Global Resolution of Chance-Constrained Optimization Problems: Minkowski Functionals and Monotone Inclusions
2023 62ND IEEE CONFERENCE ON DECISION AND CONTROL, CDC(2023)
摘要
Chance-constrained optimization problems, an important subclass of stochastic optimization problems, are often complicated by nonsmoothness, and nonconvexity. Thus far, non-asymptotic rates and complexity guarantees for computing an epsilon-global minimizer remain open questions. We consider a subclass of problems in which the probability is defined as P {zeta vertical bar zeta is an element of K(x)}, where K is a set defined as K(x) = {zeta is an element of K vertical bar c(x, zeta) <= 1}, c(x, .) is a positively homogeneous function for any x is an element of X, and K is a nonempty and convex set, symmetric about the origin. We make two contributions in this context. (i) First, when zeta admits a log-concave density on K, the probability function is equivalent to an expectation of a nonsmooth Clarke-regular integrand, allowing for the chance-constrained problem to be restated as a convex program. Under a suitable regularity condition, the necessary and sufficient conditions of this problem are given by a monotone inclusion with a compositional expectation-valued operator. (ii) Second, when zeta admits a uniform density, we present a variance-reduced proximal scheme and provide amongst the first rate and complexity guarantees for resolving chance-constrained optimization problems.
更多查看译文
AI 理解论文
溯源树
样例
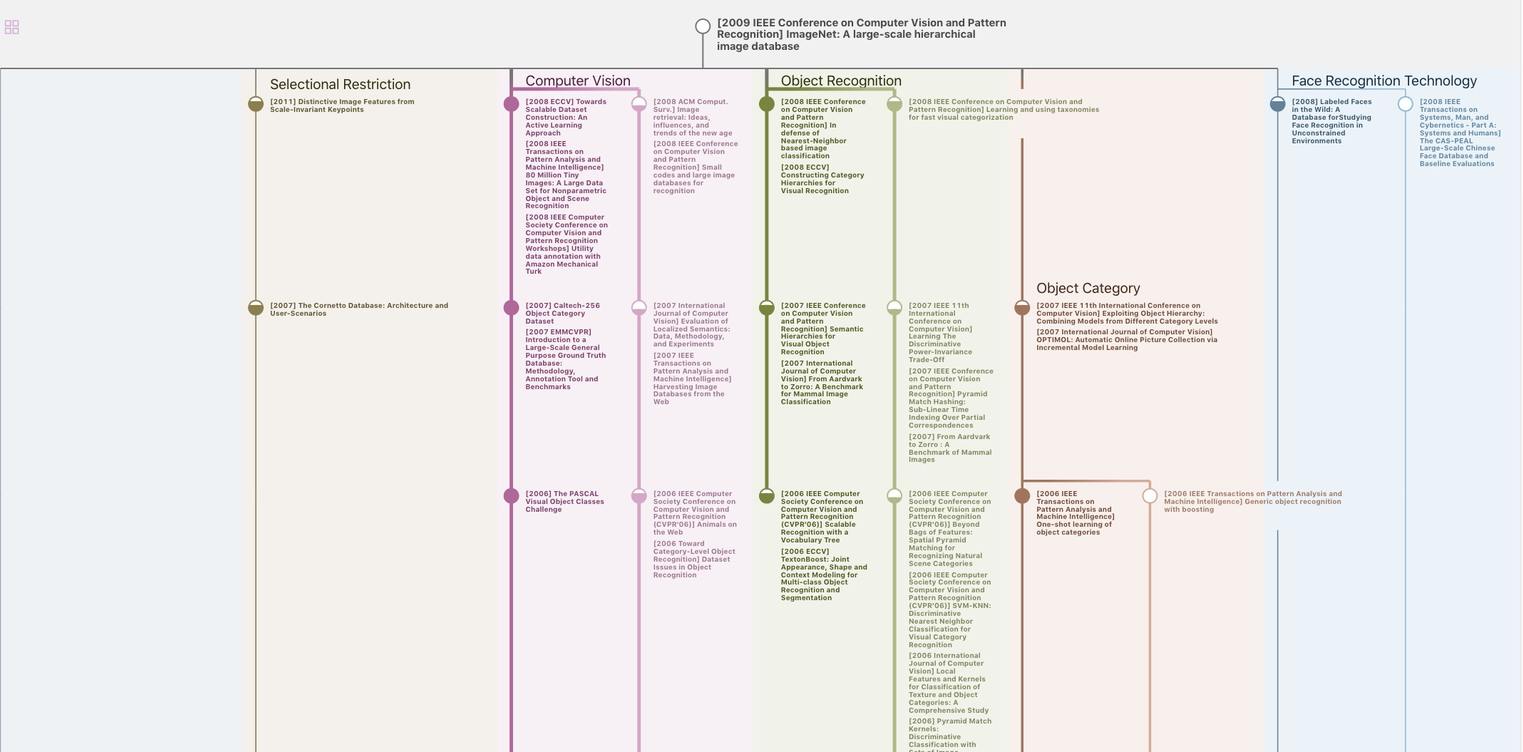
生成溯源树,研究论文发展脉络
Chat Paper
正在生成论文摘要