Approximate Model Predictive Control of Switched Affine Systems using Multitask Learning with Safety and Stability Guarantees
2023 62ND IEEE CONFERENCE ON DECISION AND CONTROL, CDC(2023)
摘要
We study the problem of designing an approximate model predictive control (MPC) for discrete time switched affine systems. The MPC design for the switched affine system requires an online solution of a mixed integer program. However, the combinatorial nature of the mixed integer problems might require a large computational time limiting its applicability in real time scenarios. To this end, we propose a framework based on the multitask learning paradigm to approximate the solution of mixed integer MPC for switched affine systems. We also provide a computational method to overapproximate the reachable sets of the closed-loop system that helps to analyze the safety and stability of the system under the influence of the learned controller. Once trained offline, the resulting controller results in a solver free approach especially suited for implementation on resource constrained embedded hardware. We demonstrate the efficacy of the approach on a real world example of an induced draft cooling tower.
更多查看译文
AI 理解论文
溯源树
样例
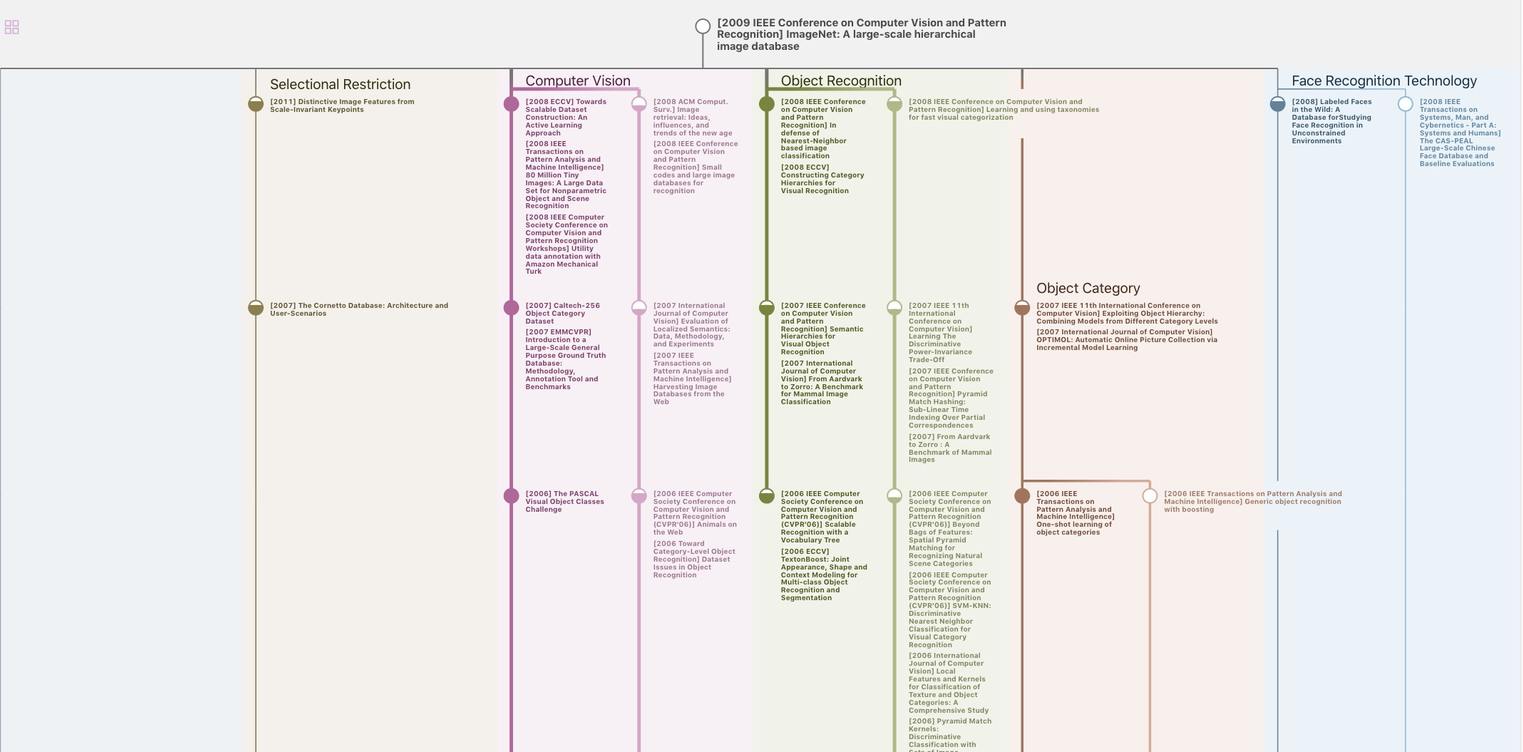
生成溯源树,研究论文发展脉络
Chat Paper
正在生成论文摘要