Efficient Off-Policy Algorithms for Structured Markov Decision Processes
2023 62ND IEEE CONFERENCE ON DECISION AND CONTROL, CDC(2023)
摘要
Reinforcement Learning (RL) algorithms help in training an autonomous agent to learn the optimal actions in an unknown environment. RL, in conjunction with neural networks, known as deep RL, has achieved remarkable success in many real-life practical applications. However, most of these algorithms are complex to train and require a lot of data to learn optimal decisions. Hence, it is imperative to develop RL algorithms that are simple and data efficient. In this work, we propose off-policy RL algorithms that can exploit special structures present in the optimal policy of the underlying Markov Decision process. The off-policy learning enables us to make use of the available data efficiently. To this end, we first propose an offpolicy algorithm that estimates the value function of only those policies with the optimal policy structure to determine the best policy. We then propose two novel off-policy algorithms utilizing Upper Confidence Bound (UCB) with less time and space complexity than our first algorithm. Through extensive experimental evaluations on RL benchmark tasks, we illustrate the efficacy of the proposed algorithms.
更多查看译文
AI 理解论文
溯源树
样例
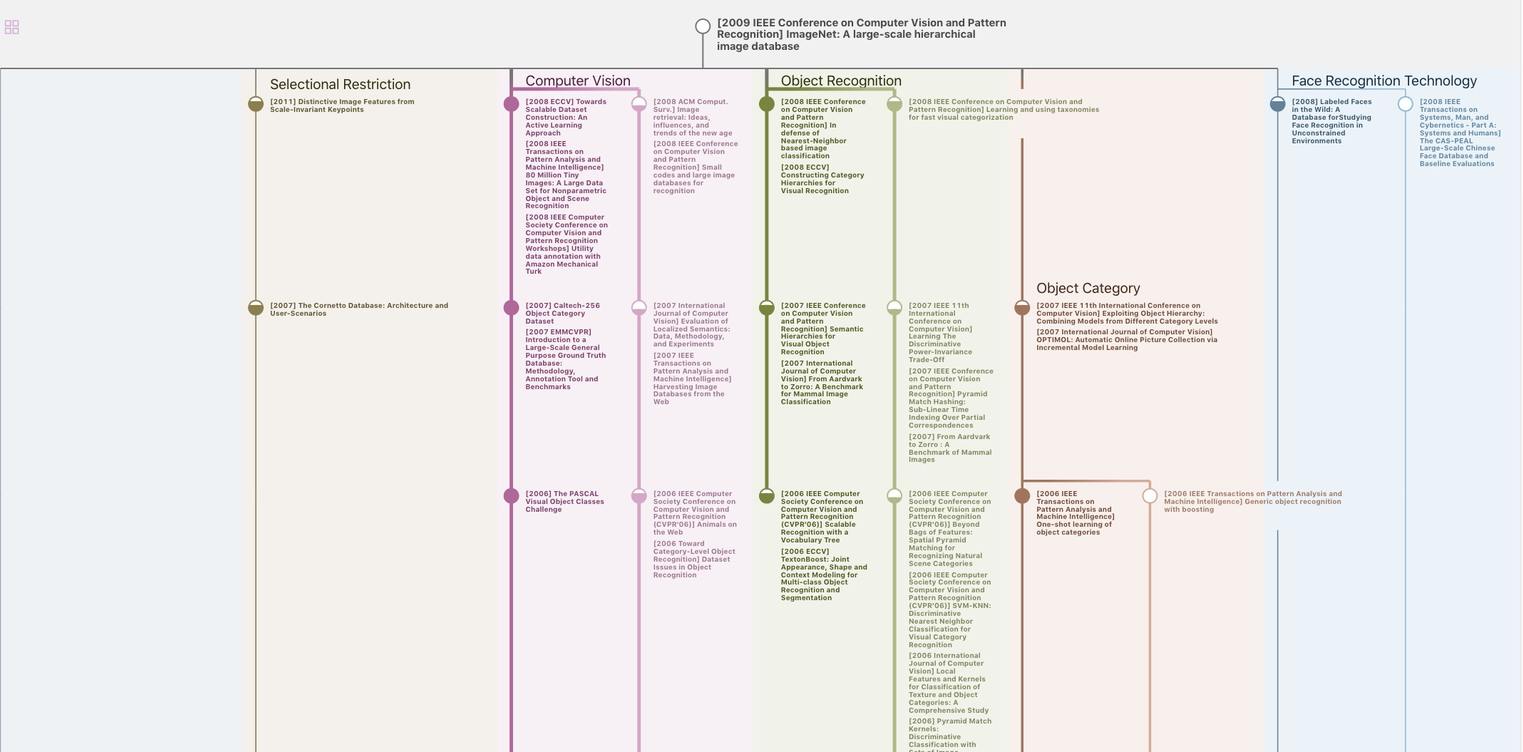
生成溯源树,研究论文发展脉络
Chat Paper
正在生成论文摘要