Stochastic Dynamic Power Dispatch with High Generalization and Few-Shot Adaption via Contextual Meta Graph Reinforcement Learning
CoRR(2024)
摘要
Reinforcement learning is an emerging approaches to facilitate multi-stage sequential decision-making problems. This paper studies a real-time multi-stage stochastic power dispatch considering multivariate uncertainties. Current researches suffer from low generalization and practicality, that is, the learned dispatch policy can only handle a specific dispatch scenario, its performance degrades significantly if actual samples and training samples are inconsistent. To fill these gaps, a novel contextual meta graph reinforcement learning (Meta-GRL) for a highly generalized multi-stage optimal dispatch policy is proposed. Specifically, a more general contextual Markov decision process (MDP) and scalable graph representation are introduced to achieve a more generalized multi-stage stochastic power dispatch modeling. An upper meta-learner is proposed to encode context for different dispatch scenarios and learn how to achieve dispatch task identification while the lower policy learner learns context-specified dispatch policy. After sufficient offline learning, this approach can rapidly adapt to unseen and undefined scenarios with only a few updations of the hypothesis judgments generated by the meta-learner. Numerical comparisons with state-of-the-art policies and traditional reinforcement learning verify the optimality, efficiency, adaptability, and scalability of the proposed Meta-GRL.
更多查看译文
AI 理解论文
溯源树
样例
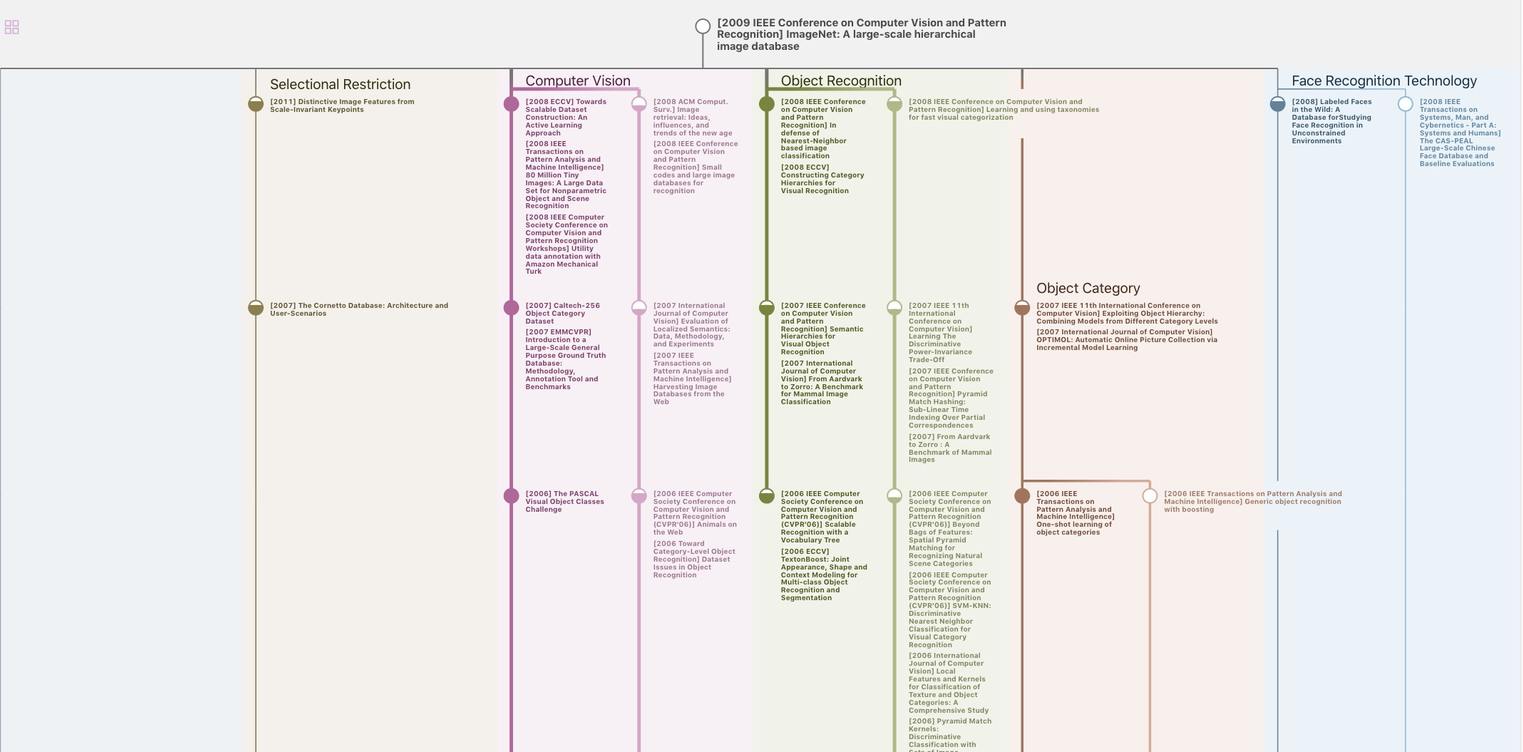
生成溯源树,研究论文发展脉络
Chat Paper
正在生成论文摘要