Discriminative boundary generation for effective outlier detection
Knowledge and Information Systems(2024)
摘要
Outlier detection is often considered a challenge due to the inherent class imbalance in datasets, with the small number of available outliers that are insufficient to describe their overall distribution. This makes it difficult for classifiers to effectively learn the demarcation (boundary) between normal samples and outliers, which is the key for accurate detection. In this paper, we propose a novel discriminative boundary generation framework, called BoG. The framework extracts the border samples in the dataset and expands them to form the initial boundary outliers. With the adversarial training in GAN, the boundary outliers are further augmented, which, together with the boundary normal data, provides the valuable demarcation information for the classifier. Two method variants are proposed under our BoG framework to achieve a balance between detection efficiency and effectiveness. Extensive experiments show that our proposed framework achieves significant improvements compared to the existing outlier detection methods.
更多查看译文
关键词
Outlier detection,Boundary generation,GAN
AI 理解论文
溯源树
样例
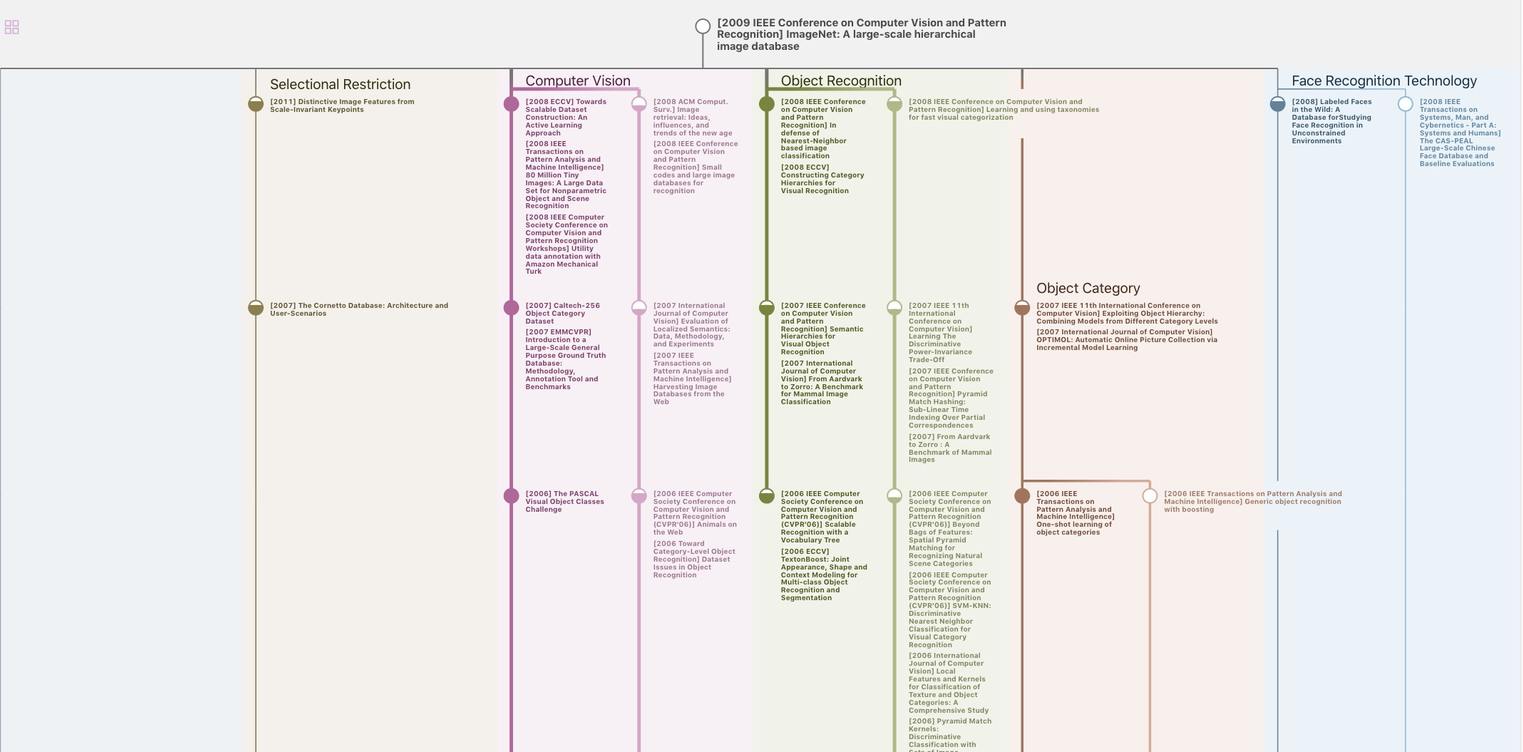
生成溯源树,研究论文发展脉络
Chat Paper
正在生成论文摘要