CCG-YOLOv7: A Wood Defect Detection Model for Small Targets Using Improved YOLOv7
IEEE ACCESS(2024)
摘要
The Chinese furniture market has a high demand for wood floors. Manual defect detection in wood floors is inefficient and lacks stability in accuracy. It is necessary to conduct research on automatic defect detection in wood floors. To improve the accuracy of detecting small defects in wood floors, this paper proposed a new network based on YOLOv7. The new network is called the cascade center of gravity YOLOv7 (CCG-YOLOv7). This paper designed cascade efficient layer aggregation networks (C-ELAN), streamlined the CBS, replaced the ELAN with the C-ELAN, introduced the rapid supervised attention module to connect the backbone and head layers, and simplified the head layer of the YOLOv7 network. These methods improved the detection accuracy and speed for detecting small defects on wood floor surfaces. The improved network can effectively detect small defects on the wooden board surfaces, including knots, scratches, and mildew. Compared to the original YOLOv7, CCG-YOLOv7 improves precision, recall, and mean average precision by 2.1%, 1.6%, and 1.2%, respectively.
更多查看译文
关键词
Floors,Feature extraction,Production,Gravity,Testing,Shape,Convolutional neural networks,Deep learning,Surface cracks,small target,wood floor defect detection,YOLOv7
AI 理解论文
溯源树
样例
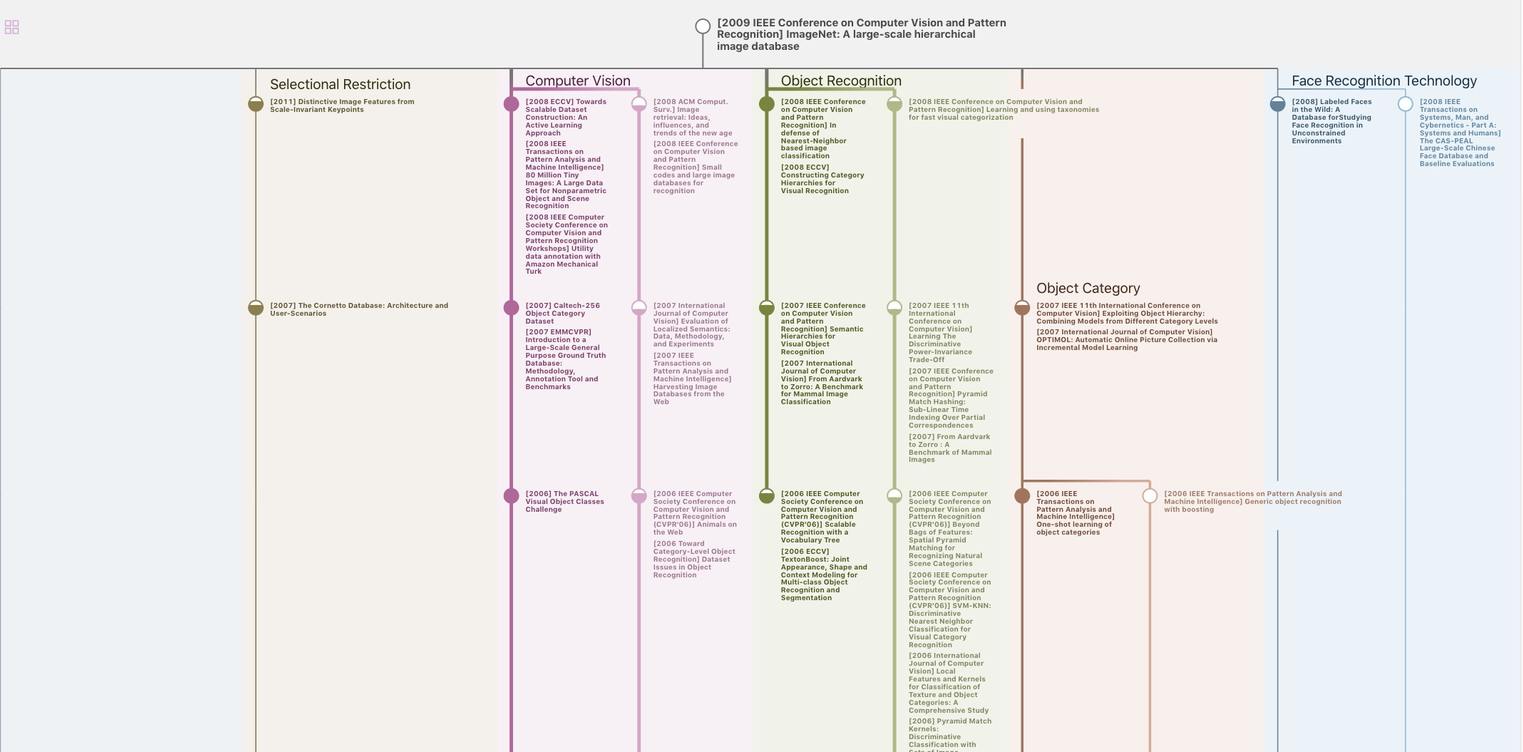
生成溯源树,研究论文发展脉络
Chat Paper
正在生成论文摘要