Monitoring response to neoadjuvant therapy for breast cancer in all treatment phases using an ultrasound deep learning model
FRONTIERS IN ONCOLOGY(2024)
Abstract
Purpose: The aim of this study was to investigate the value of a deep learning model (DLM) based on breast tumor ultrasound image segmentation in predicting pathological response to neoadjuvant chemotherapy (NAC) in breast cancer. Methods: The dataset contains a total of 1393 ultrasound images of 913 patients from Renmin Hospital of Wuhan University, of which 956 ultrasound images of 856 patients were used as the training set, and 437 ultrasound images of 57 patients underwent NAC were used as the test set. A U-Net-based end-to-end DLM was developed for automatically tumor segmentation and area calculation. The predictive abilities of the DLM, manual segmentation model (MSM), and two traditional ultrasound measurement methods (longest axis model [LAM] and dual-axis model [DAM]) for pathological complete response (pCR) were compared using changes in tumor size ratios to develop receiver operating characteristic curves. Results: The average intersection over union value of the DLM was 0.856. The early-stage ultrasound-predicted area under curve (AUC) values of pCR were not significantly different from those of the intermediate and late stages (p< 0.05). The AUCs for MSM, DLM, LAM and DAM were 0.840, 0.756, 0.778 and 0.796, respectively. There was no significant difference in AUC values of the predictive ability of the four models. Conclusion: Ultrasonography was predictive of pCR in the early stages of NAC. DLM have a similar predictive value to conventional ultrasound for pCR, with an add benefit in effectively improving workflow.
MoreTranslated text
Key words
breast cancer,ultrasound image,deep learning,neoadjuvant chemotherapy,pathological complete response
AI Read Science
Must-Reading Tree
Example
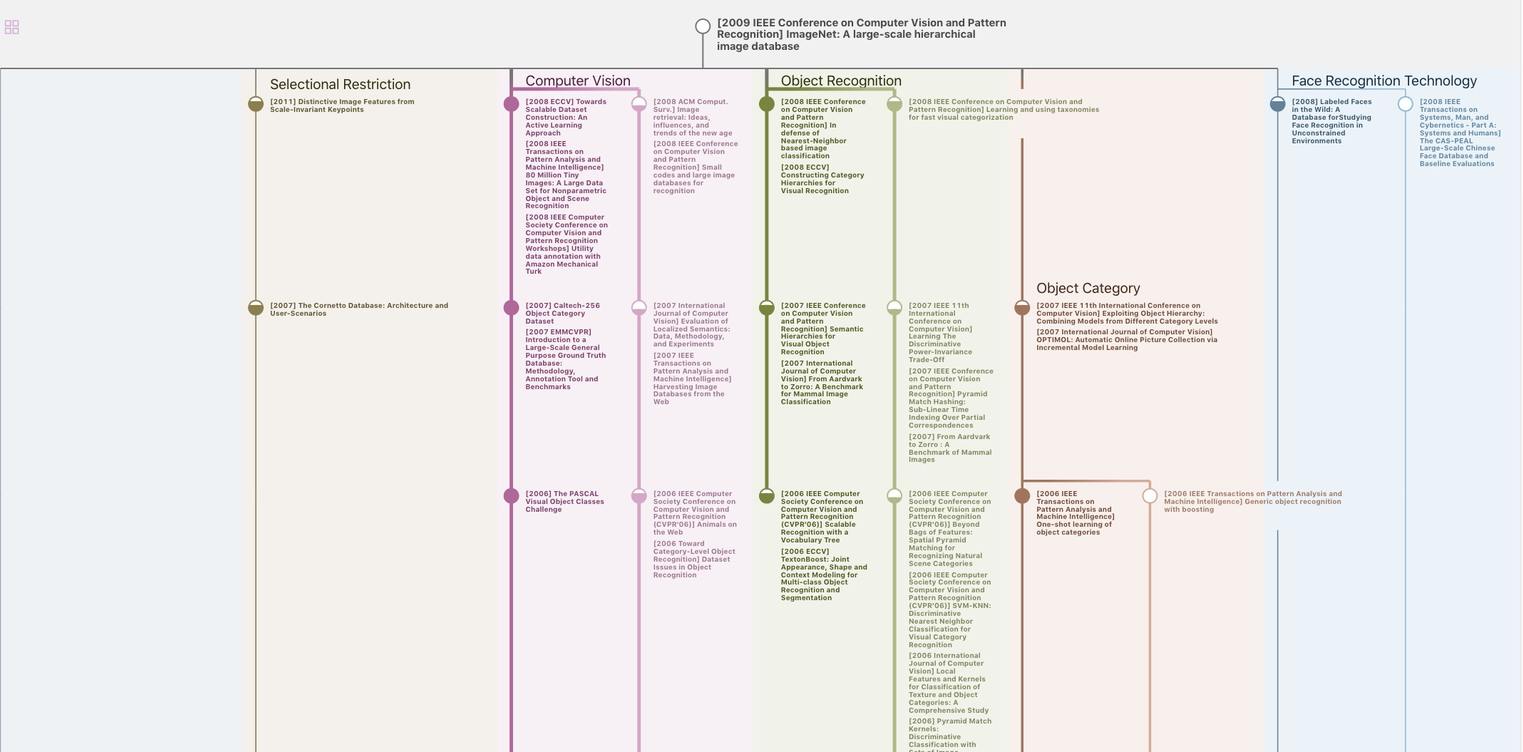
Generate MRT to find the research sequence of this paper
Chat Paper
Summary is being generated by the instructions you defined