Predicting Linguistically Sophisticated Social Determinants of Health Disparities with Neural Networks: The Case of LGBTQ+ Minority Stress.
2023 IEEE International Conference on Big Data (BigData)(2023)
摘要
LGBTQ+ minority stress is a pervasive form of anti-LGBTQ+ adverse events and psychological strain that drives health inequities among LGBTQ+ people. Minority stress is also linguistically sophisticated (e.g., composed of cultural idioms, psycholinguistic permutations, and lexical density). Because minority stress is a linguistically sophisticated social determinant of health disparities, it is challenging to detect using natural language processing (NLP). Using 5,789 human-annotated Reddit posts from the LGBTQ+ Minority Stress on Social Media (MiSSoM+) Dataset, we investigated and compared the performance of four neural networks and two traditional machine learning architectures in modeling minority stress at both the factor (i.e., separate components of minority stress) and composite level. A novel hybrid model combining Bidirectional Encoder Representations from Transformers and convolutional neural network (BERT-CNN) improved the prediction of composite minority stress (F1 = 0.84). Our experiments on separate factors of minority stress are the first to demonstrate that hybrid neural network models can detect semantically complex expressions of prejudiced events (F1 = 0.87), expected rejection (F1 = 0.92), internalized stigma (F1 = 0.91), identity concealment (F1 = 0.92), and minority coping (F1 = 0.84). We also substantially improved the prediction of gender dysphoria (F1 = 0.94)—a conceptually new candidate component of minority stress. Big data analytics may not be a panacea for the problem of minority stress, but our work joins a growing literature base to show that deep learning models are remarkable in detecting linguistically sophisticated social determinants of health disparities in big data, thus providing evidence in support of the potential benefit from the innovative use of such technology in eliminating group-specific health inequities.
更多查看译文
关键词
sexual and gender minority,multi-label text classification,deep learning,word embedding,bidirectional encoder representation of transformers (BERT),convolutional neural network (CNN)
AI 理解论文
溯源树
样例
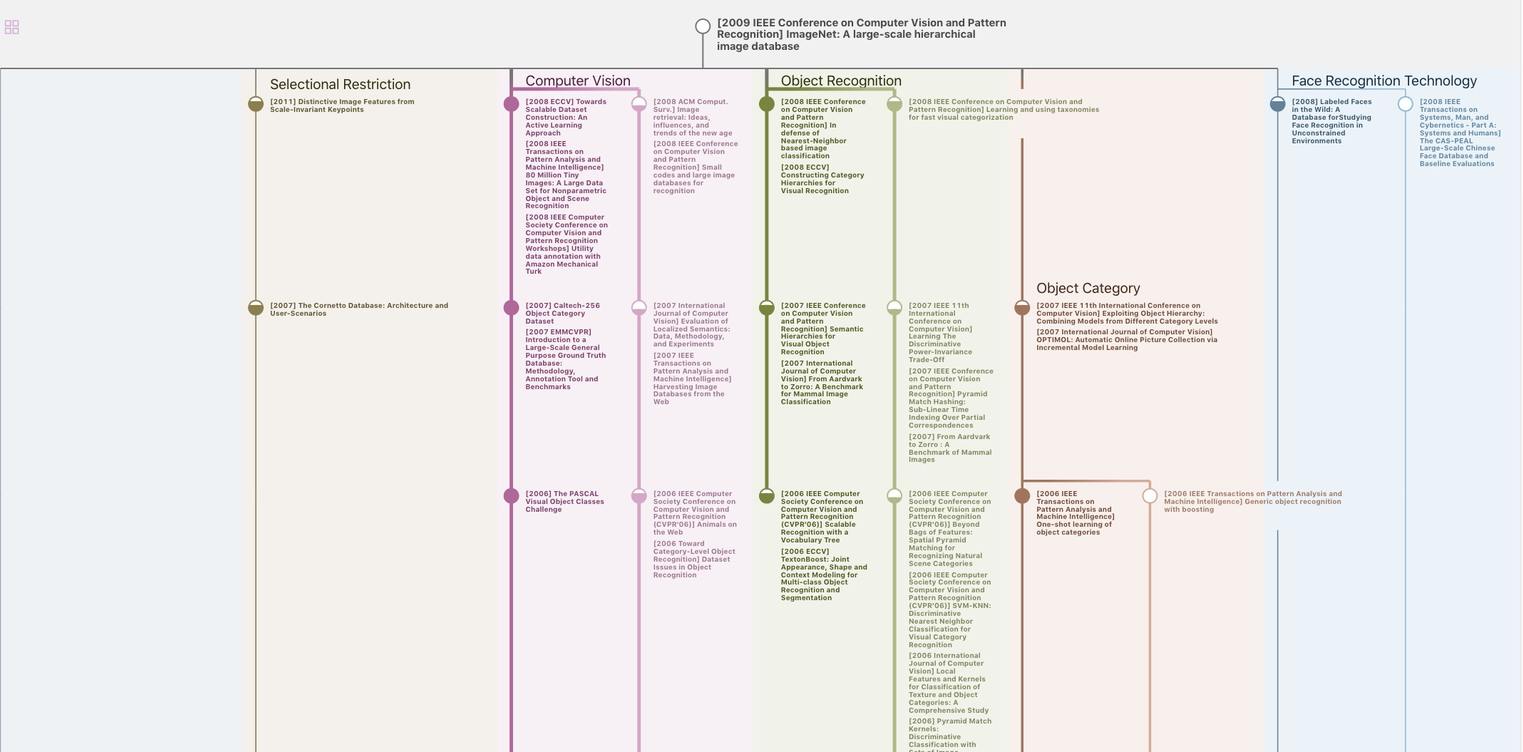
生成溯源树,研究论文发展脉络
Chat Paper
正在生成论文摘要