Cardiovascular Diseases Diagnosis Using an ECG Multi-Band Non-Linear Machine Learning Framework Analysis
BIOENGINEERING-BASEL(2024)
摘要
Background: cardiovascular diseases (CVDs), which encompass heart and blood vessel issues, stand as the leading cause of global mortality for many people. Methods: the present study intends to perform discrimination between seven well-known CVDs (bundle branch block, cardiomyopathy, myocarditis, myocardial hypertrophy, myocardial infarction, valvular heart disease, and dysrhythmia) and one healthy control group, respectively, by feeding a set of machine learning (ML) models with 10 non-linear features extracted every 1 s from electrocardiography (ECG) lead signals of a well-known ECG database (PTB diagnostic ECG database) using multi-band analysis performed by discrete wavelet transform (DWT). The ML models were trained and tested using a leave-one-out cross-validation approach, assessing the individual and combined capabilities of features, per each lead or combined, to distinguish between pairs of study groups and for conducting a comprehensive all vs. all analysis. Results: the Accuracy discrimination results ranged between 73% and 100%, the Recall between 68% and 100%, and the AUC between 0.42 and 1. Conclusions: the results suggest that our method is a good tool for distinguishing CVDs, offering significant advantages over other studies that used the same dataset, including a multi-class comparison group (all vs. all), a wider range of binary comparisons, and the use of classical non-linear analysis under ECG multi-band analysis performed by DWT.
更多查看译文
关键词
ECG signals,cardiovascular diseases,machine learning models,discrete wavelet transform,non-linear analysis,discrimination
AI 理解论文
溯源树
样例
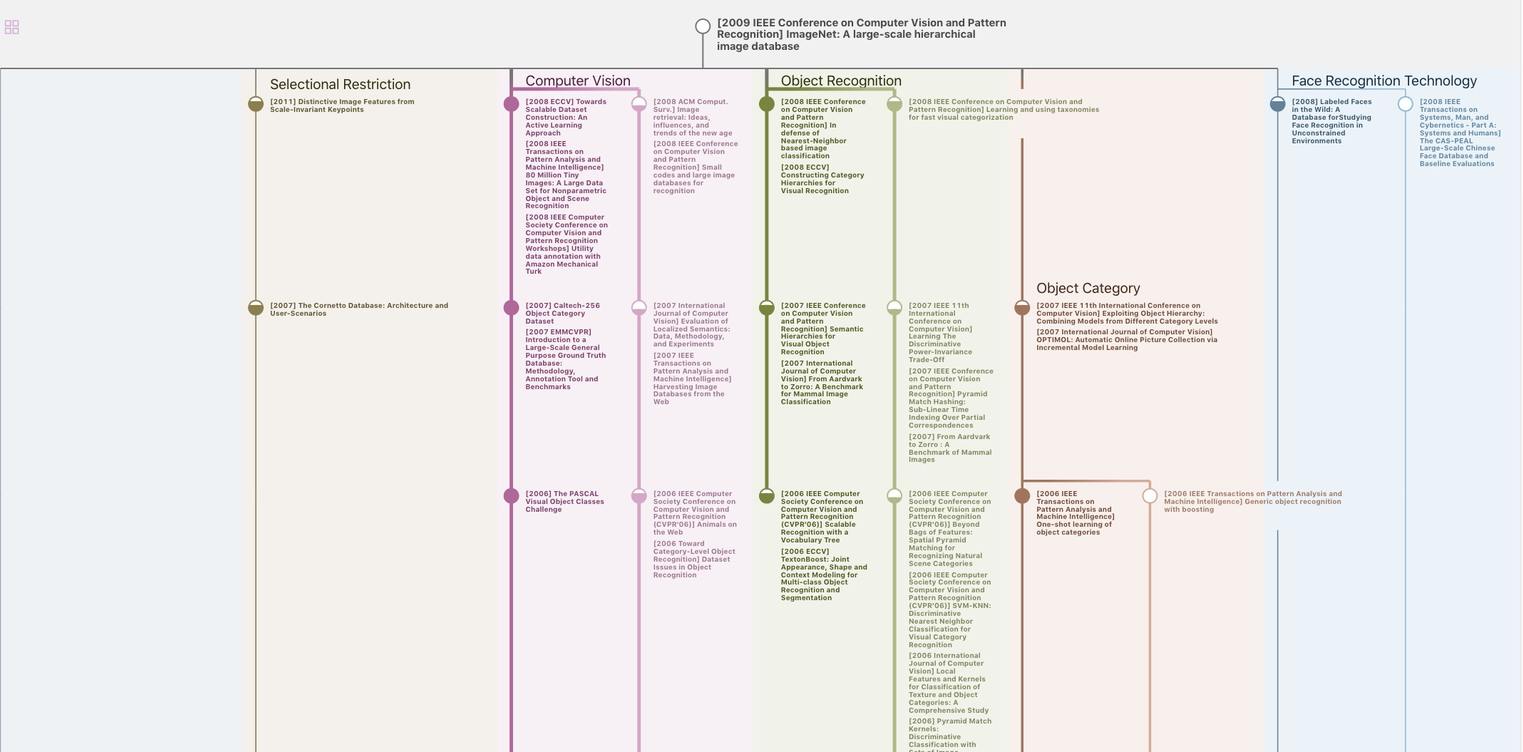
生成溯源树,研究论文发展脉络
Chat Paper
正在生成论文摘要