U-Net Convolutional Neural Network for Real-Time Prediction of the Number of Cultured Corneal Endothelial Cells for Cellular Therapy
BIOENGINEERING-BASEL(2024)
摘要
Corneal endothelial decompensation is treated by the corneal transplantation of donor corneas, but donor shortages and other problems associated with corneal transplantation have prompted investigations into tissue engineering therapies. For clinical use, cells used in tissue engineering must undergo strict quality control to ensure their safety and efficacy. In addition, efficient cell manufacturing processes are needed to make cell therapy a sustainable standard procedure with an acceptable economic burden. In this study, we obtained 3098 phase contrast images of cultured human corneal endothelial cells (HCECs). We labeled the images using semi-supervised learning and then trained a model that predicted the cell centers with a precision of 95.1%, a recall of 92.3%, and an F-value of 93.4%. The cell density calculated by the model showed a very strong correlation with the ground truth (Pearson's correlation coefficient = 0.97, p value = 8.10 x 10-52). The total cell numbers calculated by our model based on phase contrast images were close to the numbers calculated using a hemocytometer through passages 1 to 4. Our findings confirm the feasibility of using artificial intelligence-assisted quality control assessments in the field of regenerative medicine.
更多查看译文
关键词
corneal endothelial cell,tissue engineering,cellular therapy,artificial intelligence,deep learning,U-Net
AI 理解论文
溯源树
样例
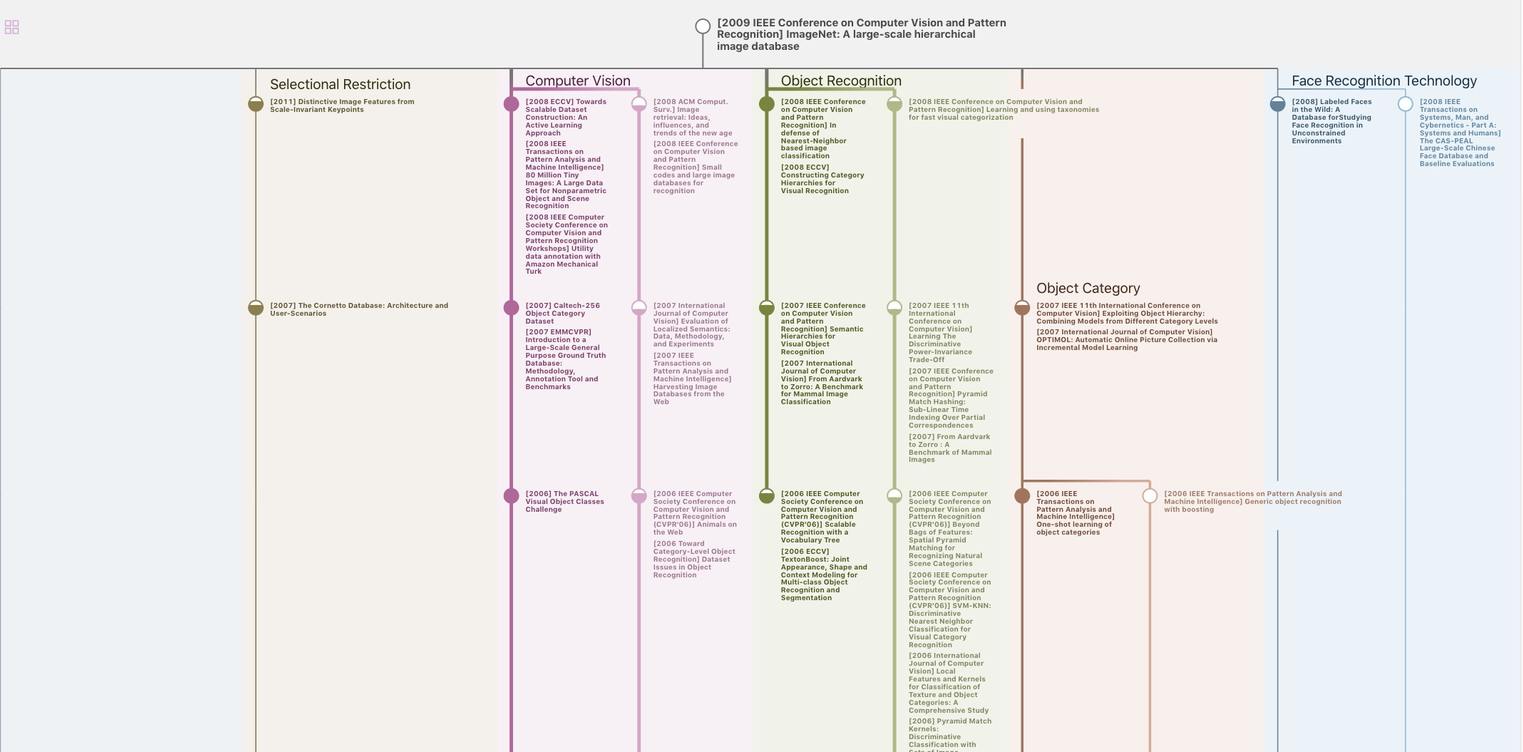
生成溯源树,研究论文发展脉络
Chat Paper
正在生成论文摘要