Towards Scalable Kernel-Based Regularized System Identification
2023 62ND IEEE CONFERENCE ON DECISION AND CONTROL, CDC(2023)
摘要
This paper proposes a methodology for scalable kernel-based regularized system identification based on indirect methods. It leverages stochastic trace estimation methods and an iterative solver such as LSQR for the efficient evaluation of hyperparameter selection criteria. It also uses a derivative-free optimization approach to hyperparameter estimation, which avoids the need for computing gradients or Hessians of the objective function. Moreover, the method is matrix-free, which means it only relies on a matrix-vector oracle and exploits fast routines for various structured matrix-vector products. Our preliminary numerical experiments indicate that the methodology scales significantly better than direct methods, especially when dealing with large datasets and slowly decaying impulse responses.
更多查看译文
AI 理解论文
溯源树
样例
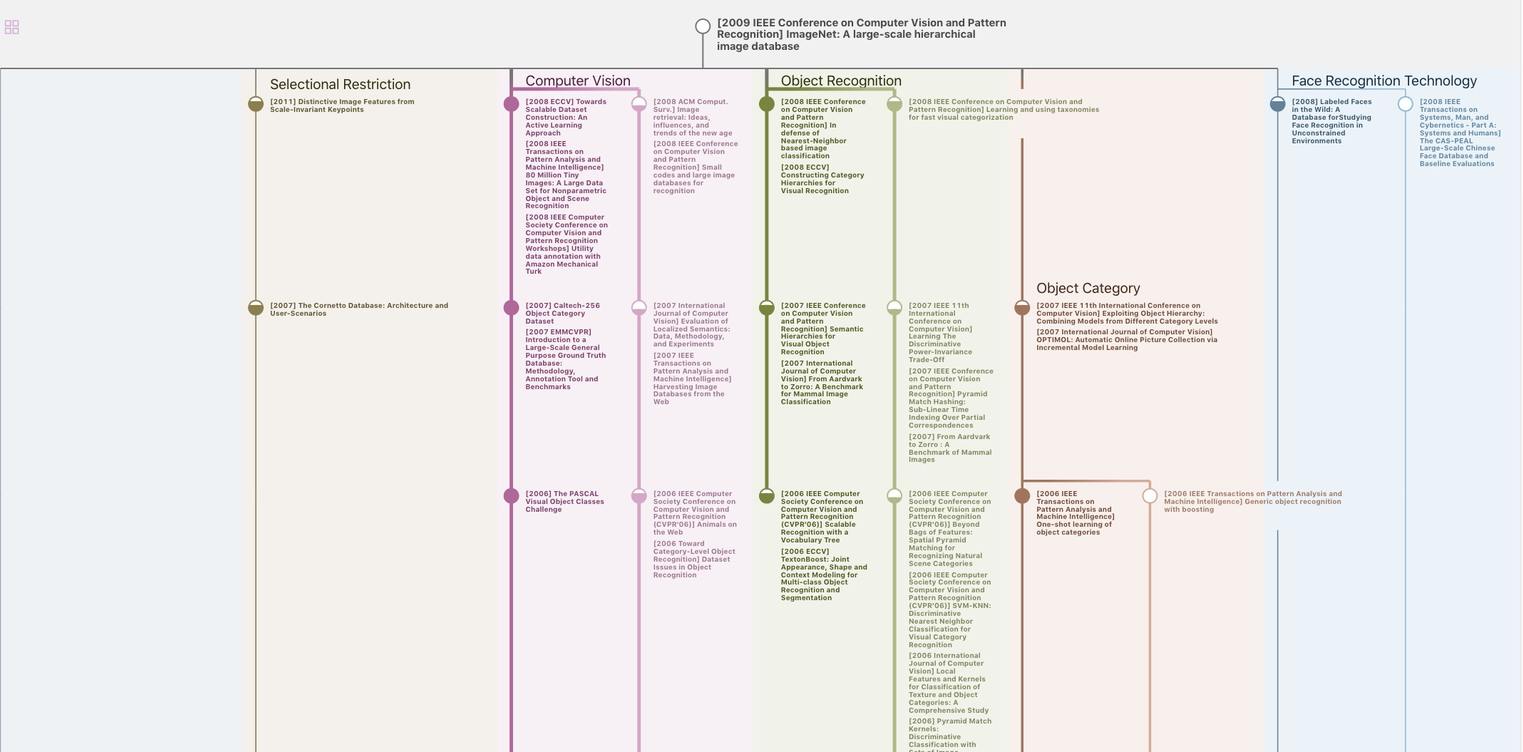
生成溯源树,研究论文发展脉络
Chat Paper
正在生成论文摘要