On-Device Recommender Systems: A Comprehensive Survey
CoRR(2024)
摘要
Recommender systems have been widely deployed in various real-world
applications to help users identify content of interest from massive amounts of
information. Traditional recommender systems work by collecting user-item
interaction data in a cloud-based data center and training a centralized model
to perform the recommendation service. However, such cloud-based recommender
systems (CloudRSs) inevitably suffer from excessive resource consumption,
response latency, as well as privacy and security risks concerning both data
and models. Recently, driven by the advances in storage, communication, and
computation capabilities of edge devices, there has been a shift of focus from
CloudRSs to on-device recommender systems (DeviceRSs), which leverage the
capabilities of edge devices to minimize centralized data storage requirements,
reduce the response latency caused by communication overheads, and enhance user
privacy and security by localizing data processing and model training. Despite
the rapid rise of DeviceRSs, there is a clear absence of timely literature
reviews that systematically introduce, categorize and contrast these methods.
To bridge this gap, we aim to provide a comprehensive survey of DeviceRSs,
covering three main aspects: (1) the deployment and inference of DeviceRSs (2)
the training and update of DeviceRSs (3) the security and privacy of DeviceRSs.
Furthermore, we provide a fine-grained and systematic taxonomy of the methods
involved in each aspect, followed by a discussion regarding challenges and
future research directions. This is the first comprehensive survey on DeviceRSs
that covers a spectrum of tasks to fit various needs. We believe this survey
will help readers effectively grasp the current research status in this field,
equip them with relevant technical foundations, and stimulate new research
ideas for developing DeviceRSs.
更多查看译文
AI 理解论文
溯源树
样例
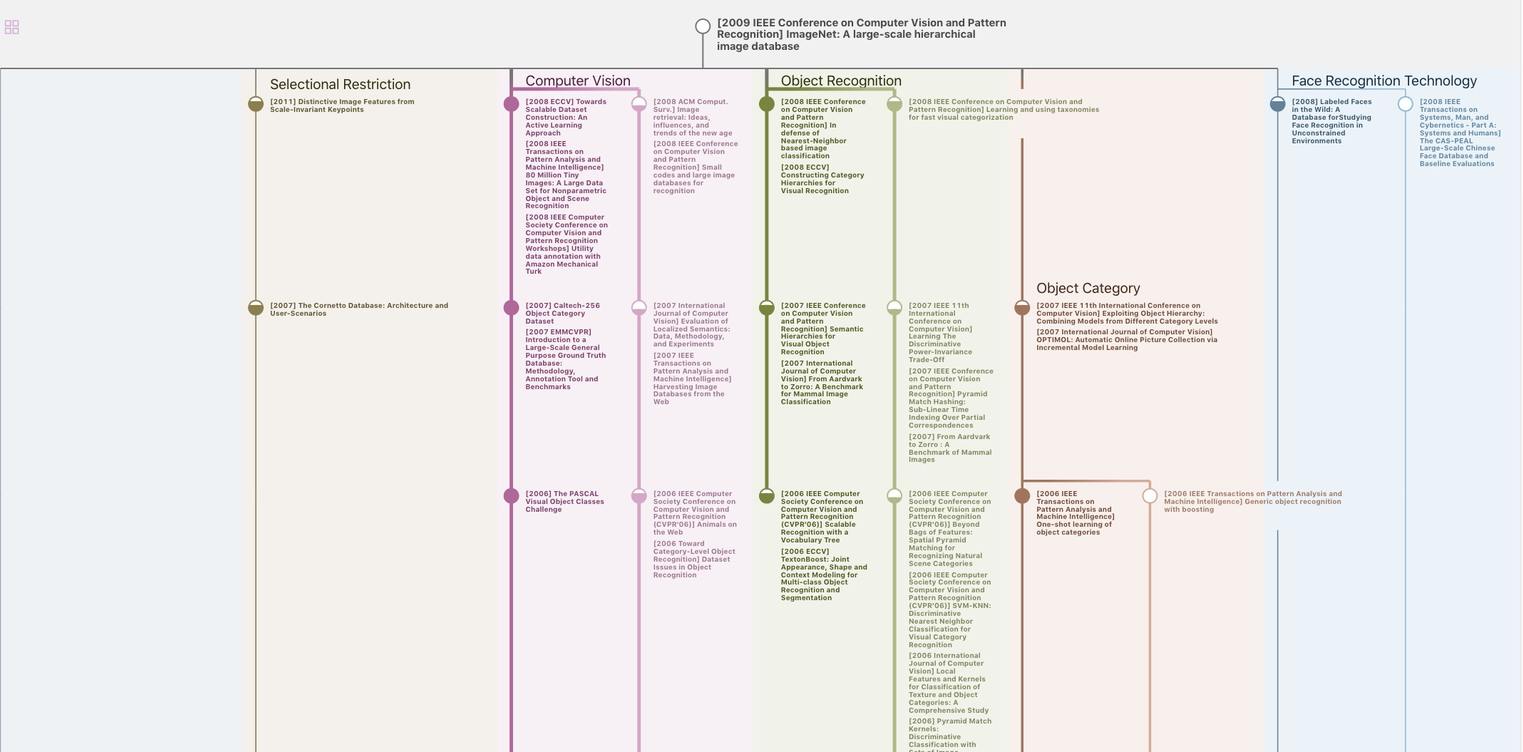
生成溯源树,研究论文发展脉络
Chat Paper
正在生成论文摘要