Tpgen: a language model for stable protein design with a specific topology structure
BMC Bioinformatics(2024)
摘要
Background Natural proteins occupy a small portion of the protein sequence space, whereas artificial proteins can explore a wider range of possibilities within the sequence space. However, specific requirements may not be met when generating sequences blindly. Research indicates that small proteins have notable advantages, including high stability, accurate resolution prediction, and facile specificity modification. Results This study involves the construction of a neural network model named TopoProGenerator(TPGen) using a transformer decoder. The model is trained with sequences consisting of a maximum of 65 amino acids. The training process of TopoProGenerator incorporates reinforcement learning and adversarial learning, for fine-tuning. Additionally, it encompasses a stability predictive model trained with a dataset comprising over 200,000 sequences. The results demonstrate that TopoProGenerator is capable of designing stable small protein sequences with specified topology structures. Conclusion TPGen has the ability to generate protein sequences that fold into the specified topology, and the pretraining and fine-tuning methods proposed in this study can serve as a framework for designing various types of proteins.
更多查看译文
关键词
De novo protein design,Protein topologies,Transformer,LSTM,Neural network
AI 理解论文
溯源树
样例
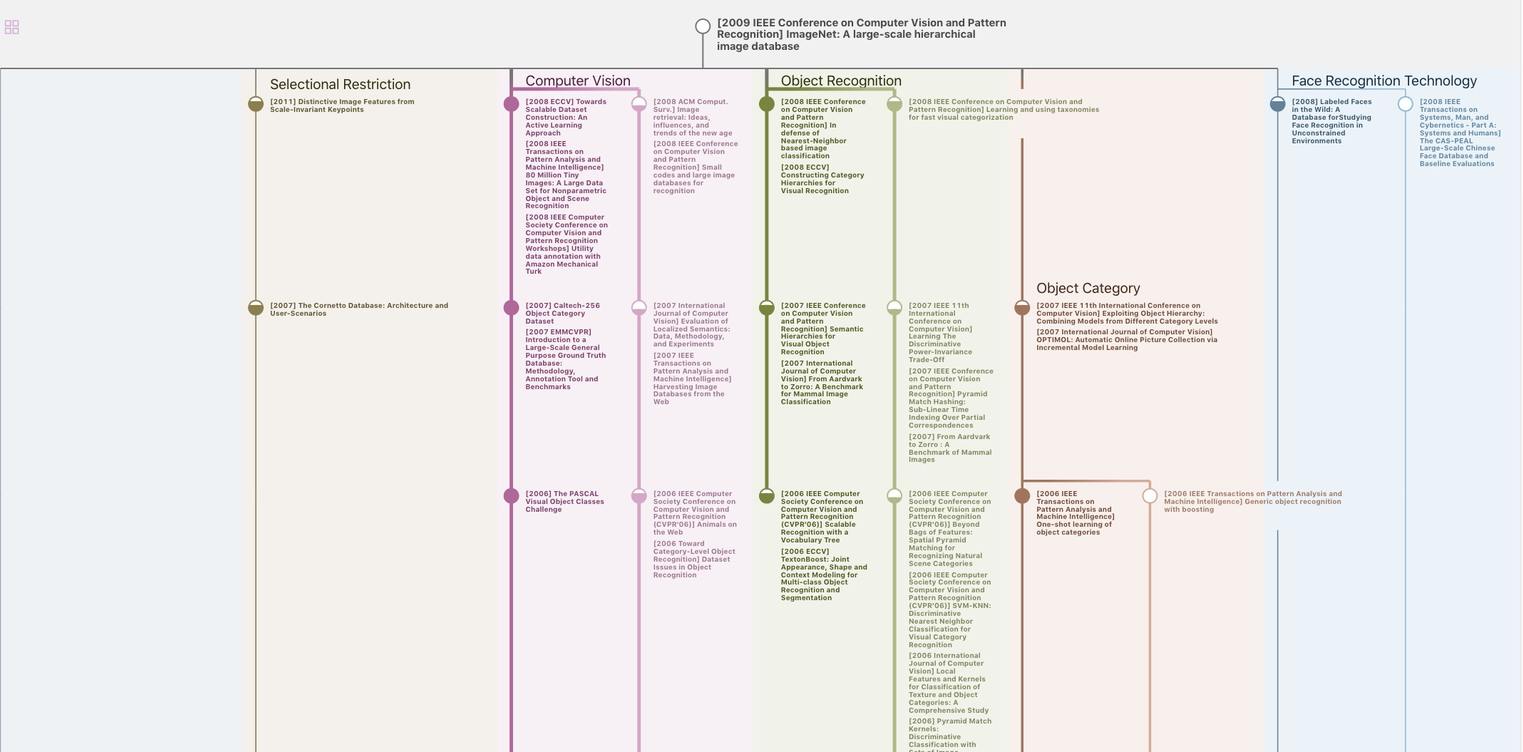
生成溯源树,研究论文发展脉络
Chat Paper
正在生成论文摘要