GI-PIP: Do We Require Impractical Auxiliary Dataset for Gradient Inversion Attacks?
CoRR(2024)
摘要
Deep gradient inversion attacks expose a serious threat to Federated Learning
(FL) by accurately recovering private data from shared gradients. However, the
state-of-the-art heavily relies on impractical assumptions to access excessive
auxiliary data, which violates the basic data partitioning principle of FL. In
this paper, a novel method, Gradient Inversion Attack using Practical Image
Prior (GI-PIP), is proposed under a revised threat model. GI-PIP exploits
anomaly detection models to capture the underlying distribution from fewer
data, while GAN-based methods consume significant more data to synthesize
images. The extracted distribution is then leveraged to regulate the attack
process as Anomaly Score loss. Experimental results show that GI-PIP achieves a
16.12 dB PSNR recovery using only 3.8
methods necessitate over 70
distribution generalization compared to GAN-based methods. Our approach
significantly alleviates the auxiliary data requirement on both amount and
distribution in gradient inversion attacks, hence posing more substantial
threat to real-world FL.
更多查看译文
关键词
Federated learning,Gradient inversion,Privacy leakage,Anomaly detection,Practical image prior
AI 理解论文
溯源树
样例
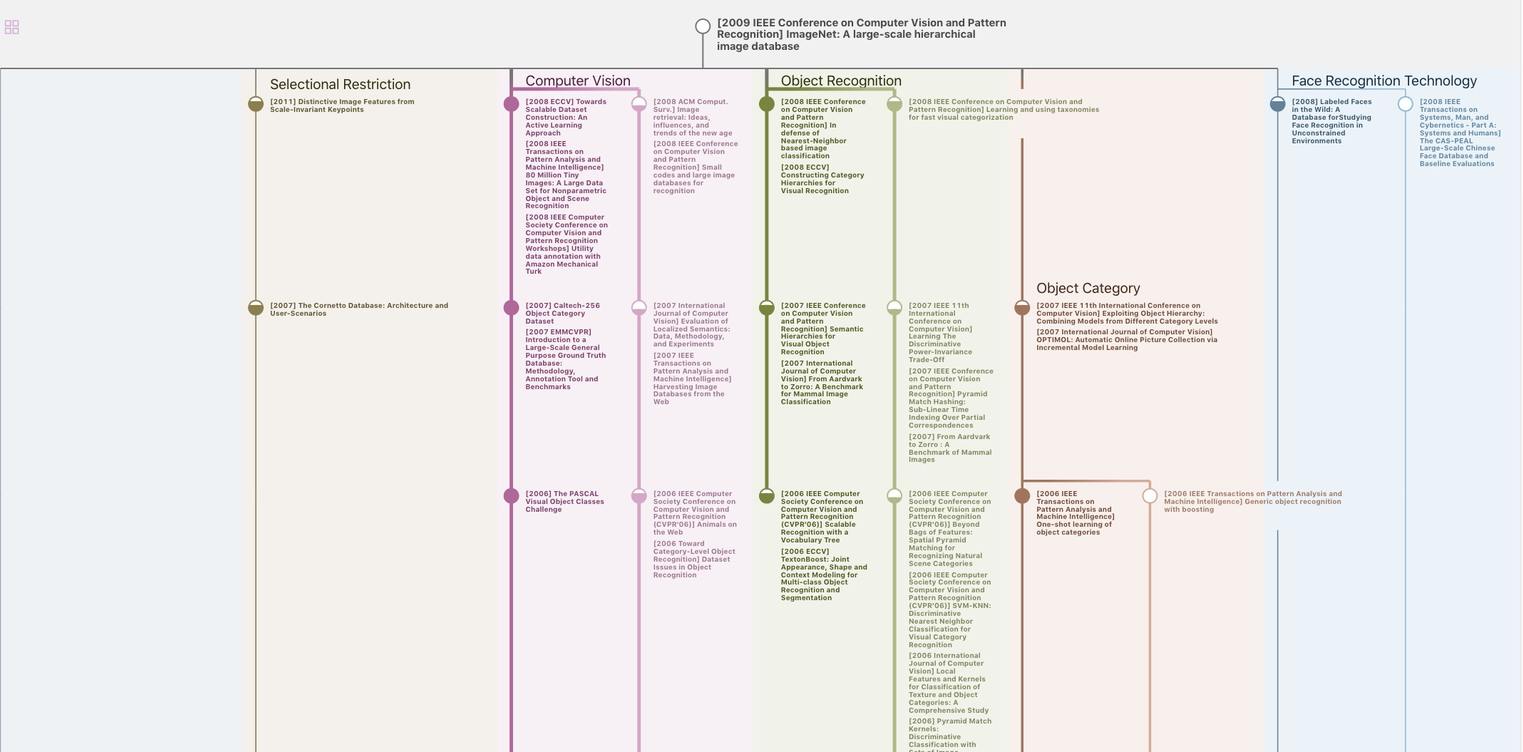
生成溯源树,研究论文发展脉络
Chat Paper
正在生成论文摘要