Latent domain knowledge distillation for nighttime semantic segmentation
ENGINEERING APPLICATIONS OF ARTIFICIAL INTELLIGENCE(2024)
摘要
Despite significant progress made in image semantic segmentation, most research has primarily focused on daytime scenes. Semantic segmentation of nighttime images is equally critical for autonomous driving; however, this task poses more challenges due to inadequate lighting and difficulties associated with obtaining accurate manual annotations. In this paper, we propose a novel method called Latent Domain Knowledge Distillation (LDKD) for accurate nighttime semantic segmentation. Within a teacher -student framework, our LDKD combines domain alignment and knowledge distillation in a mutually reinforcing manner. First, we introduce a bidirectional photometric alignment module capable of generating daytime -like and nighttime -like latent images in real-time. This module effectively bridges the appearance gap between the source domain (daytime) and the target domain (nighttime). Second, we enhance collaboration between the teacher and student networks. The student network unifies image -level and feature -level domain alignment, while the teacher network generates reliable pseudo -labels by distilling knowledge from the latent domain. Furthermore, to account for potential noise in pseudo -labels, we propose a noise -tolerant learning method aimed at mitigating the risks associated with overreliance on pseudo -labels during model training. Extensive experiments on Dark Zurich and ACDC datasets show that our LDKD achieves state-of-the-art performance, demonstrating the effectiveness of our method for nighttime semantic segmentation.
更多查看译文
关键词
Semantic segmentation,Domain adaptation,Knowledge distillation,Noise-tolerant learning loss
AI 理解论文
溯源树
样例
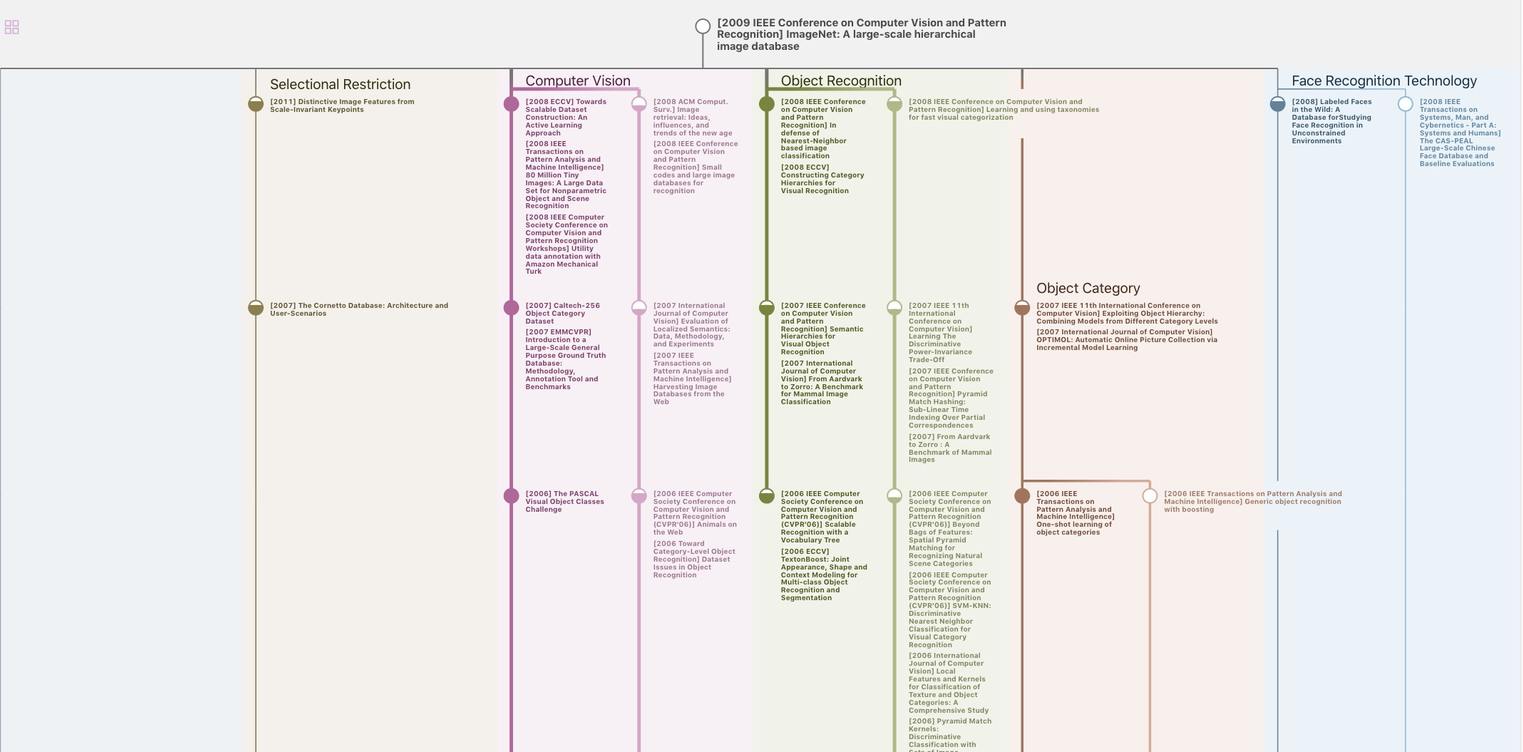
生成溯源树,研究论文发展脉络
Chat Paper
正在生成论文摘要