AI-based Models to Predict the Heart Rate Using PPG and Accelerometer Signals During Physical Exercise.
2023 IEEE International Conference on Bioinformatics and Biomedicine (BIBM)(2023)
摘要
PPG signal is a valuable resource for continuous heart rate monitoring; however, this signal suffers from artifact movements, which is particularly relevant during physical exercise and makes this biomedical signal difficult to use for heart rate detection during those activities. The purpose of this study was to develop learning models to determine heart rate using data from wearables (PPG and acceleration signals) and dealing with noise during physical exercise. Learning models based on CNNs and LSTMs were developed to predict the heart rate. The PPG signal was combined with data from accelerometers trying to overcome the noise movement on the PPG signal. Two datasets were used on this work: the 2015 IEEE Signal Processing Cup (SPC) dataset was used for training and testing, and another dataset was used for validation of the learning model (PPG-DaLiA dataset). The predictions obtained by the learning model represented a mean average error of 7.033±5.376 bpm for the SCP dataset, while a mean average error of 9.520±8.443 bpm for the validation set. The use of acceleration data increases the performance of the learning models on the prediction of the heart rate, showing the benefits of using this source of data to overcome the noise movement problem on the PPG signal. The combination of PPG signal with acceleration data could allow the learning models to use more information regarding the motion artifacts that affect the PPG and improve performance on the physiological event detections, which will largely spread the use of wearables on the healthcare applications for continuous monitor the physiological state allowing early and accurate detection of pathological events.
更多查看译文
关键词
Convolutional Neural Network,Long Short Term Memory,Photoplethysmography,Motion Artifacts,Heart Rate Estimation
AI 理解论文
溯源树
样例
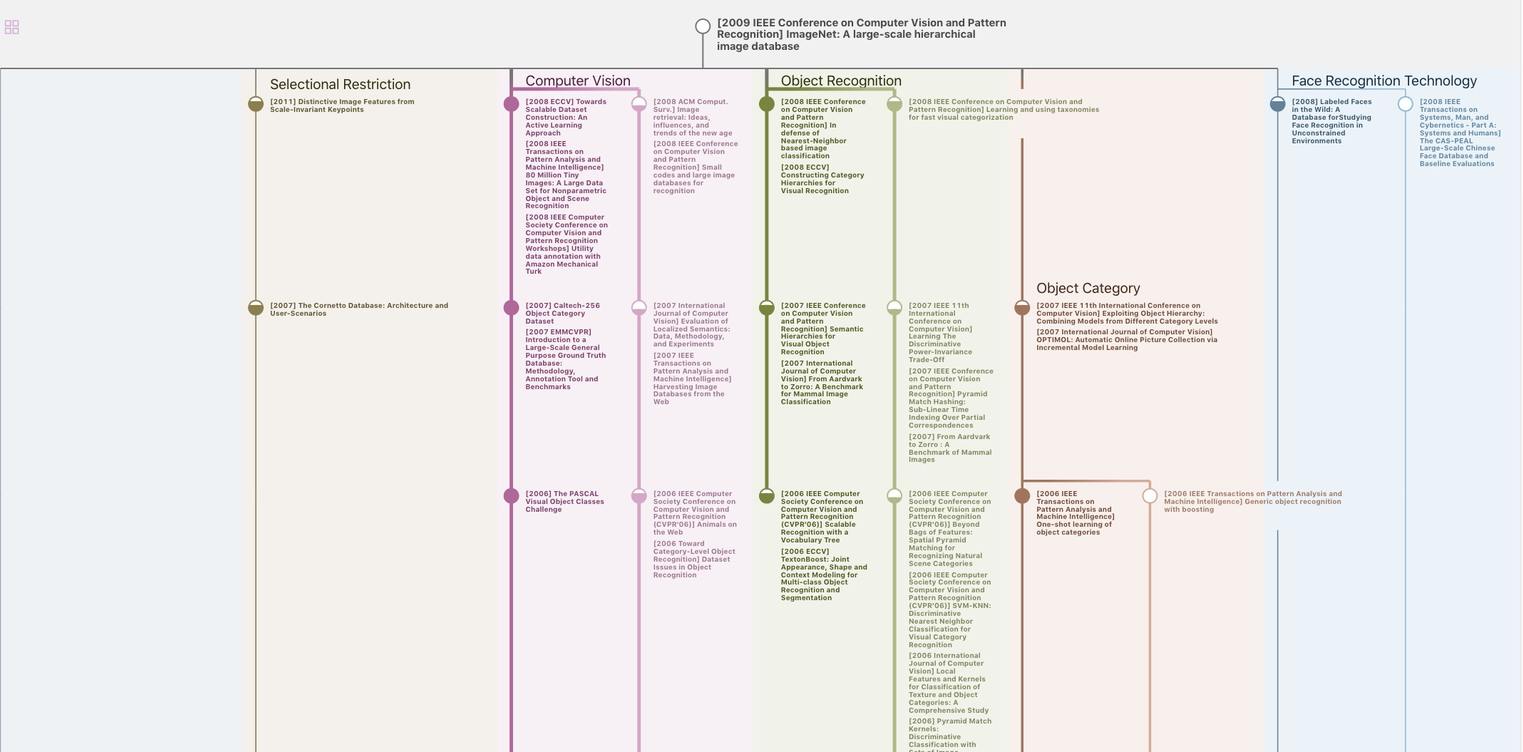
生成溯源树,研究论文发展脉络
Chat Paper
正在生成论文摘要