DODGE: Automated point source bacterial outbreak detection using cumulative long term genomic surveillance.
medrxiv(2024)
摘要
The reliable and timely recognition of outbreaks is a key component of public health surveillance for foodborne diseases. Whole genome sequencing (WGS) offers high resolution typing of foodborne bacterial pathogens and facilitates the accurate detection of outbreaks. This detection relies on grouping WGS data into clusters at an appropriate genetic threshold, however, methods and tools for selecting and adjusting such thresholds according to the required resolution of surveillance and epidemiological context are lacking. Here we present DODGE (Dynamic Outbreak Detection for Genomic Epidemiology), an algorithm to dynamically select and compare these genetic thresholds. DODGE can analyse expanding datasets over time and clusters that are predicted to correspond to outbreaks (or investigation clusters) can be named with the established genomic nomenclature systems to facilitate integrated analysis across jurisdictions. DODGE was tested in two real-world genomic surveillance datasets of different duration, two months from Australia and nine years from the UK. In both cases only a minority of isolates were identified as investigation clusters. Two known outbreaks in the UK dataset were detected by DODGE and were recognised at an earlier timepoint than the outbreaks were reported. These findings demonstrated the potential of the DODGE approach to improve the effectiveness and timeliness of genomic surveillance for foodborne diseases and the effectiveness of the algorithm developed. Availability and implementation: DODGE is freely available at https://github.com/LanLab/dodge and can easily be installed using Conda.
### Competing Interest Statement
The authors have declared no competing interest.
### Funding Statement
This work was supported by The National Health and Medical Research Council: [Grant Number 1146938].
### Author Declarations
I confirm all relevant ethical guidelines have been followed, and any necessary IRB and/or ethics committee approvals have been obtained.
Yes
I confirm that all necessary patient/participant consent has been obtained and the appropriate institutional forms have been archived, and that any patient/participant/sample identifiers included were not known to anyone (e.g., hospital staff, patients or participants themselves) outside the research group so cannot be used to identify individuals.
Yes
I understand that all clinical trials and any other prospective interventional studies must be registered with an ICMJE-approved registry, such as ClinicalTrials.gov. I confirm that any such study reported in the manuscript has been registered and the trial registration ID is provided (note: if posting a prospective study registered retrospectively, please provide a statement in the trial ID field explaining why the study was not registered in advance).
Yes
I have followed all appropriate research reporting guidelines, such as any relevant EQUATOR Network research reporting checklist(s) and other pertinent material, if applicable.
Yes
All data produced in the present work are contained in the manuscript and at https://github.com/LanLab/dodge
更多查看译文
AI 理解论文
溯源树
样例
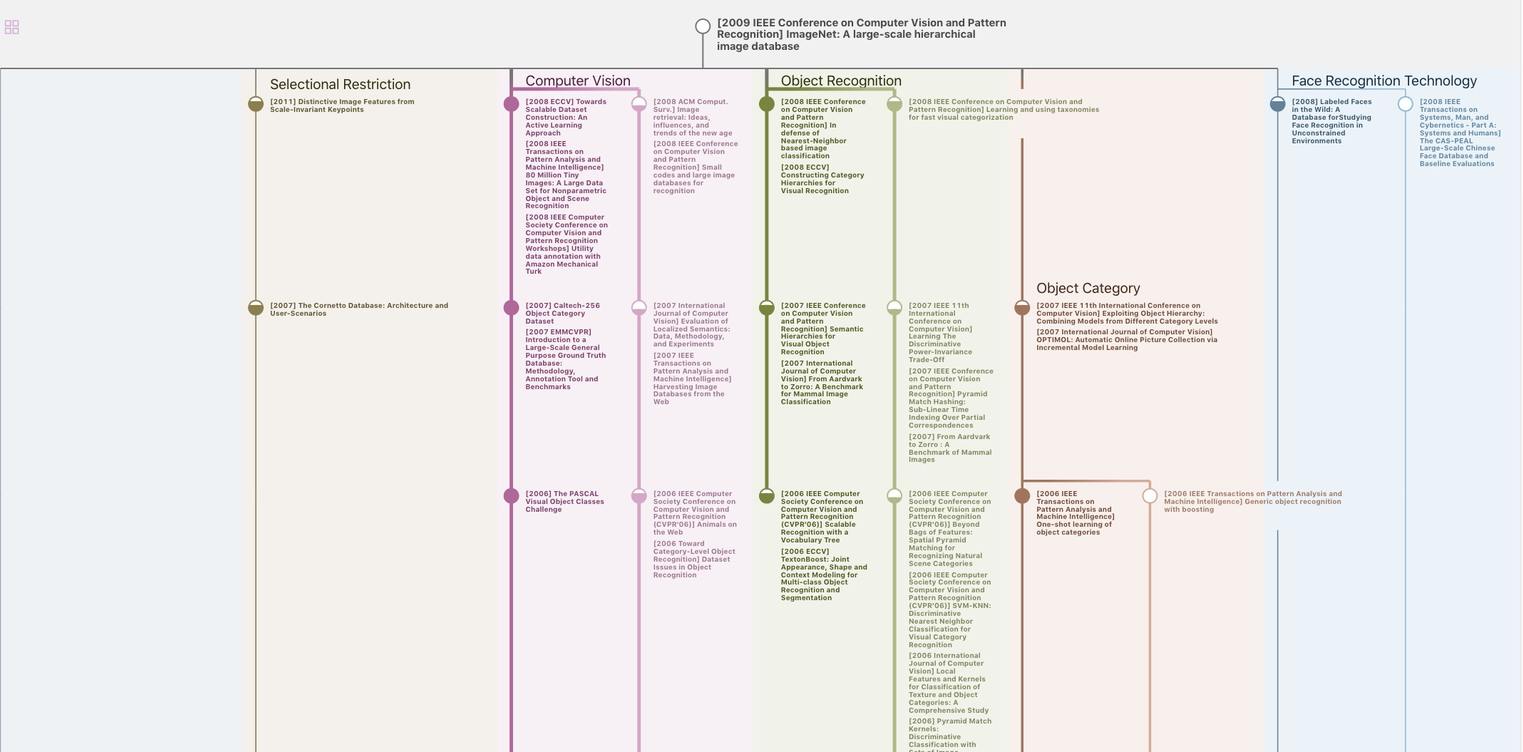
生成溯源树,研究论文发展脉络
Chat Paper
正在生成论文摘要