Efficient federated learning privacy preservation method with heterogeneous differential privacy
COMPUTERS & SECURITY(2024)
摘要
Federated learning (FL) is a distributed machine learning method that effectively protects personal data. Many studies on federated learning assumed that all clients have consistent privacy parameters. However, in practice, different clients have different privacy requirements, and heterogeneous differential privacy can personalize privacy protection according to each client's privacy budget and requirements. In this study, we propose an improved efficient FL privacy preservation method with heterogeneous differential privacy, which can compute the corresponding privacy budget weights for each client according to noise size using the secure differential privacy stochastic gradient descent protocol, histogram of oriented gradients feature extraction and weighted averaging of the heterogeneous privacy budgets. Through this method, the noisier clients are given smaller privacy budgets weights to mitigate their negative impact on the aggregation model. Experiments comparing the baseline method were performed on the MNIST, fMNIST and cifar10 datasets. More precisely, the experimental results showed that our method improves the model accuracy by 6.68% and 7.18% of 20 to 50 clients and 16.08% and 17.37% of 60 to 100 clients, respectively. Moreover, the communication overhead time was reduced by 23.85%, which validates the effectiveness and usability of the proposed method.
更多查看译文
关键词
Federated learning,Differential privacy,Privacy computing,Heterogeneous differential privacy,Privacy-utility tradeoff
AI 理解论文
溯源树
样例
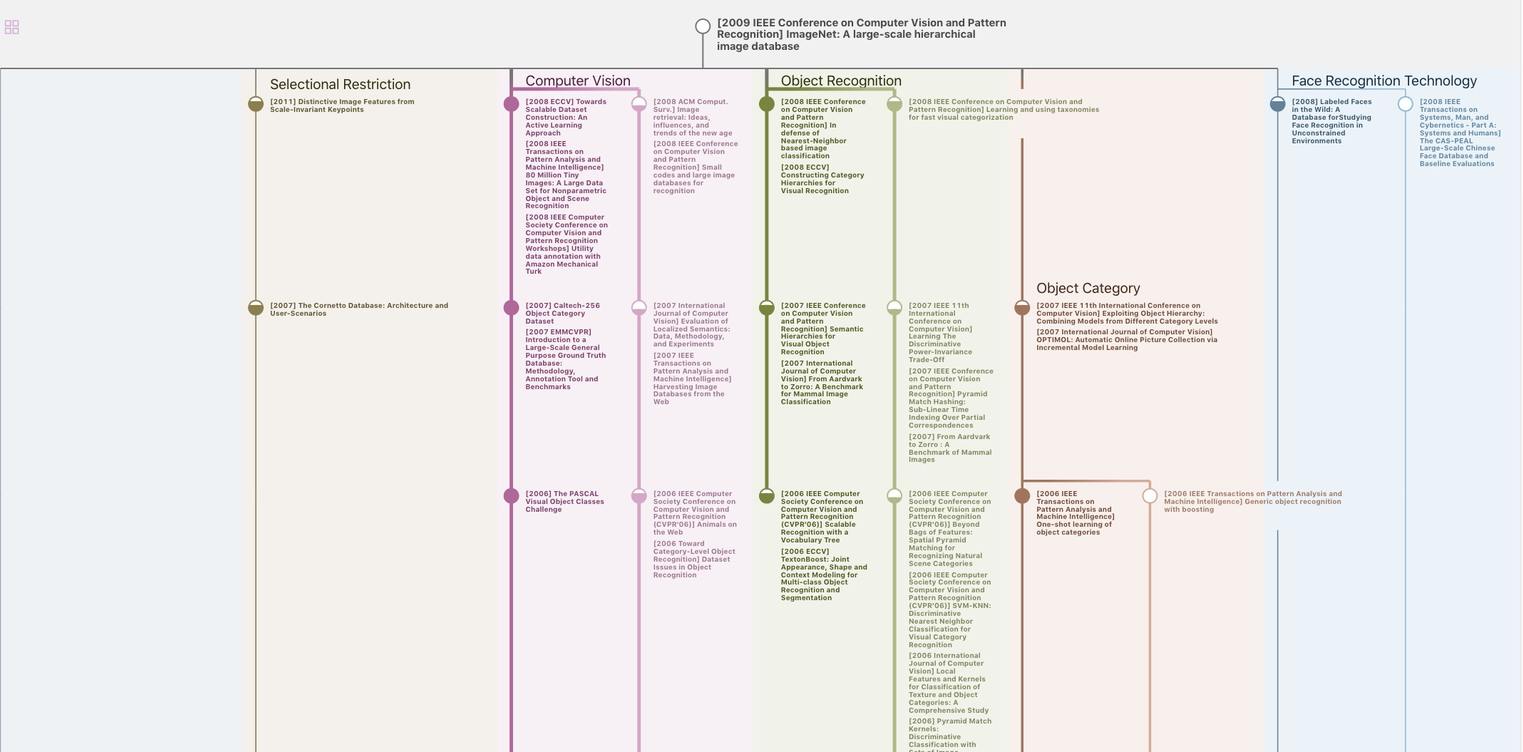
生成溯源树,研究论文发展脉络
Chat Paper
正在生成论文摘要