DFNodule: a Novel Deformable Faster R-CNN for Lung Nodule Detection.
2023 IEEE International Conference on Bioinformatics and Biomedicine (BIBM)(2023)
摘要
In computer-aided diagnosis systems, lung nodule detection plays a crucial role in the overall framework. In this work, we propose a new three-dimensional deformable convolutional neural network (dcnn) method for lung nodule detection based on the Faster R-CNN framework. We incorporate deformable convolutions to design a hybrid convolutional module, which enhances feature extraction in the lung nodule detection model. By leveraging the deformable convolutions’ characteristics, the network is capable of capturing the diverse morphological variations of lung nodules, addressing challenges such as large morphological variations and the inability to capture unified image features. This improves the accuracy of the lung nodule detection algorithm. Additionally, we employ a second-stage network to further discriminate suspected nodules, which enhances the recognition of non-nodule tissues and reduces false positive nodules. To comprehensively evaluate the performance of various lung nodule detection models, we conducted experiments using the publicly available LUNA16 dataset. Our method surpasses other detection algorithms in terms of CPM, achieving a 1.5% improvement. Particularly, the nodule recognition rate is significantly improved at lower false positive rates. In addition, in other metrics such as F1-score, AP, we also achieved 0.6%, 2% improvement.
更多查看译文
关键词
Lung Nodule Detection,Faster R-CNN,Deformable Convolution
AI 理解论文
溯源树
样例
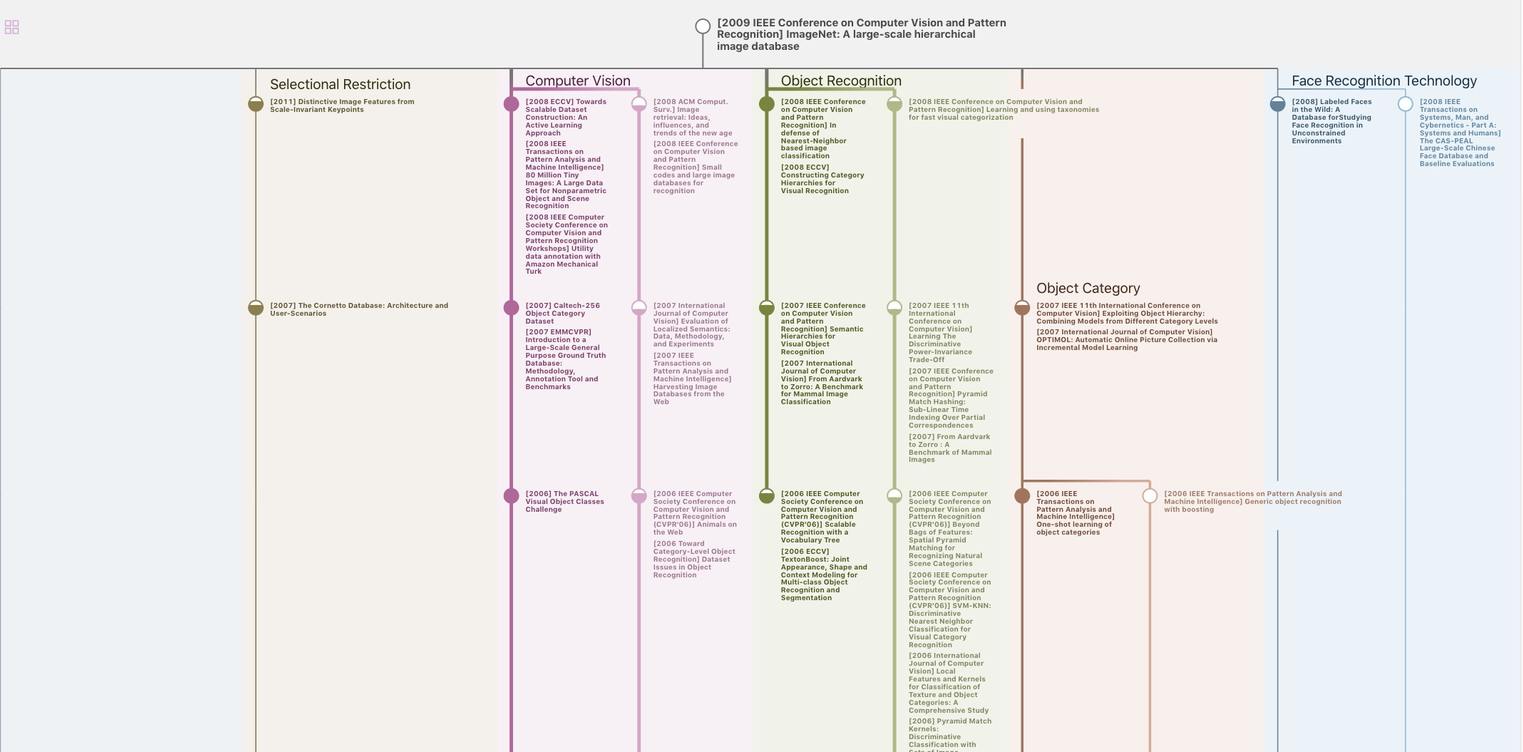
生成溯源树,研究论文发展脉络
Chat Paper
正在生成论文摘要