Hyperspectral Image Denoising via $L_{0}$ Regularized Low-Rank Tucker Decomposition
IEEE Journal of Selected Topics in Applied Earth Observations and Remote Sensing(2024)
Abstract
This article studies the mixed noise removal problem for hyperspectral images (HSIs), which often suffer from Gaussian noise and sparse noise. Conventional denoising models mainly employ the
$L_{1}$
-norm-based regularizers to remove sparse noise and ensure piecewise smoothness. However, the denoising performance is poor for highly structured images with severe noise since the
$L_{1}$
-norm overpenalizes large entries. To tackle this limitation, we propose a denoising model that combines tensor decomposition with two kinds of
$L_{0}$
-norm-based regularizers. First, we use low-rank Tucker decomposition with the Stiefel manifold to characterize the global correlation of HSIs. Then, we utilize the
$L_{0}$
-norm to leverage the intrinsic sparsity information of the corruption domain, thereby enhancing the effectiveness of sparse noise removal. Simultaneously, we introduce a weighted
$L_{0}$
-norm regularizer on the gradient of each pixel to promote the local spectral-spatial smoothness. To solve the proposed model, we design a hard-thresholding-based alternating direction method of multipliers algorithm. Instead of spending time to find a proper rank in advance, we adopt a rank-increasing scheme to dynamically adjust the tensor rank during the optimization procedure. In this way, our algorithm avoids the rank selection burden and improves computational efficiency. Finally, we test the proposed method on both synthetic and real datasets. Numerical results demonstrate its superiority, especially, the improvements of our method over the best-compared results up to 2.07 dB for mixed noise removal.
MoreTranslated text
Key words
Low-rank,piecewise smooth,rank-increasing,sparse
AI Read Science
Must-Reading Tree
Example
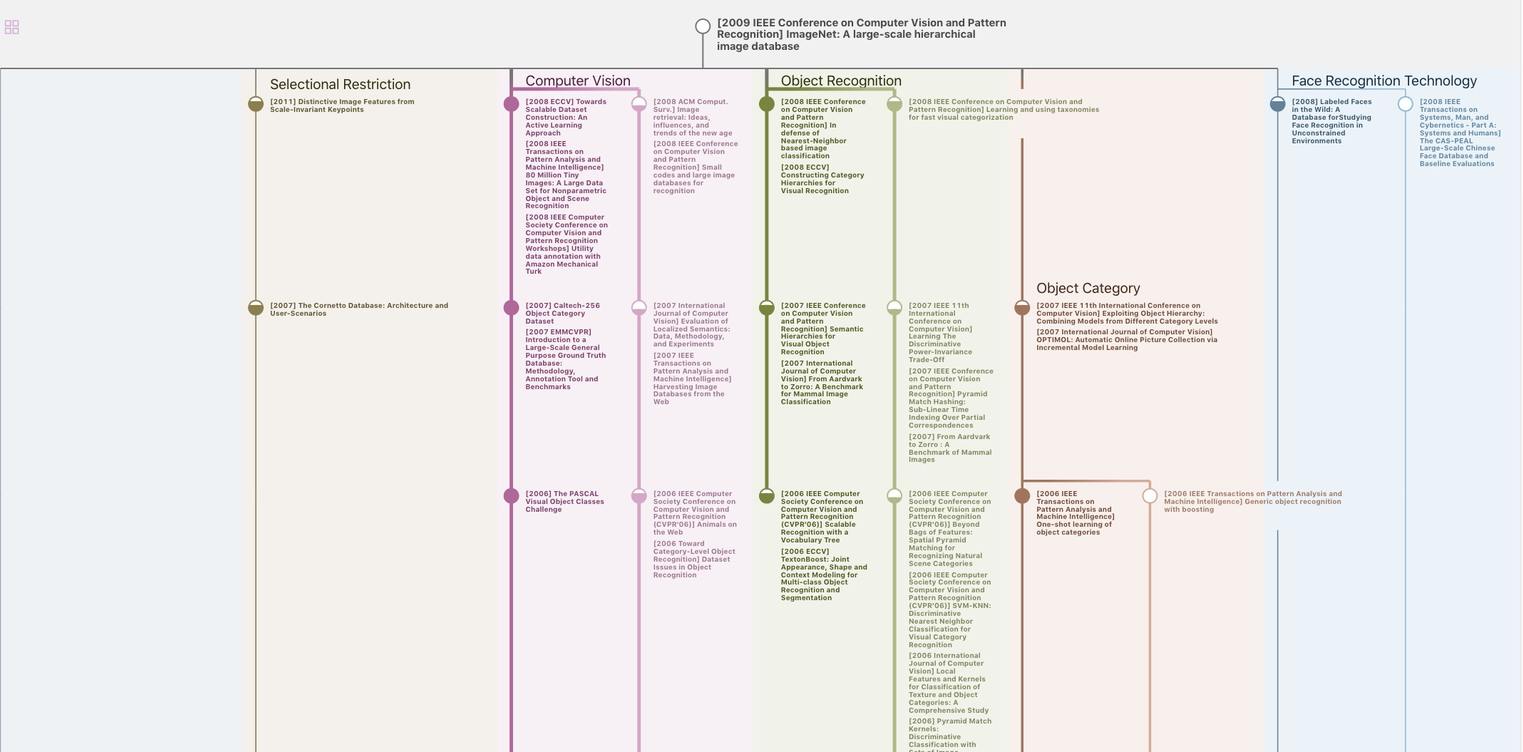
Generate MRT to find the research sequence of this paper
Chat Paper
Summary is being generated by the instructions you defined