Robustification of Bayesian-Inference-Based Gait Estimation for Lower-Limb Wearable Robots
IEEE ROBOTICS AND AUTOMATION LETTERS(2024)
摘要
Lower-limb wearable robots designed to assist people in everyday activities must reliably recover from any momentary confusion about what the user is doing. Such confusion might arise from momentary sensor failure, collision with an obstacle, losing track of gait due to an out-of-distribution stride, etc. Systems that infer a user's walking condition from angle measurements using Bayesian filters (e.g., extended Kalman filters) have been shown to accurately track gait across a range of activities. However, due to the fundamental problem structure and assumptions of Bayesian filter implementations, such estimators risk becoming 'lost' with little hope of a quick recovery. In this letter, we 1) introduce a Monte Carlo-based metric to quantify the robustness of pattern-tracking gait estimators, 2) propose strategies for improving tracking robustness, and 3) systematically evaluate them against this new metric using a publicly available gait biomechanics dataset. Our results, aggregating 2,700 trials of simulated walking of 10 able-bodied subjects under random perturbations, suggest that drastic improvements in robustness (from 8.9% to 99%) are possible using relatively simple modifications to the estimation process without noticeably degrading estimator accuracy.
更多查看译文
关键词
Thigh,Kinematics,Legged locomotion,Bayes methods,Robustness,Task analysis,Wearable robots,Prosthetics and exoskeletons,wearable robotics,rehabilitation robotics
AI 理解论文
溯源树
样例
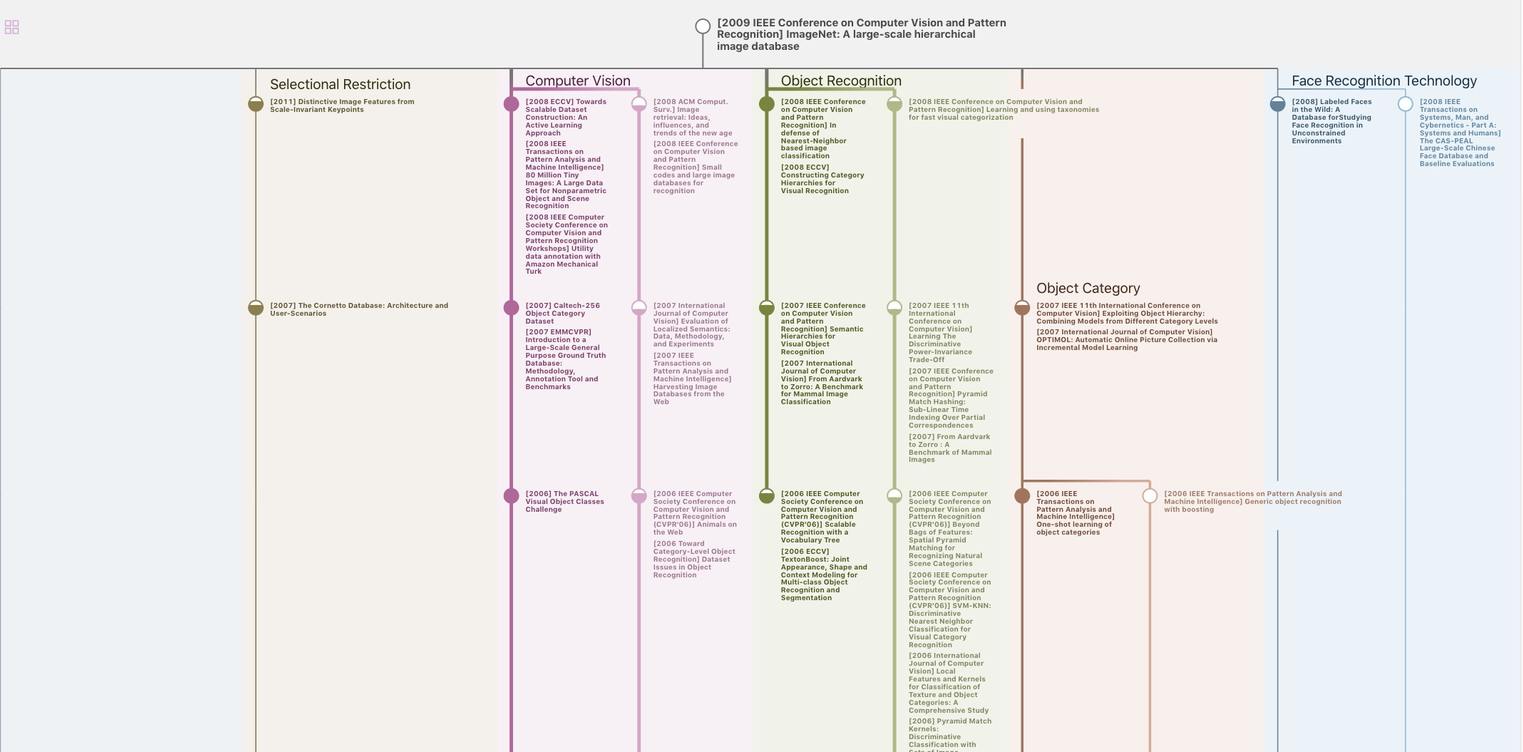
生成溯源树,研究论文发展脉络
Chat Paper
正在生成论文摘要