Data-fused and concatenated-ensemble learning for in-situ anomaly detection in wire and arc-based direct energy deposition
JOURNAL OF MANUFACTURING PROCESSES(2024)
摘要
Convolutional neural network (CNN), a type of deep learning algorithm, is a powerful tool for analyzing visual images. It has been actively investigated to monitor metal additive manufacturing (AM) processes for quality control and has been proven effective. However, typical CNN algorithms inherently have two issues when used in metal AM processes. First, in many cases, acquiring datasets with sufficient quantity and quality, as well as necessary information, is challenging because of technical difficulties and/or cost intensiveness. Second, determining a near-optimal CNN model takes considerable effort and is time-consuming. This is because the types and quality of datasets can be significantly different with respect to different AM processes and materials. The study proposes a novel concatenated ensemble learning method to obtain a flexible and robust algorithm for in-situ anomaly detection in wire + arc additive manufacturing (WAAM), a type of wire-based direct energy deposition (DED) process. For this, data, as well as machine learning models, were seamlessly integrated to overcome the limitations and difficulties in acquiring sufficient data and finding a near-optimal machine learning model. Using inexpensively obtainable and comprehensive datasets from the WAAM process, the proposed method was investigated and validated. In contrast to the one-dimensional and two-dimensional CNN models' accuracies of 81.6 % and 88.6 %, respectively, the proposed concatenated ensemble model achieved an accuracy of 98 %.
更多查看译文
关键词
Direct energy deposition,Anomaly detection,Wire plus arc additive manufacturing,Concatenated ensemble learning
AI 理解论文
溯源树
样例
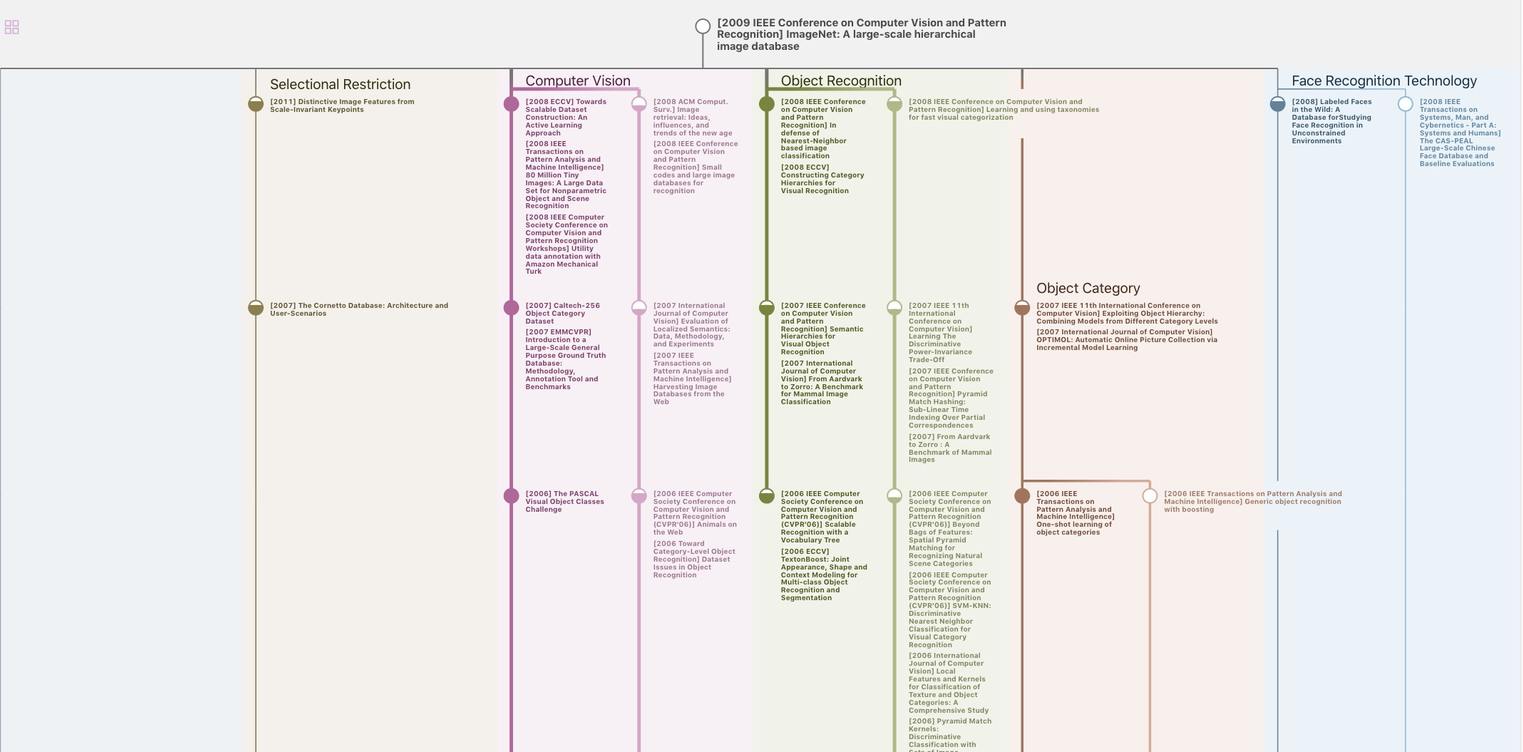
生成溯源树,研究论文发展脉络
Chat Paper
正在生成论文摘要