TetraLoss: Improving the Robustness of Face Recognition against Morphing Attacks
CoRR(2024)
摘要
Face recognition systems are widely deployed in high-security applications
such as for biometric verification at border controls. Despite their high
accuracy on pristine data, it is well-known that digital manipulations, such as
face morphing, pose a security threat to face recognition systems. Malicious
actors can exploit the facilities offered by the identity document issuance
process to obtain identity documents containing morphed images. Thus, subjects
who contributed to the creation of the morphed image can with high probability
use the identity document to bypass automated face recognition systems. In
recent years, no-reference (i.e., single image) and differential morphing
attack detectors have been proposed to tackle this risk. These systems are
typically evaluated in isolation from the face recognition system that they
have to operate jointly with and do not consider the face recognition process.
Contrary to most existing works, we present a novel method for adapting deep
learning-based face recognition systems to be more robust against face morphing
attacks. To this end, we introduce TetraLoss, a novel loss function that learns
to separate morphed face images from its contributing subjects in the embedding
space while still preserving high biometric verification performance. In a
comprehensive evaluation, we show that the proposed method can significantly
enhance the original system while also significantly outperforming other tested
baseline methods.
更多查看译文
关键词
Face Recognition,Morphing Attack,Loss Function,Cross-border,Single Image,Original System,Latent Space,Face Images,Learning-based System,Digital Manipulation,Differential Attacks,Deep Learning-based System,Benchmark,Detection System,System Performance,Positive Samples,Percentage Points,Negative Samples,Real Scenarios,Reference Image,Triplet Loss,Vulnerability Of Systems,Imaging Probes,ResNet-50 Backbone,European Union's Horizon,Intra-class Variance,Multilayer Perception,Union's Horizon,Quadruplet
AI 理解论文
溯源树
样例
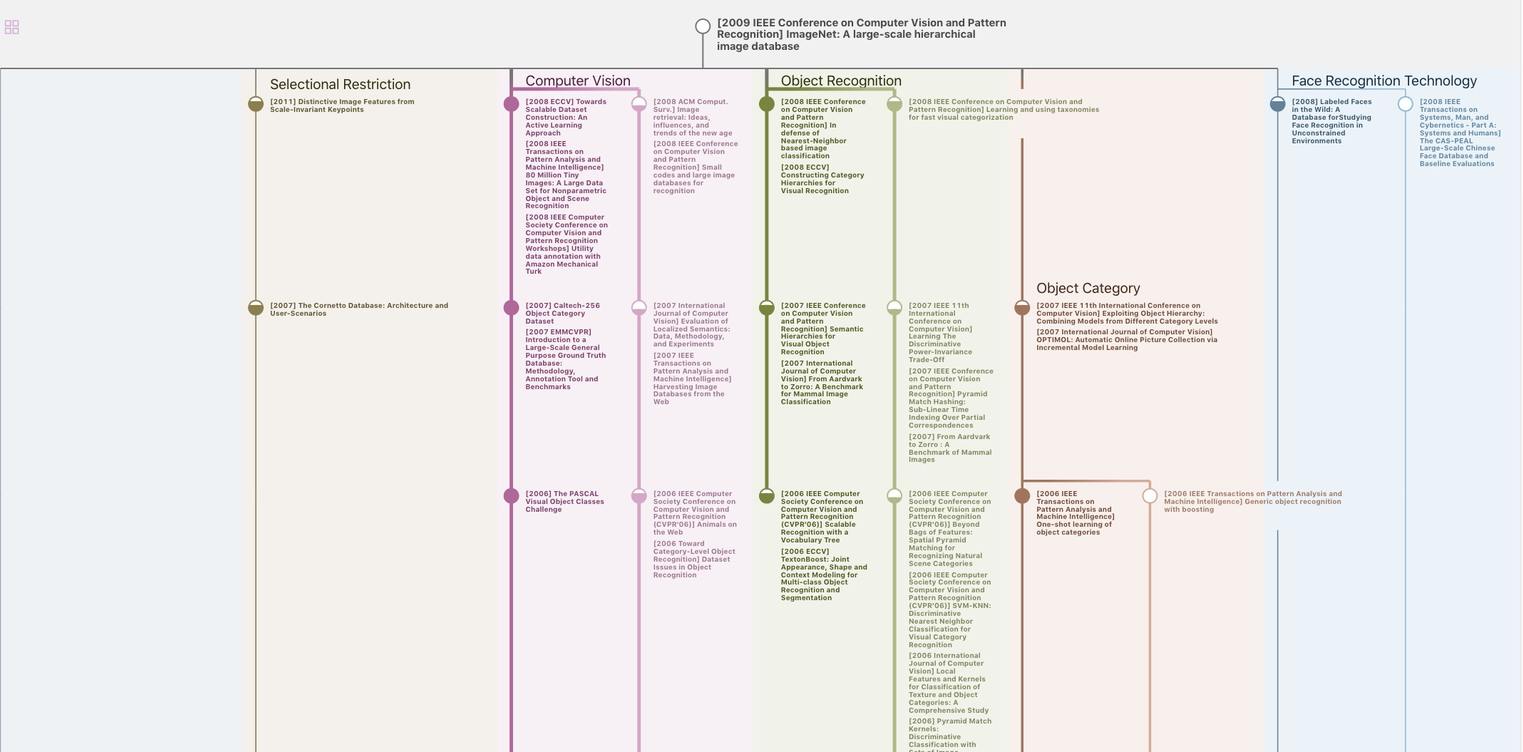
生成溯源树,研究论文发展脉络
Chat Paper
正在生成论文摘要