Time series prediction of sea surface temperature based on BiLSTM model with attention mechanism
JOURNAL OF SEA RESEARCH(2024)
摘要
With the advancement of technology, ocean observation techniques have become increasingly prevalent in estimating marine variables such as Sea Surface Temperature (SST). This progress has led to a substantial surge in the volume of marine data. Presently, the abundance of available data presents a remarkable opportunity for training predictive models. The prediction of SST poses a challenge due to its temporal-dependent structure and multi-level seasonality. In this study, we propose a deep learning approach that combines the Bidirectional Long Short-Term Memory (BiLSTM) model with the attention mechanism to forecast SST. By leveraging the BiLSTM's ability to effectively capture long-term dependencies through both forward and backward LSTM processing, the attention mechanism accentuates salient features, thereby enhancing the model's evaluation accuracy. To evaluate the effectiveness of the Attention-BiLSTM model in predicting SST, we conducted a case study in the Moroccan Sea, focusing on four distinct regions. We compared the performance of the Attention-BiLSTM model against alternative models such as LSTM, Attention-BiGRU, XGBoost, Random Forest (RF), Support Vector Regression (SVR), and Transformers in forecasting the SST time series. The experimental results unequivocally demonstrate that the Attention-BiLSTM model achieves significantly superior prediction outcomes and is a good candidate for deployment in the field.
更多查看译文
关键词
Bidirectional Long Short-Term Memory,(BiLSTM),Attention,Sea Surface Temperature (SST),Prediction,Marine data,Morocco
AI 理解论文
溯源树
样例
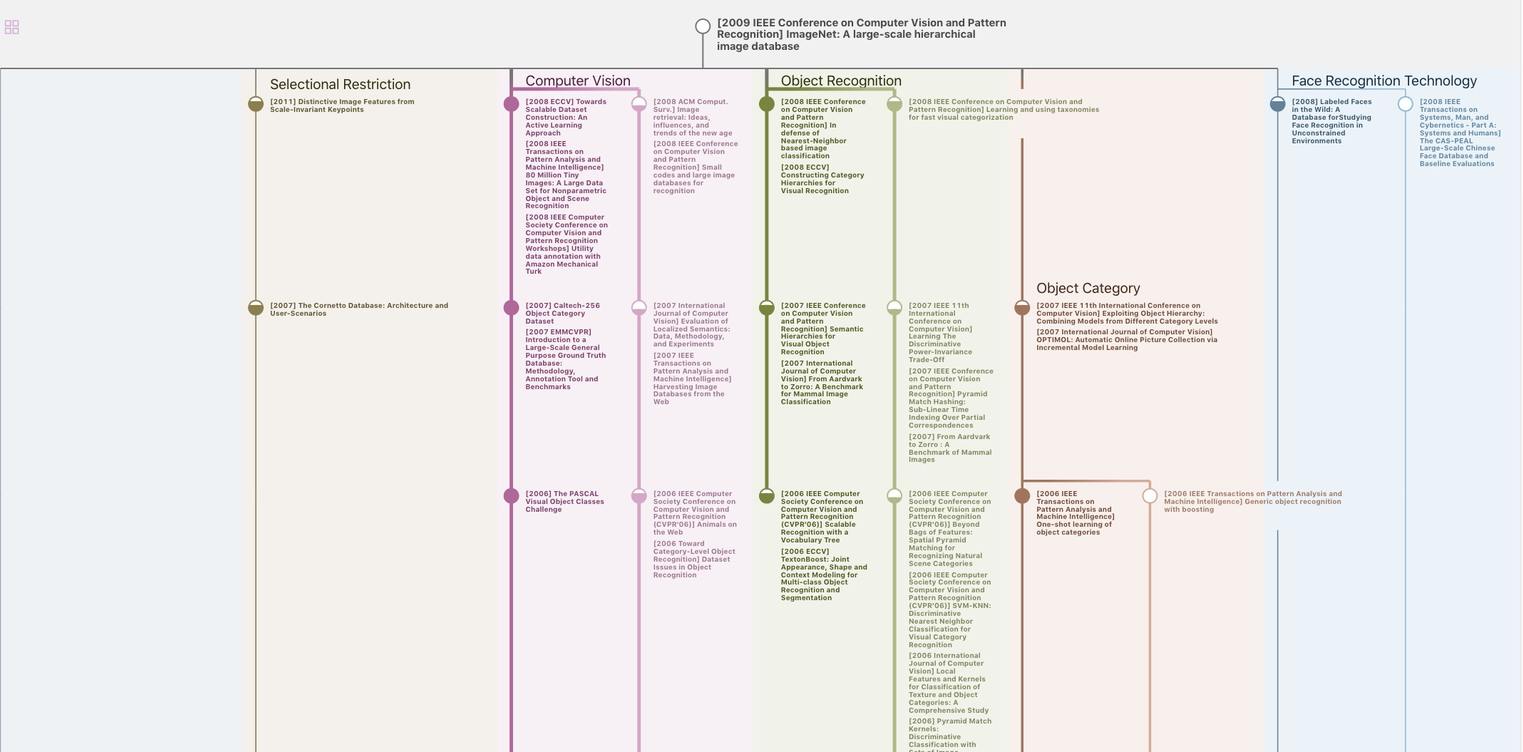
生成溯源树,研究论文发展脉络
Chat Paper
正在生成论文摘要