Defending Against Backdoor Attacks by Quarantine Training
IEEE ACCESS(2024)
摘要
Deep neural networks (DNNs) are powerful yet vulnerable to backdoor attacks simply by adding backdoor samples to the training set without controlling the training process. To filter out the backdoor samples in the training set, this paper proposes a novel and effective backdoor defense method called Quarantine Training (QT). Specifically, QT creates a quarantine class for each class in the training set and relabels all sample labels to associate with their corresponding quarantine classes during training. In this process, the backdoor samples are gradually categorized into the quarantine classes, thus effectively filtering out the backdoor samples. Experiments on multiple benchmark datasets with a variety of backdoor attacks demonstrate that QT has state-of-the-art backdoor defense performance without reducing the prediction accuracy of benign samples - and even improving it. Our codes are available at https://github.com/Chengx-Yu/Quarantine-Training.
更多查看译文
关键词
Deep neural networks,trustworthy A,backdoor attack,backdoor defense
AI 理解论文
溯源树
样例
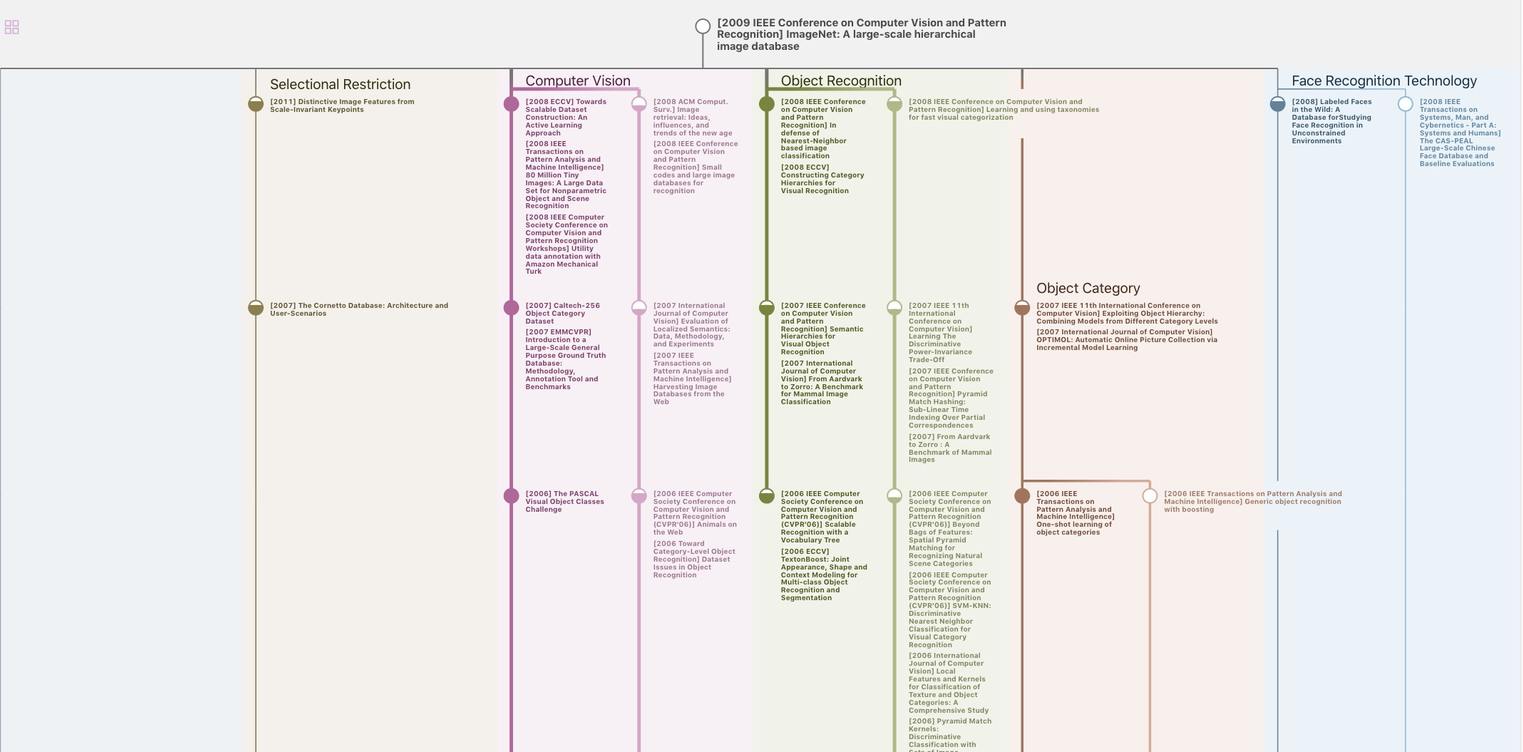
生成溯源树,研究论文发展脉络
Chat Paper
正在生成论文摘要