A Sparsity-Adapted Hardware Implementation of SNN for Cortical Spike Trains Decoding.
2023 IEEE Biomedical Circuits and Systems Conference (BioCAS)(2023)
Abstract
Neural decoding plays a crucial role in brain-computer interfaces (BCI), which is the basis for applications such as motion control and BCI-based rehabilitation. However, current neural decoding tasks are carried out with bulky, high-latency and power-consuming computers, which becomes a significant bottleneck for the development of next-generation wearable and implantable BCI systems. In this paper, we presented a Spiking neural network (SNN) processor based on adaptive Leaky Integrate-and-Fire (LIF) neurons for decoding monkey cortical spike trains collected in a 4-direction joystick reaching task. The proposed processor leveraging the temporal sparsity inherent in neural signals and SNN to reduce hardware resource requirements. Implemented on FPGA, it achieves a sparse matrix compression operation for single-bit data, which reduces dynamic power consumption and accelerates the inference between layers. Validated with our dataset, it achieves a 2.8% accuracy improvement and reduces spike counts by 70 % compared to feed-forward standard SNN, which means that the model is a suitable choice for energy-saving neural decoding at the edge.
MoreTranslated text
Key words
SNN,adaptive LIF neurons,sparse matrix operation,hardware implementation
AI Read Science
Must-Reading Tree
Example
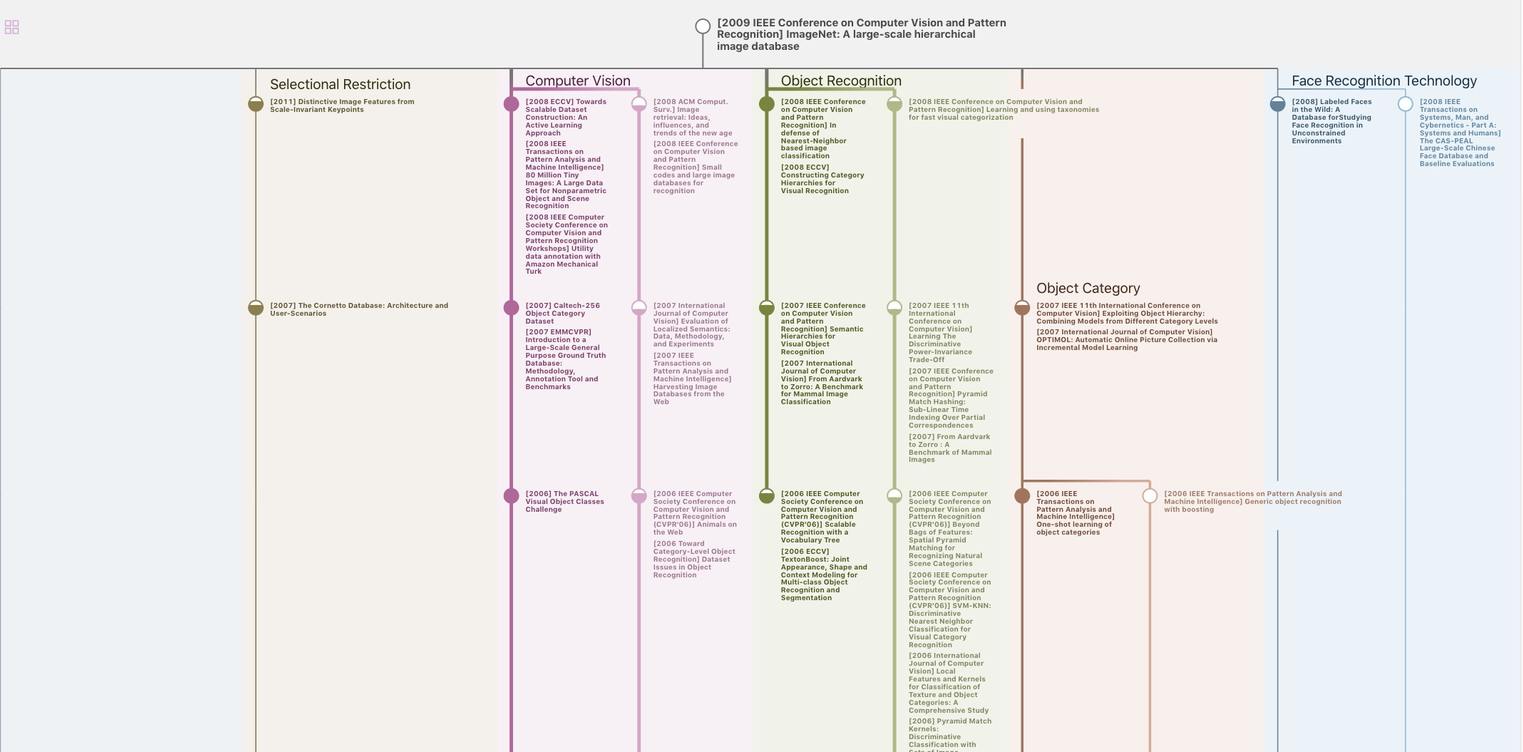
Generate MRT to find the research sequence of this paper
Chat Paper
Summary is being generated by the instructions you defined