Faster and Lighter: A Novel Ship Detector for SAR Images
IEEE GEOSCIENCE AND REMOTE SENSING LETTERS(2024)
摘要
Benefiting from the rapid development of deep learning, the field of synthetic aperture radar (SAR) ship detection has been promoted with renewed vigor. However, due to the unique characteristics of SAR ship detection, several challenges have been encountered in the process of fusing SAR ship detection. On the one hand, some special properties of SAR images, including low resolution, small targets, dense ship inshore arrangements, and background clutter noise, increase the difficulty of detection; on the other hand, satellite detection requires a high degree of real-time and lightweight, and most traditional models fail to satisfy the requirements in two areas. After considering the above issues, we combine the advantages of space-to-depth convolution (SPDConv) and inception depthwise convolution (InceptionDWConv) to design SPD-InceptionDWConv (SIDConv), which resolves the above problems with fewer parameters and operations. Moreover, based on SIDConv, we also design light SIDConv (LSIDConv) and efficient SIDConv (ESIDConv) to further reduce the model complexity and to accelerate the detection process. With the above module as the core and YOLOv5 as the base, we propose a lighter and faster detector named LFer-Net, which has only 0.8M parameters and 1.9G FLOPs. Extensive experimental results on the SSDD and HRSID datasets confirm that our model is not only superior to other models with 98.2 and 90.6 accuracy but also achieves detection speeds of 144 and 96 frames per second (FPS), respectively.
更多查看译文
关键词
Faster,lighter,ship detection,synthetic aperture radar (SAR),you only look once (YOLO)
AI 理解论文
溯源树
样例
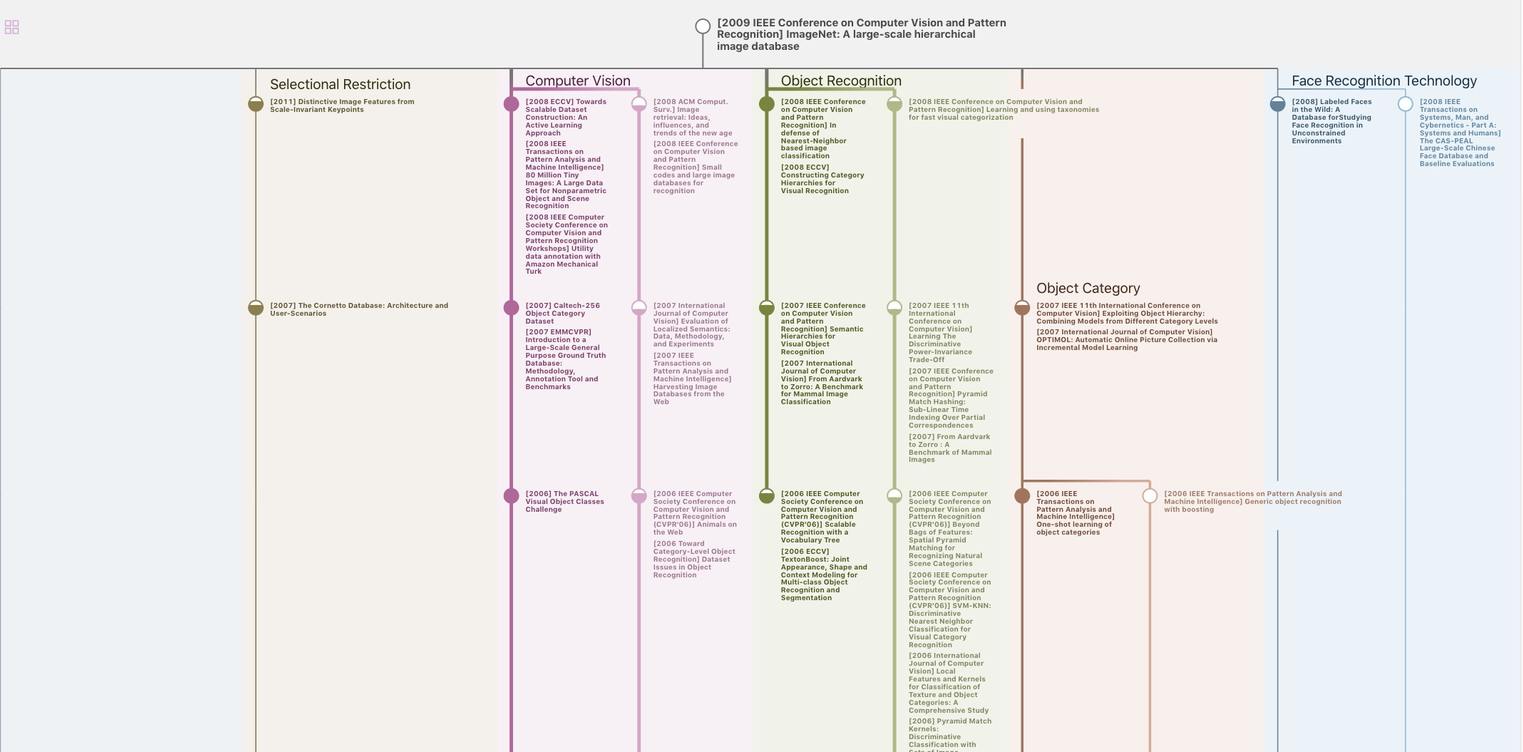
生成溯源树,研究论文发展脉络
Chat Paper
正在生成论文摘要