Easing accessibility to mass spectrometry imaging using convolutional autoencoders for deriving hypoxia-associated peptide candidates from tumors
crossref(2024)
Abstract
Abstract Spatial omics data promise to reveal novel insights into the intratumoral heterogenity of biological parameters of cancer. Mass spectrometry imaging (MSI) allows to study spatially-resolved peptides, metabolites and lipids. Yet, in biomedical research the technology is rarely used for biomarker discovery. Like all spatial omics data, its high dimensionality and multicollinearity imposes special challenges for analysis. Additionally, mass spectrometry technologies typically output mass-to-charge ratio values but not the biochemical compounds of interest. We propose a framework that addresses both issues to make MSI more accessible. We utilized convolutional autoencoders to aggregate intratumoral features of interest from MSI of cancer xenograft models. Exemplified for the parameter tumor hypoxia, which is known to show significant spatial heterogeneity, we highlight that MSI is able to capture these small intensity signals and that autoencoders can preserve them in a lower dimensional space. Complementing MSI with tandem MS data from the same tumor model, multiple promising peptide candidates were derived that were associated with hypoxia. Compared to random forest models, we showed that our autoencoder approach produced more plausible candidates. Considering other spatial omics modalities, the architecture of the convolutional autoencoder can be easily adapted while ensuring high explainability.
MoreTranslated text
AI Read Science
Must-Reading Tree
Example
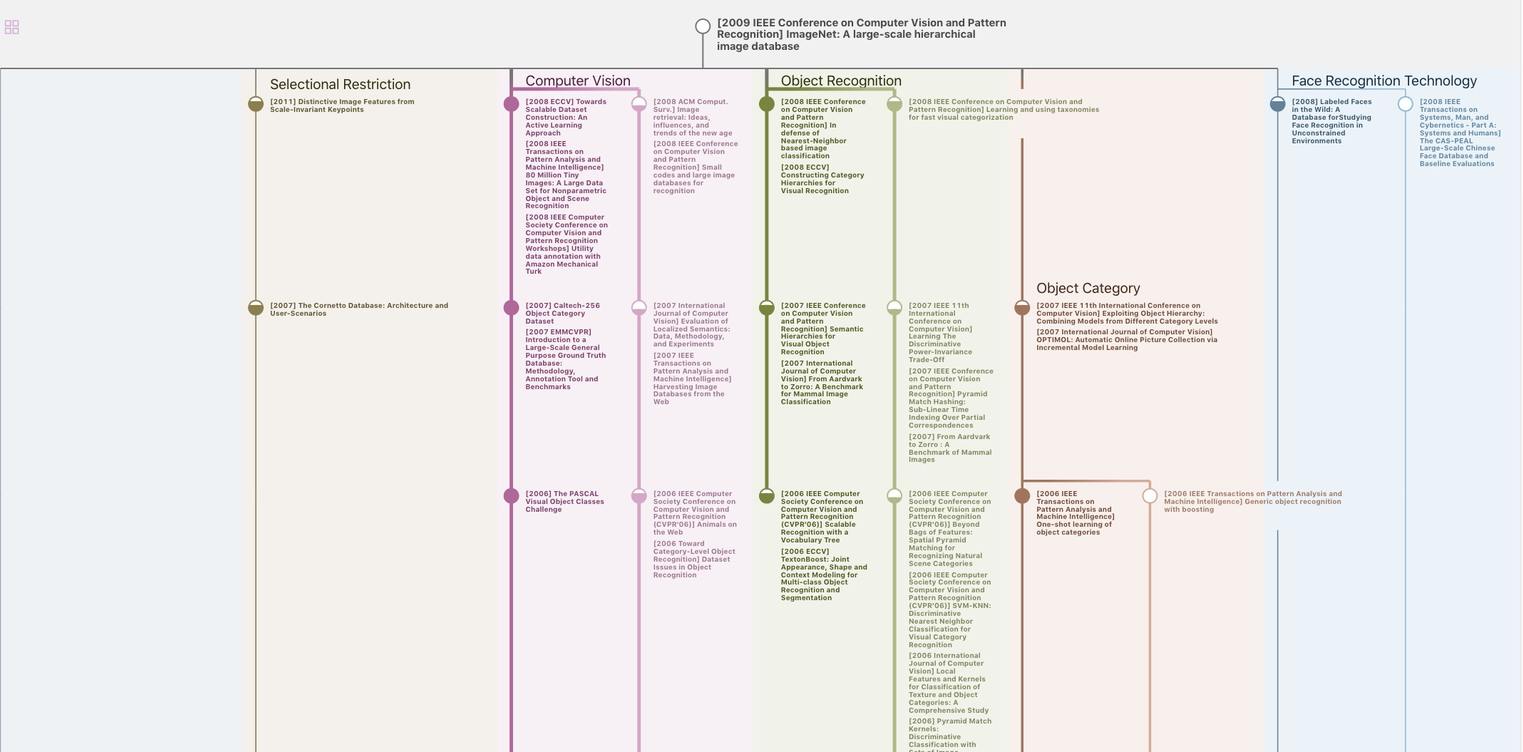
Generate MRT to find the research sequence of this paper
Chat Paper
Summary is being generated by the instructions you defined