinforcement Learning
IEEE Transactions on Cognitive Communications and Networking(2024)
摘要
Network slice embedding arranges multiple slices consisting of virtual network functions and their connections onto the shared substrate network. The embedding solution greatly affects the revenue for mobile network operators and service quality for slice tenants, making it an essential issue in the 5G and beyond era. To improve embedding quality, the algorithm must detect the holistic slice embedding status automatically, which is challenging due to the complex multidimensional information involved, including attributes of the substrate and slice networks, their topologies and their embedding relationships. However, most existing schemes lack automatic embedding solutions considering multidimensional information. Therefore, we propose a general end-to-end slice embedding scheme that can automatically extract multidimensional features of the embedding situation under constraints of realistic slice requirements. A heterogeneous graph neural network based encoder generates encoding vectors containing holistic information, which are then fed into a dueling network based decoder with variable output sizes to flexibly generate embedding decisions. The encoder and decoder are trained uniformly by reinforcement learning. Simulation results demonstrate that our proposed scheme outperforms schemes based on homogeneous GNN and some heuristics by generating higher accumulated revenues to MNOs with moderate embedding cost.
更多查看译文
关键词
5G slice embedding,graph neural network,reinforcement learning
AI 理解论文
溯源树
样例
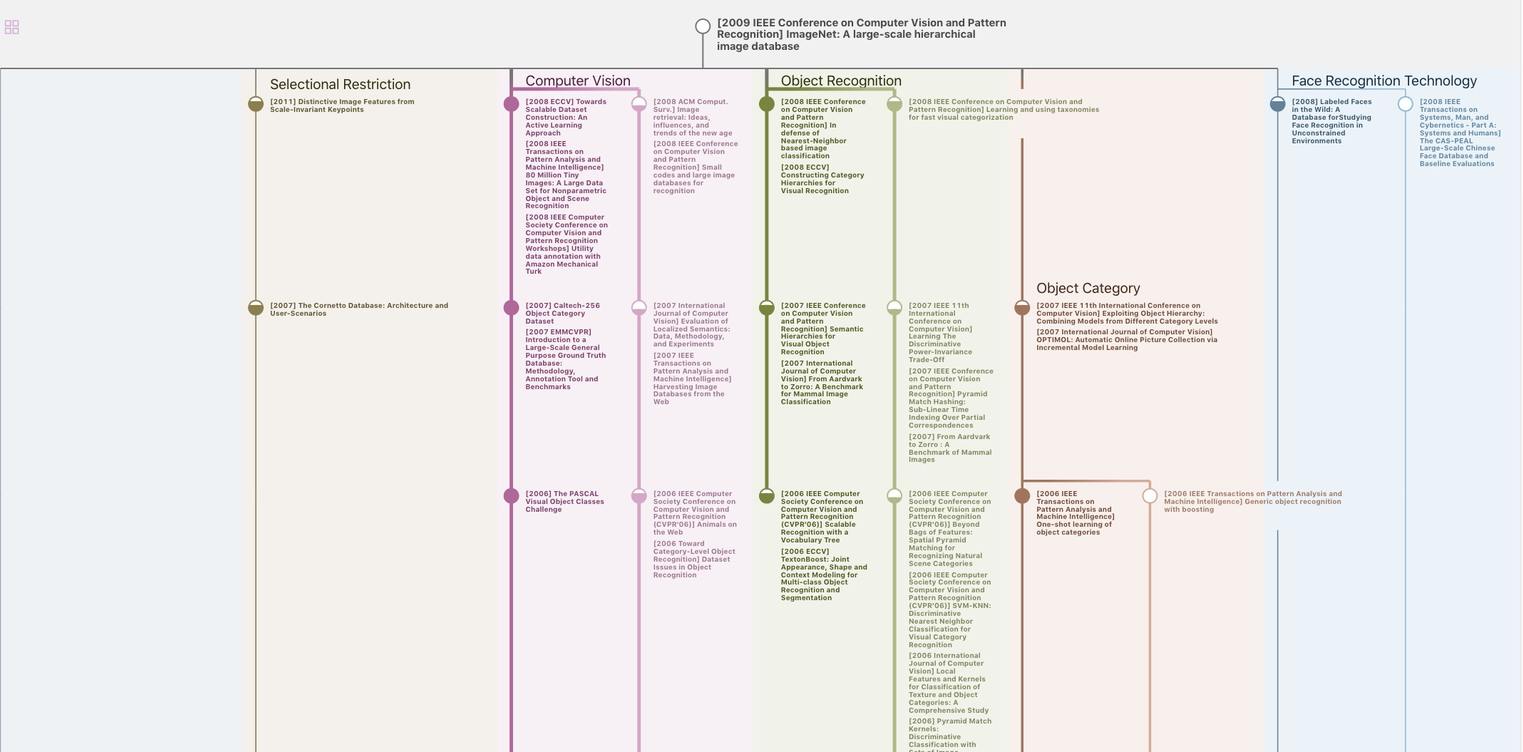
生成溯源树,研究论文发展脉络
Chat Paper
正在生成论文摘要