DeepDiabetic: An Identification System of Diabetic Eye Diseases Using Deep Neural Networks
IEEE ACCESS(2024)
Abstract
Deep Learning (DL) plays a successful and influential role in medical imaging diagnosis, image detection, and image classification. Diabetes is a significant public health concern, and diabetic eye disease will be the leading cause of vision loss across the globe. This research proposed a multi-classification deep learning model for diagnosing and identifying four different diabetic eye diseases: Diabetic Retinopathy (DR), Diabetic Macular Edema (DME), glaucoma, and cataract which we called the DeepDiabetic framework. The proposed models were assessed using 1228 images from six different available datasets (DIARETDB0, DIARETDB1, Messidor, HEI-MED, Ocular, and Retina). In addition to the original dataset, we measured the performance of the deep learning models according to two different geometric augmentation methods called online augmented and offline augmented. The present work considers five architectures' performances: EfficientNetB0, VGG16, ResNet152V2, ResNet152V2 + Gated Recurrent Unit (GRU), and ResNet152V2 + Bidirectional GRU (Bi-GRU). A comprehensive analysis and evaluation of these deep learning architectures is provided using public fundus datasets with four classes (i.e., DR, DME, Glaucoma, and Cataract). To the best of our knowledge, no other deep learning models for choosing between these models for these specific diseases are found in the literature. The results of the experiments showed that the EfficientNetB0 model outperforms the other four proposed models. The EfficientNetB0 model achieved 0.9876 in accuracy, 0.9876 recall, 0.9876 precision, and 0.9977 AUC based on fundus images. Our EfficientNetB0 model achieves 98.76% accuracy, while the previous studies only achieved 88.33%, 89.54%, 97.23%, and 80.33% accuracy, respectively. When compared to the previous studies as Fast-RCNN, RCNN-LSTM, and InceptionResNet, our EfficientNetB0 model achieves much higher accuracy, recall, precision, and AUC. According to the outcomes, our proposed models, especially the EfficientNetB0 model, are significantly more accurate than the state-of-the-art models.
MoreTranslated text
Key words
Diabetes,Eye diseases,Diseases,Deep learning,Feature extraction,Artificial neural networks,Cataracts,Retina,diabetic eye disease,multi-class classification,offline augmented,online augmented,retinal fundus images
AI Read Science
Must-Reading Tree
Example
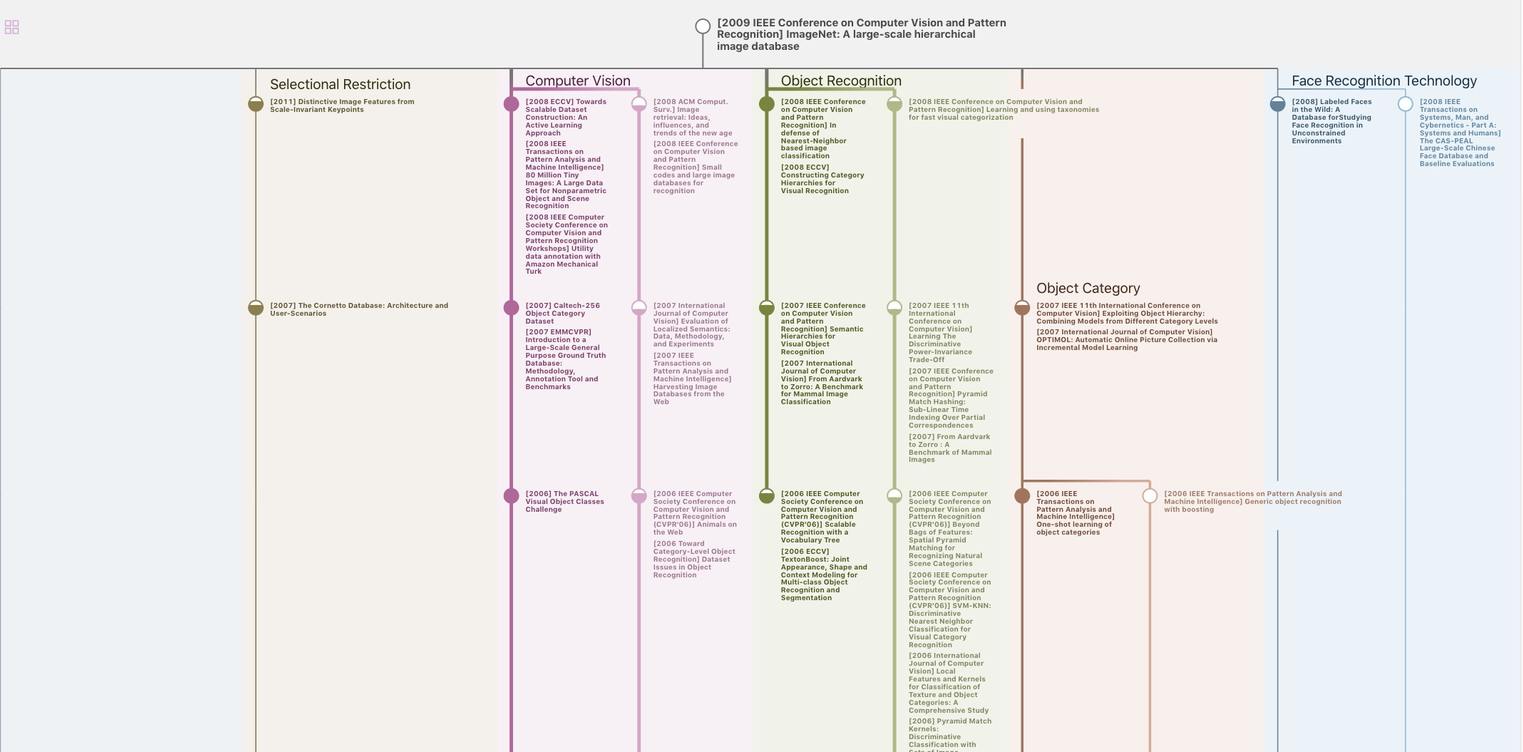
Generate MRT to find the research sequence of this paper
Chat Paper
Summary is being generated by the instructions you defined