Enhancing selectivity using Wasserstein distance based reweighing
CoRR(2024)
摘要
Given two labeled data-sets 𝒮 and 𝒯, we design a
simple and efficient greedy algorithm to reweigh the loss function such that
the limiting distribution of the neural network weights that result from
training on 𝒮 approaches the limiting distribution that would have
resulted by training on 𝒯.
On the theoretical side, we prove that when the metric entropy of the input
data-sets is bounded, our greedy algorithm outputs a close to optimal
reweighing, i.e., the two invariant distributions of network weights will be
provably close in total variation distance. Moreover, the algorithm is simple
and scalable, and we prove bounds on the efficiency of the algorithm as well.
Our algorithm can deliberately introduce distribution shift to perform (soft)
multi-criteria optimization. As a motivating application, we train a neural net
to recognize small molecule binders to MNK2 (a MAP Kinase, responsible for cell
signaling) which are non-binders to MNK1 (a highly similar protein). We tune
the algorithm's parameter so that overall change in holdout loss is negligible,
but the selectivity, i.e., the fraction of top 100 MNK2 binders that are MNK1
non-binders, increases from 54% to 95%, as a result of our reweighing. Of the
43 distinct small molecules predicted to be most selective from the enamine
catalog, 2 small molecules were experimentally verified to be selective, i.e.,
they reduced the enzyme activity of MNK2 below 50% but not MNK1, at 10μM
– a 5% success rate.
更多查看译文
AI 理解论文
溯源树
样例
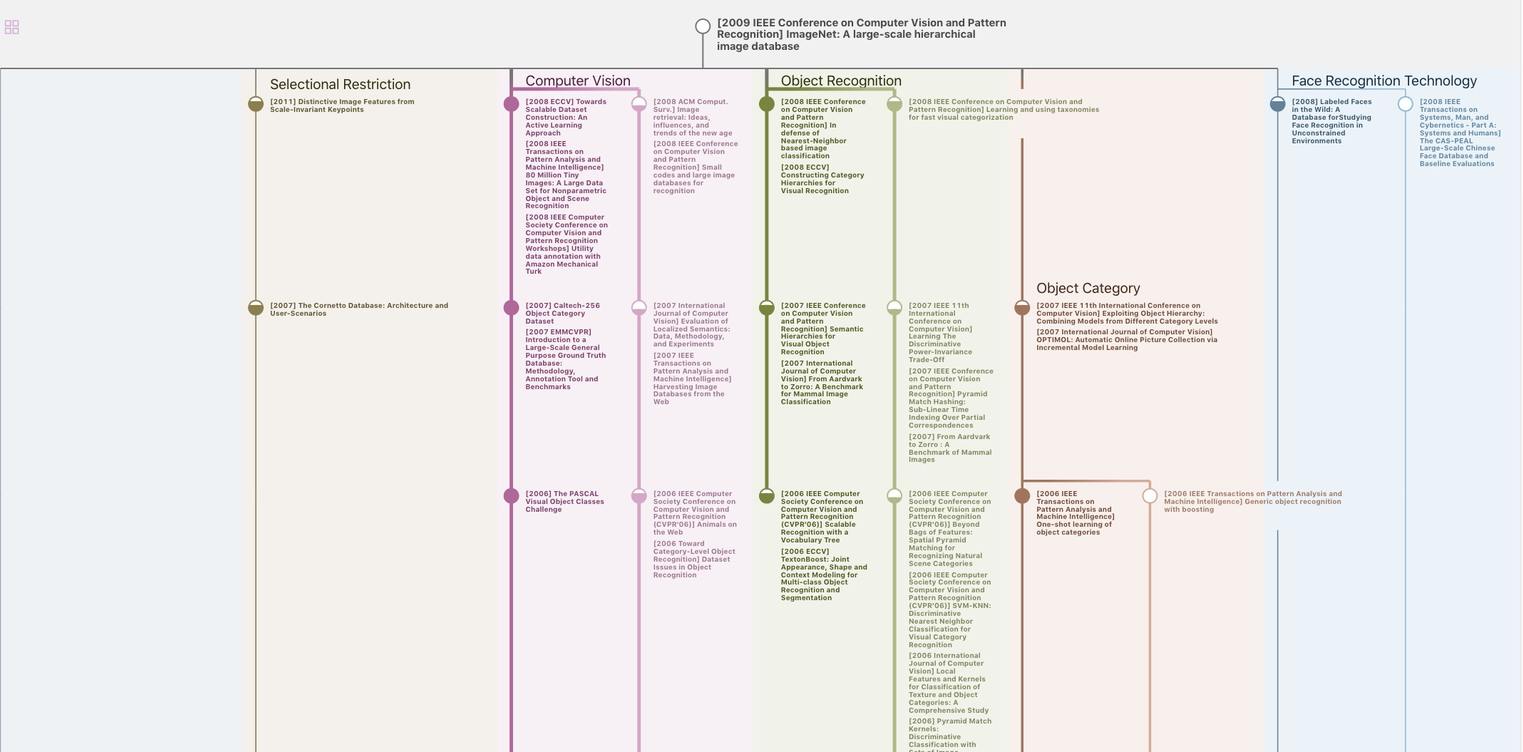
生成溯源树,研究论文发展脉络
Chat Paper
正在生成论文摘要