A Comprehensive View of the Biases of Toxicity and Sentiment Analysis Methods Towards Utterances with African American English Expressions
CoRR(2024)
摘要
Language is a dynamic aspect of our culture that changes when expressed in different technologies/communities. Online social networks have enabled the diffusion and evolution of different dialects, including African American English (AAE). However, this increased usage is not without barriers. One particular barrier is how sentiment (Vader, TextBlob, and Flair) and toxicity (Google's Perspective and the open-source Detoxify) methods present biases towards utterances with AAE expressions. Consider Google's Perspective to understand bias. Here, an utterance such as ``All n*ggers deserve to die respectfully. The police murder us.'' it reaches a higher toxicity than ``African-Americans deserve to die respectfully. The police murder us.''. This score difference likely arises because the tool cannot understand the re-appropriation of the term ``n*gger''. One explanation for this bias is that AI models are trained on limited datasets, and using such a term in training data is more likely to appear in a toxic utterance. While this may be plausible, the tool will make mistakes regardless. Here, we study bias on two Web-based (YouTube and Twitter) datasets and two spoken English datasets. Our analysis shows how most models present biases towards AAE in most settings. We isolate the impact of AAE expression usage via linguistic control features from the Linguistic Inquiry and Word Count (LIWC) software, grammatical control features extracted via Part-of-Speech (PoS) tagging from Natural Language Processing (NLP) models, and the semantic of utterances by comparing sentence embeddings from recent language models. We present consistent results on how a heavy usage of AAE expressions may cause the speaker to be considered substantially more toxic, even when speaking about nearly the same subject. Our study complements similar analyses focusing on small datasets and/or one method only.
更多查看译文
AI 理解论文
溯源树
样例
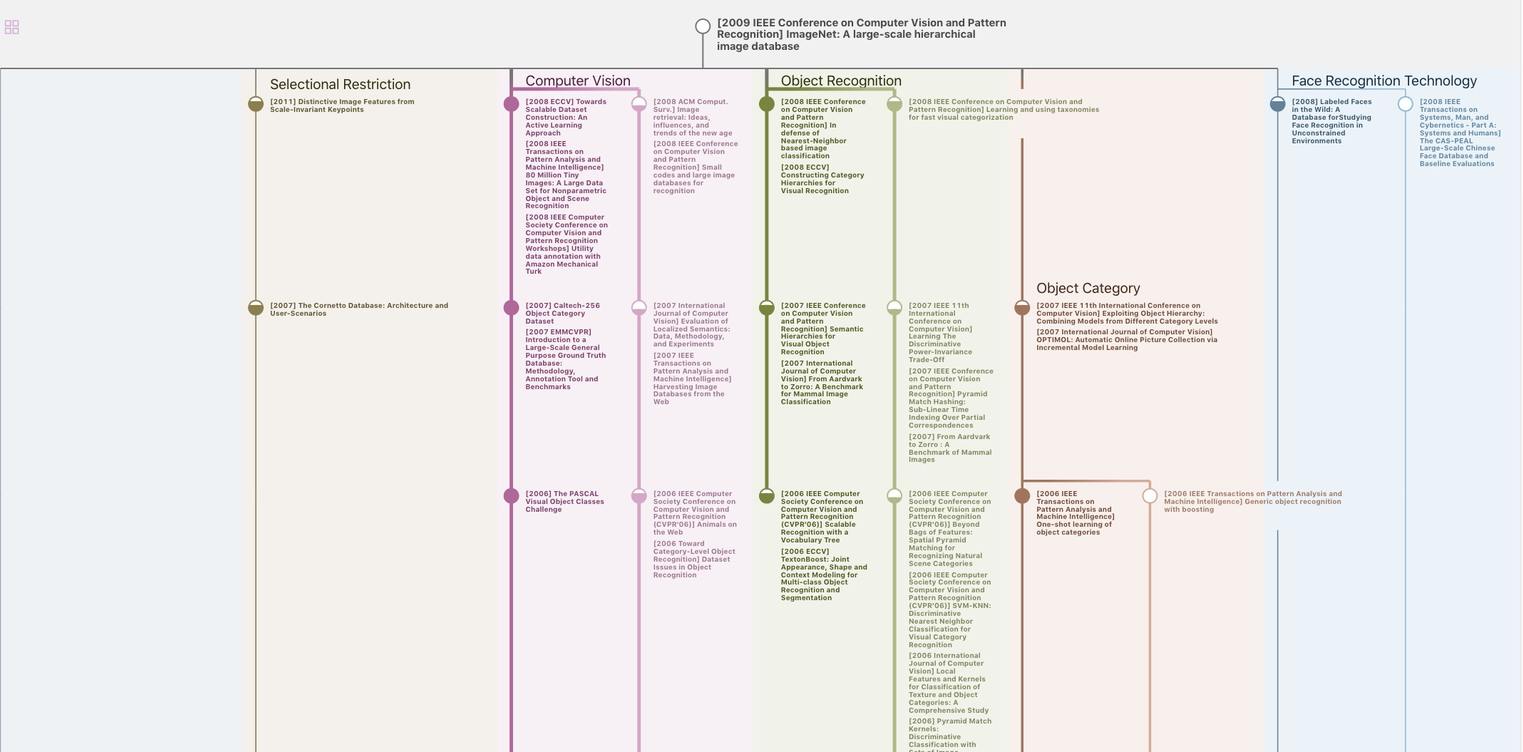
生成溯源树,研究论文发展脉络
Chat Paper
正在生成论文摘要