AQUA: A Framework for Spatiotemporal Analysis and Visualizations of Water Quality Data at Scale.
2023 IEEE International Conference on Big Data (BigData)(2023)
Abstract
Spatia1 data volumes have grown exponentially alongside the proliferation of sensing equipment and networked observational devices. In this study, we describe our framework aQua for performing visualizations and exploration of spatiotemporally evolving phenomena at scale. We validate our ideas in the context of data from the National Hydrology Database (NHD) and the Environmental Protection Agency (EPA) to support longitudinal analysis (53 years of data) for the vast majority of water bodies in the United States. Our methodology addresses issues relating to preserving interactivity, effective analysis, GPU accelerated visualizations, dynamic query generation, and scaling. We consider optimizations and refinements at the server-side, client-side, and how information exchange occurs between the client and server-side. We report both quantitative and qualitative assessments of several aspects of our tool to demonstrate its suitability. Finally, our methodology is broadly applicable to domains where visualization-driven explorations of spatiotemporally evolving phenomena are needed.
MoreTranslated text
Key words
Spatiotemporal Phenomena,Big Data,Visualizations,and High Dimensional Analyses
AI Read Science
Must-Reading Tree
Example
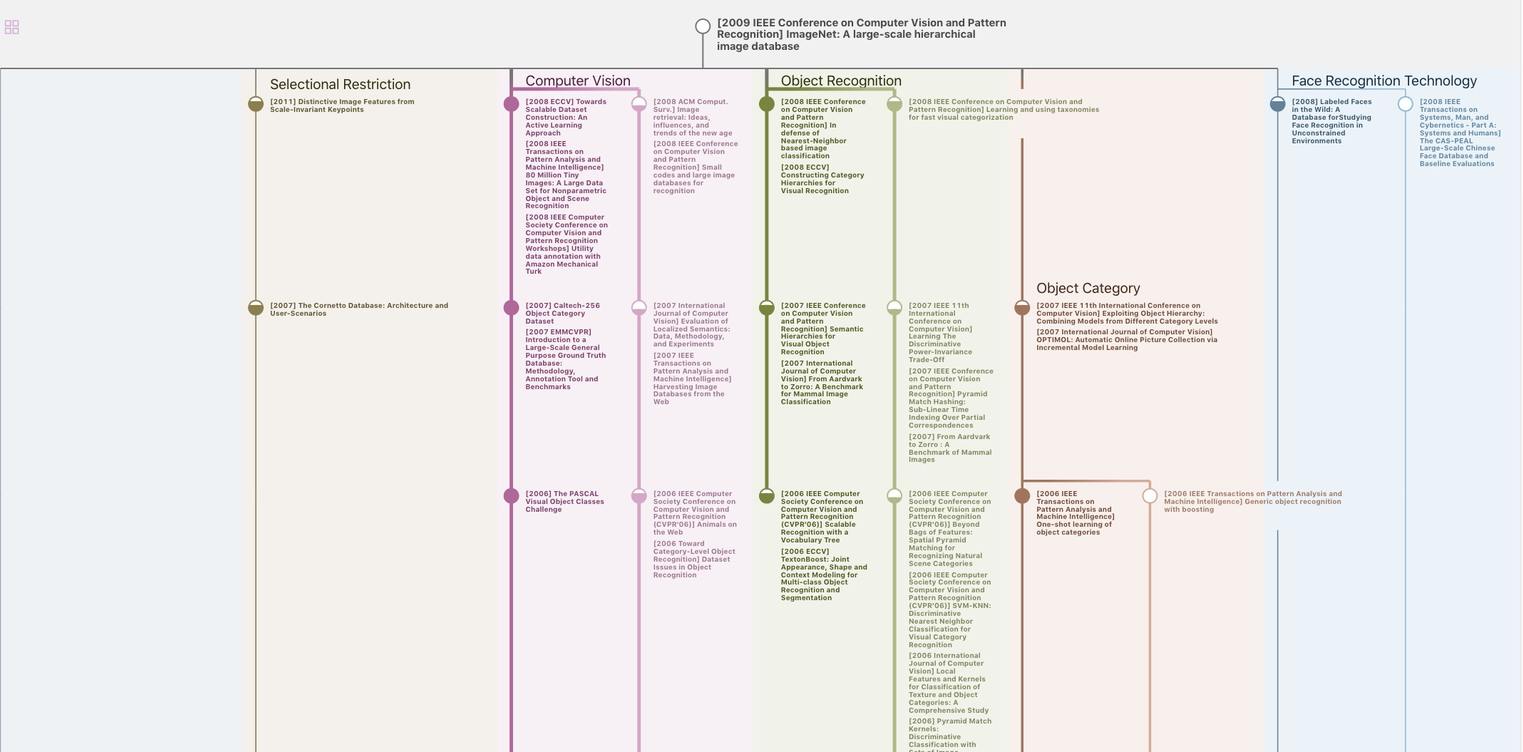
Generate MRT to find the research sequence of this paper
Chat Paper
Summary is being generated by the instructions you defined