Tilted Least-Squares Parameter Estimation of Linear Regression Models in the Presence of Outliers
2023 62ND IEEE CONFERENCE ON DECISION AND CONTROL, CDC(2023)
摘要
The least squares estimator is the most popular identification method. In the absence of prior knowledge on the unknown noise, uniform weights on all samples are often assumed. In reality, potentially unknown contamination is always present and the uniform weights are not necessarily the best. Further, explicit information about the nature of contamination is usually absent. To this end, a relaxed-tilted least squares method is proposed here to assign unequal weights so that the effect of undesired noise contamination can be mitigated. The relaxed-tilted least squares method tilts the uniform prior on the samples so as to move the uniform distribution in a direction that enjoys the smallest estimation error in the neighborhood of the uniform distribution. Theoretical results are established including the ability of outlier removal and the guaranteed parameter convergence in the presence of outliers. Numerical algorithms are proposed and simulated, which support the theoretical derivations.
更多查看译文
关键词
Robust least squares,Outliers,Heavy-tailed noises,System identification,Parameter estimation
AI 理解论文
溯源树
样例
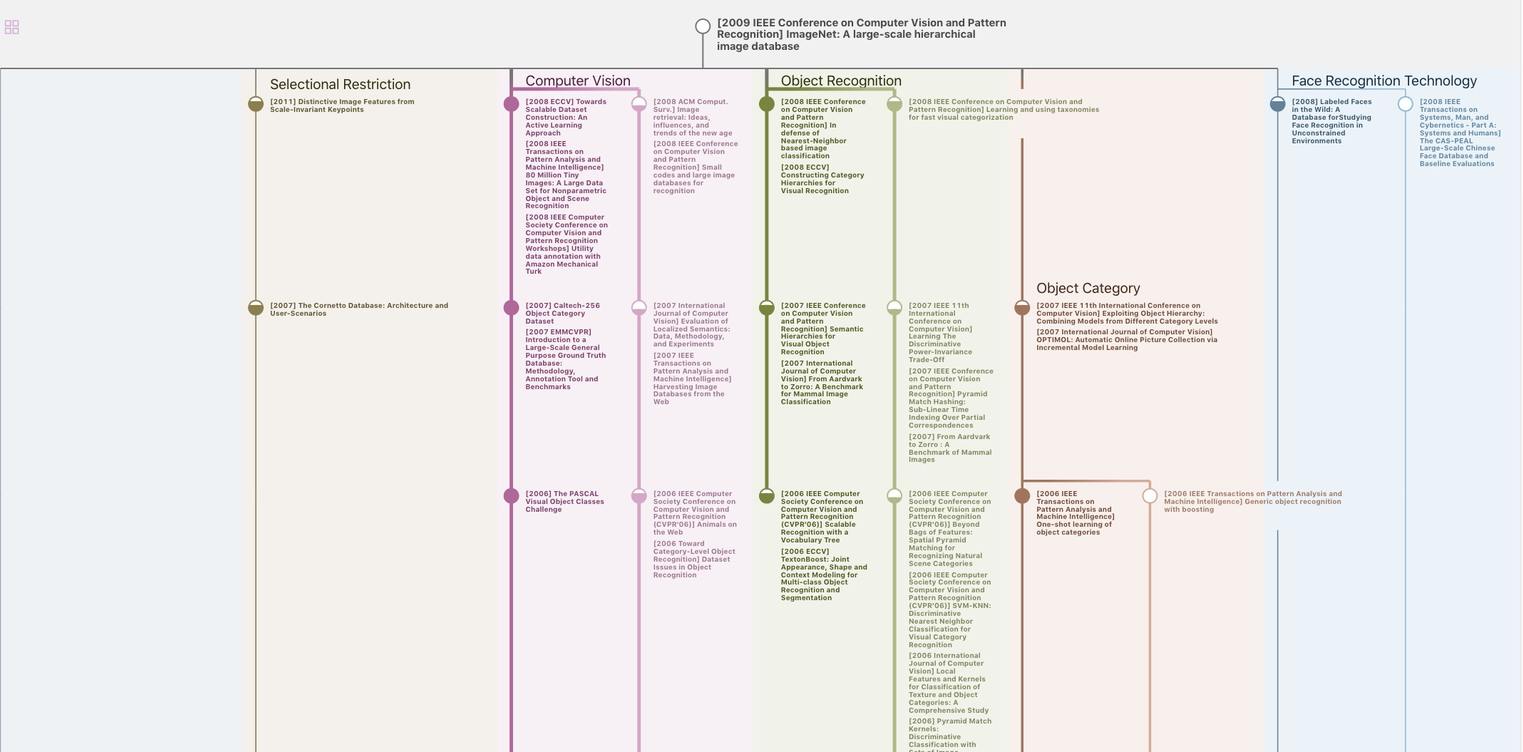
生成溯源树,研究论文发展脉络
Chat Paper
正在生成论文摘要