Adaptive Identification under Saturated Output Observations with Possibly Correlated and Unbounded Input Signals
2023 62ND IEEE CONFERENCE ON DECISION AND CONTROL, CDC(2023)
摘要
This paper considers adaptive identification and prediction problems for stochastic dynamical systems with saturated output observations, which arise from various problems in science and technology as well as in social and economic systems. A new adaptive algorithm is introduced, which avoids the projection operators used in the related existing work. More importantly, unlike most previous works that require independent and identically distributed conditions as well as bounded conditions on system signals, it is shown that the global convergence of the average regret and strong consistency of the parameter estimates can be established under possibly unbounded, correlated, and non-stationary signal conditions. A numerical example is also given to illustrate the effectiveness of the proposed adaptive algorithm.
更多查看译文
AI 理解论文
溯源树
样例
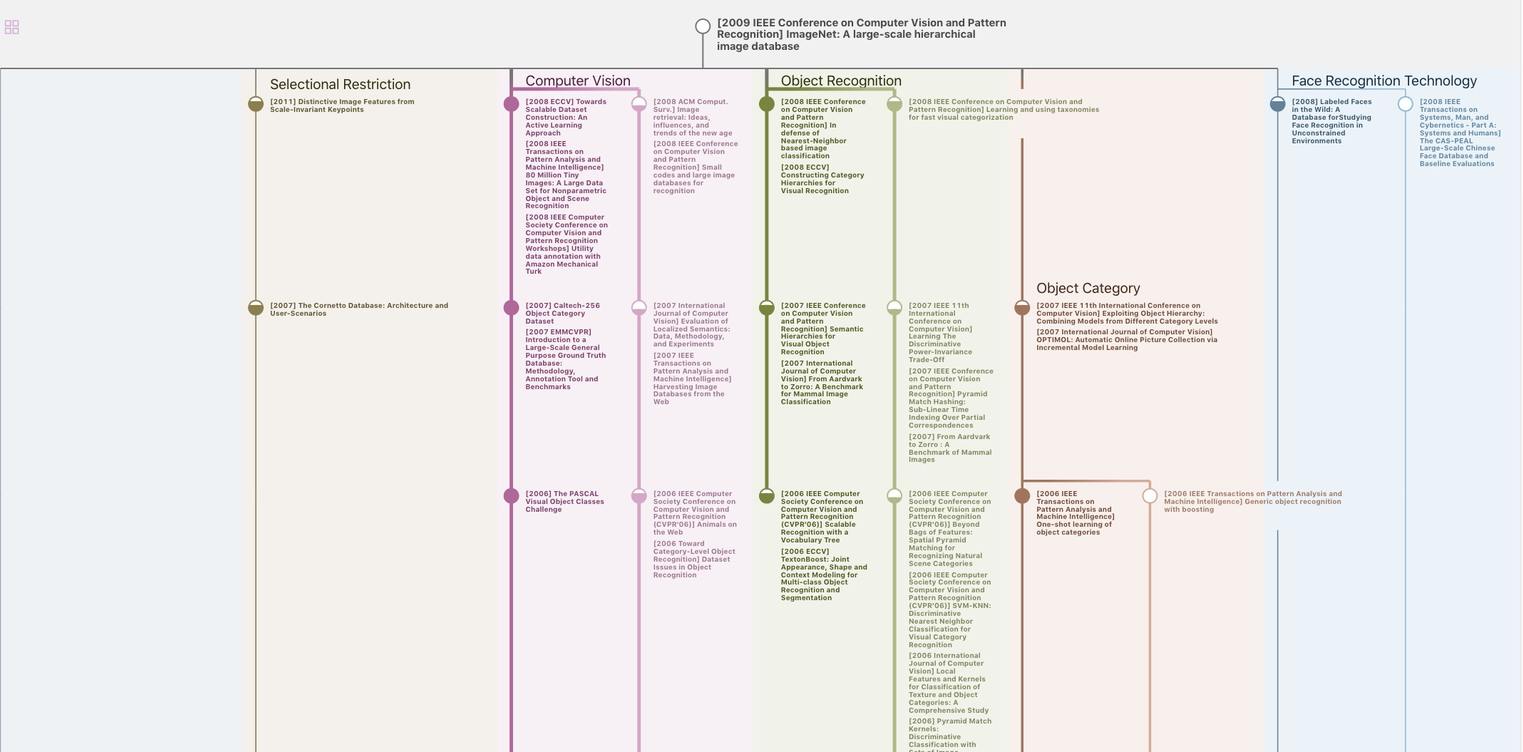
生成溯源树,研究论文发展脉络
Chat Paper
正在生成论文摘要