Emulation of Quantitative Systems Pharmacology models to accelerate virtual population inference in immuno-oncology
METHODS(2024)
摘要
Quantitative Systems Pharmacology (QSP) models are increasingly being applied for target discovery and dose selection in immuno-oncology (IO). Typical application involves virtual trial, a simulation of a virtual population of hundreds of model instances with model inputs reflecting individual variability. While the structure of the model and initial parameterisation are based on literature describing the underlying biology, calibration of the virtual population by existing clinical data is frequently required to create tumour and patient population specific model instances. Since comparison of a virtual trial with clinical output requires hundreds of large-scale, nonlinear model evaluations, the inference of a virtual population is computationally expensive, frequently becoming a bottleneck. Here, we present novel approach to virtual population inference in IO using emulation of the QSP model and an objective function based on Kolmogorov-Smirnov statistics to maximise congruence of simulated and observed clinical tumour size distributions. We sample the parameter space of a QSP IO model to collect a set of tumour growth time profiles. We evaluate performance of several machine learning approaches in interpolating these time profiles and create a surrogate model, which computes tumor growth profiles faster than the original model and allows examination of tens of millions of virtual patients. We use the surrogate model to infer a virtual population maximising congruence with the waterfall plot of a pembrolizumab clinical trial. We believe that our approach is applicable not only in QSP IO, but also in other applications where virtual populations need to be inferred for computationally expensive mechanistic models.
更多查看译文
关键词
Machine learning,Model emulation,Objective function optimization,Virtual population inference,Quantitative systems pharmacology,Immuno-oncology
AI 理解论文
溯源树
样例
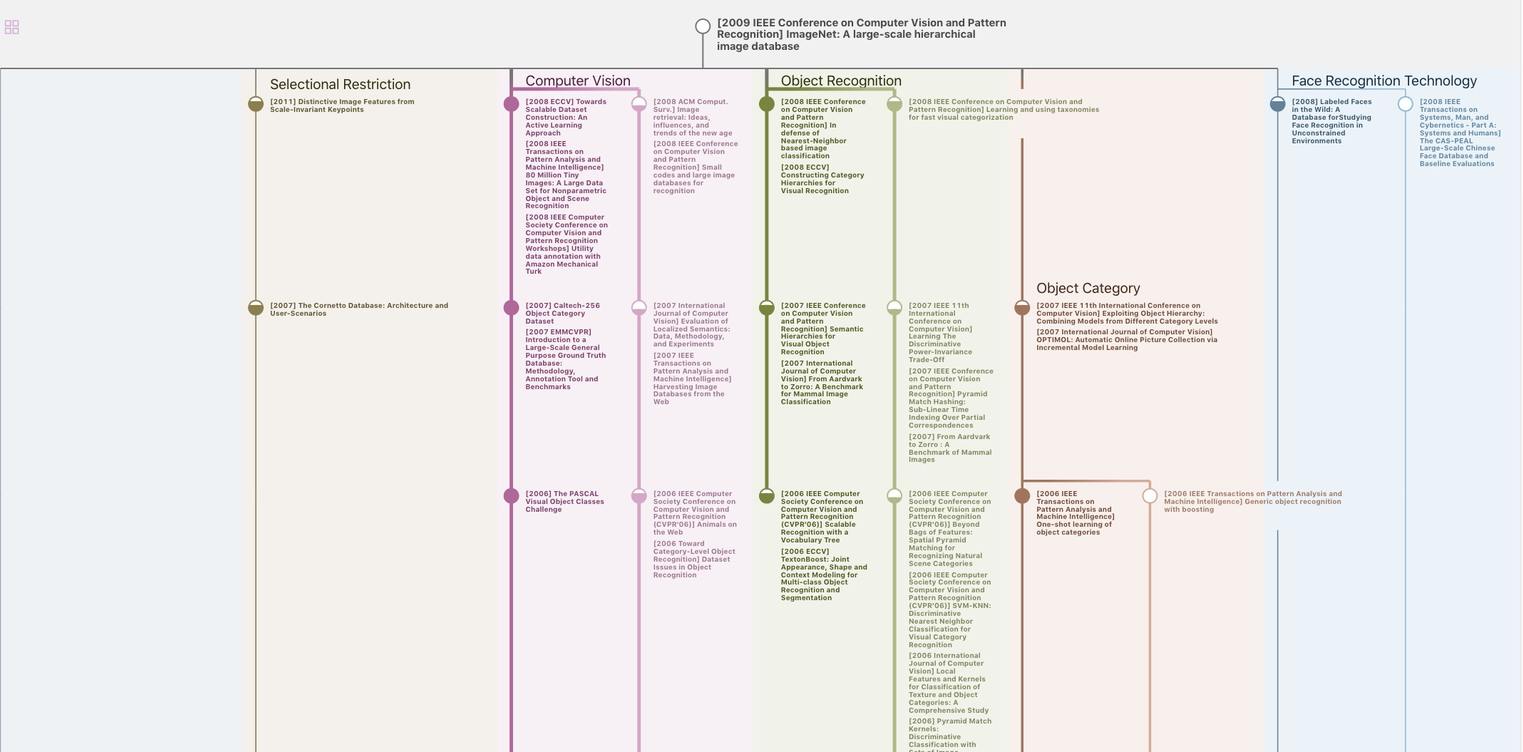
生成溯源树,研究论文发展脉络
Chat Paper
正在生成论文摘要