A machine learning approach to uncover nicotinamide and other antioxidants as novel markers for chicken meat quality assessment
FOOD BIOSCIENCE(2024)
摘要
This study analyses various chicken cuts (breast, leg, and thigh) in terms of ten biocompounds: nicotinamide, anserine, carnosine, malondialdehyde, and biogenic amines (putrescine, cadaverine, histamine, tyramine, spermidine, and spermine). The analysis is conducted on refrigerated chicken meat cuts using three different packaging solutions: modified atmosphere packaging (MAP), vacuum skin packaging (SKIN), and permeable O2 plastic film (STRETCH). Our results show that nicotinamide was the most discriminant compound followed for cuts and time. Remarkably, its concentration decreases over time, unaffected by any of the packaging solutions. The collective contribution of all the examined biocompounds is highlighted through multivariate statistical analysis, utilizing partial least square discriminant analysis (PLS-DA) and the support vector machine (SVM) algorithm. Both machine learning models demonstrate high classification accuracy: for cut, storage time, and packaging, PLS-DA yields classification accuracy rates of 87%, 85%, and 70%, respectively. SVM achieves even higher accuracy rates of 97%, 99%, and 94% for cut, storage time, and packaging, respectively. These results underscore the importance of considering the combined influence of all the biocompounds investigated in this study for a finer classification of chicken meat cuts and packaging. Furthermore, our findings highlight the efficacy of SVM as a discriminant multivariate approach in food classification.
更多查看译文
关键词
Packaged chicken meat,Nicotinamide,Carnosine,Anserine,Biogenic amines,Malondialdehyde,PLS-DA,SVM
AI 理解论文
溯源树
样例
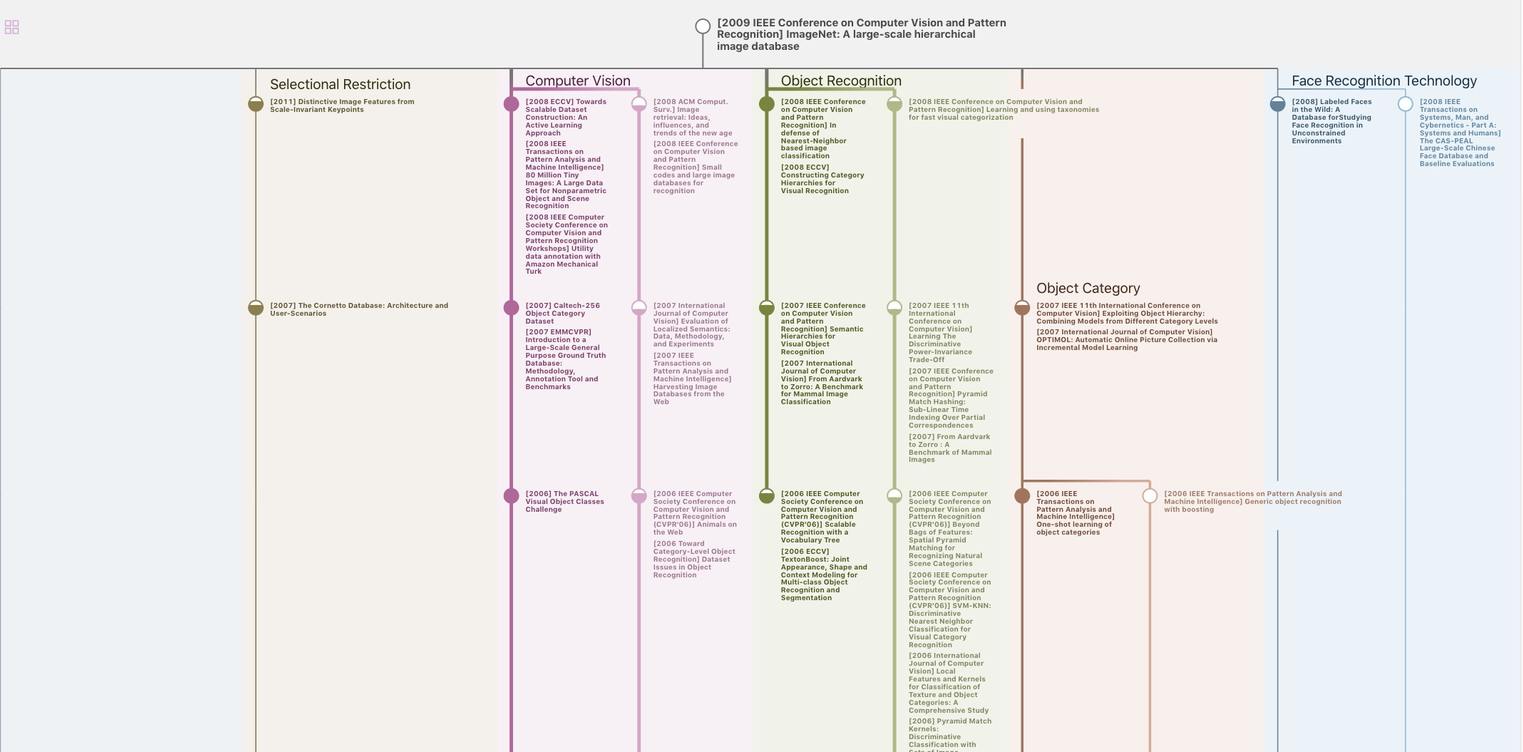
生成溯源树,研究论文发展脉络
Chat Paper
正在生成论文摘要