Multi-Scale-Matching neural networks for thin plate bending problem
THEORETICAL AND APPLIED MECHANICS LETTERS(2024)
摘要
Physics -informed neural networks (PINN) are a useful machine learning method for solving differential equations, but encounter challenges in effectively learning thin boundary layers within singular perturbation problems. To resolve this issue, Multi -Scale -Matching Neural Networks (MSM-NN) are proposed to solve the singular perturbation problems. Inspired by matched asymptotic expansions, the solution is decomposed into inner solutions for small scales and outer solutions for large scales, corresponding to boundary layers and outer regions, respectively. Moreover, to conform neural networks, we introduce exponential stretched variables in the boundary layers to avoid semi -infinite region problems. Numerical results for the thin plate problem validate the proposed method.
更多查看译文
关键词
Singular perturbation,Physics-informed neural networks,Boundary layer,Machine learning
AI 理解论文
溯源树
样例
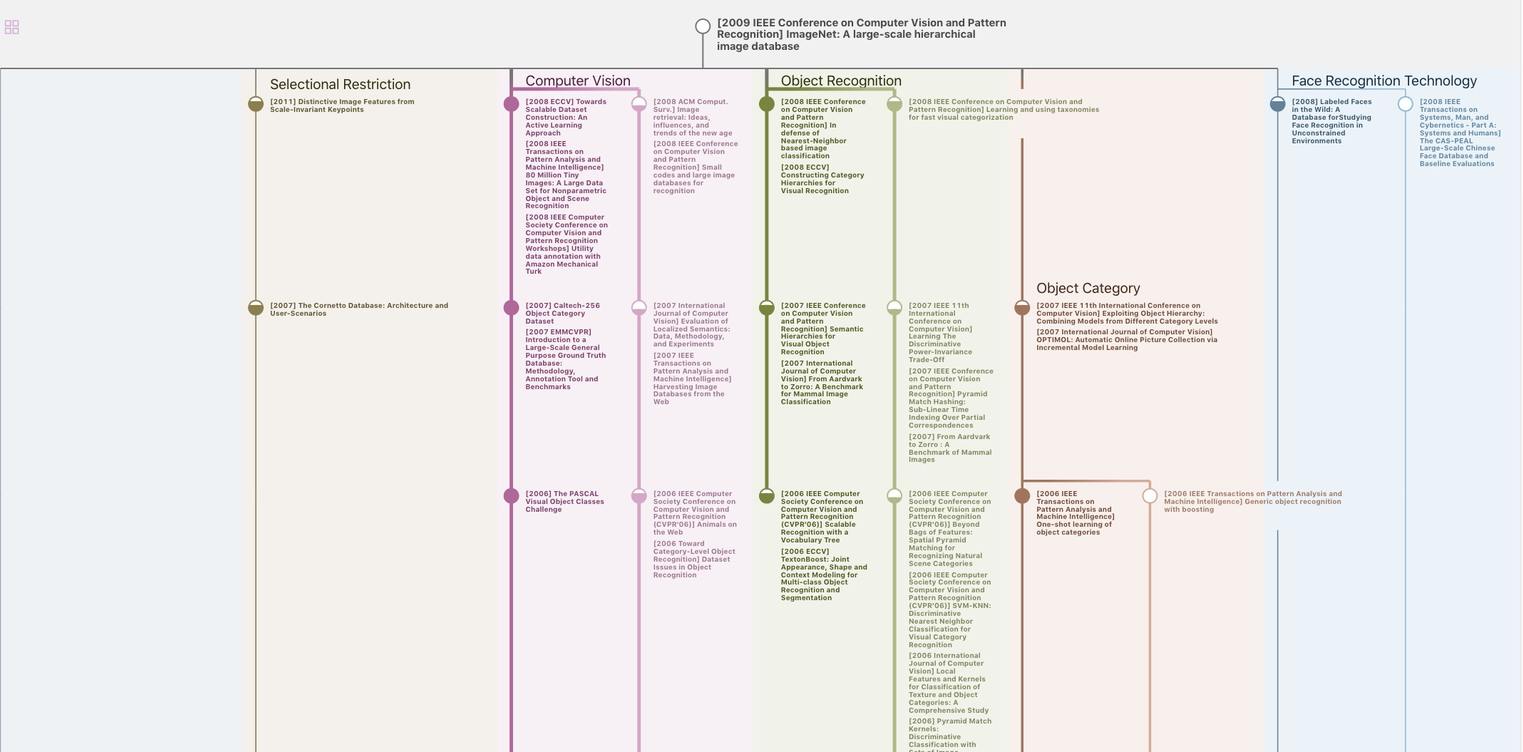
生成溯源树,研究论文发展脉络
Chat Paper
正在生成论文摘要