A distributed stochastic first-order method for strongly concave-convex saddle point problems
2023 62ND IEEE CONFERENCE ON DECISION AND CONTROL, CDC(2023)
摘要
In this paper, we propose a distributed stochastic first-order method for saddle point problems over strongly connected graphs. Existing methods generally suffer from a steady-state error that arises due to the heterogeneous nature of data distribution (captured by the local versus global cost gaps) and the variance of the stochastic gradients. We propose GT-SGDA, a distributed stochastic gradient descent ascent method that uses network-level gradient tracking to eliminate the steady-state error component due to the local versus global cost gap. We show that GT-SGDA converges linearly to an error ball around the unique saddle point for sufficiently small constant step-sizes when the global cost is strongly concave-convex (a necessary condition for the existence of a unique saddle point). Moreover, we show that the size of this error ball depends on the variance of the stochastic gradients. We provide numerical experiments to illustrate the convergence properties of GT-SGDA for different applications and highlight the significance of gradient tracking. We also show the performance of GT-SGDA for training modern applications like distributed generative adversarial networks (GANs).
更多查看译文
关键词
Stochastic min-max optimization,first-order methods,saddle point problems,distributed algorithms
AI 理解论文
溯源树
样例
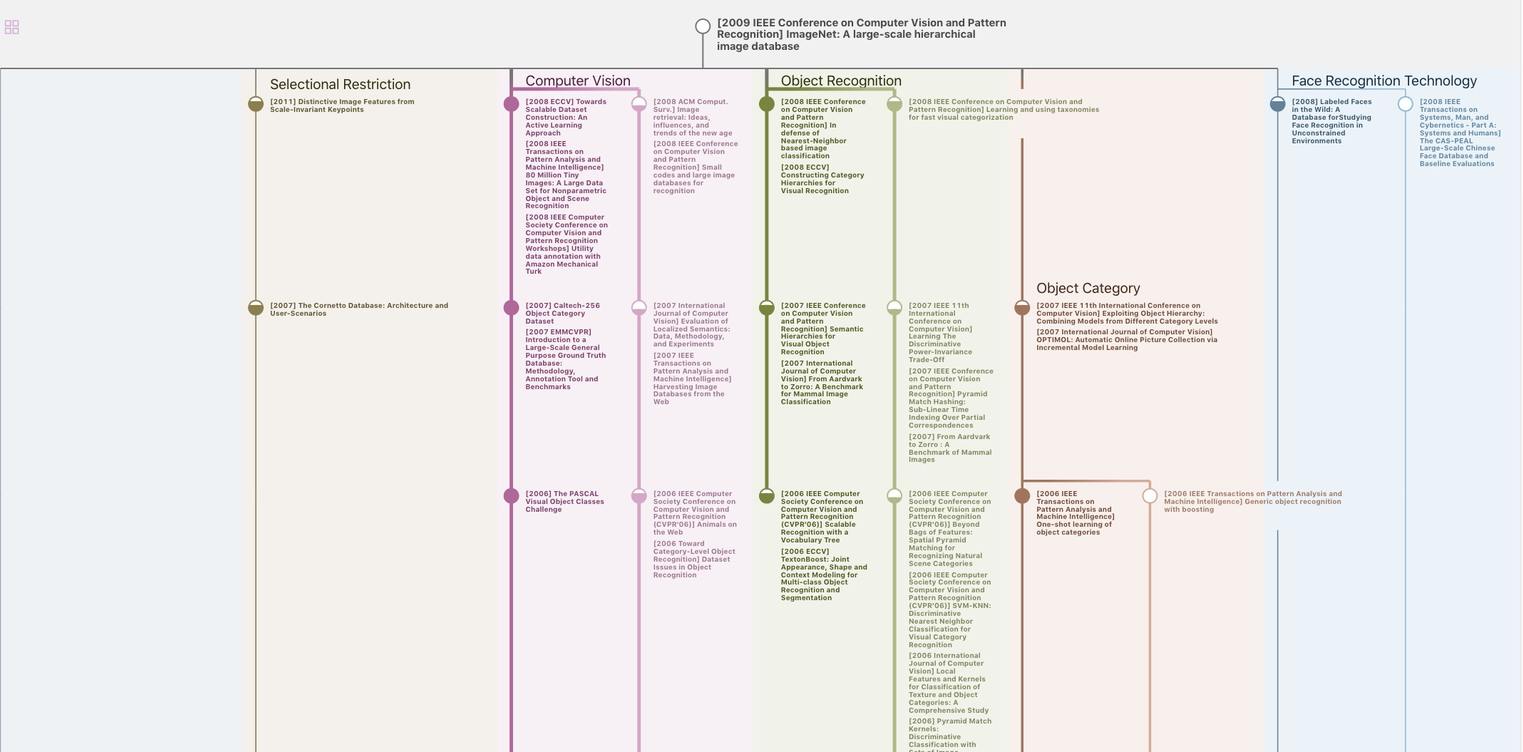
生成溯源树,研究论文发展脉络
Chat Paper
正在生成论文摘要