Effective Surrogate Models for Docking Scores Prediction of Candidate Drug Molecules on SARS-CoV-2 Protein Targets.
2023 IEEE International Conference on Bioinformatics and Biomedicine (BIBM)(2023)
摘要
Emerging infectious diseases, such as coronavirus disease 2019 (COVID-19), pose a major threat to public health and present a critical challenge for drug discovery. Due to the cost- and time-consuming process of new drug development, virtual pre-screening methods such as protein-ligand docking prediction have become essential tools in enhancing drug refurbishment and repurposing. In this study, we propose a machine learning-based surrogate model for docking score prediction of drug candidates on SARS-CoV-2 protein targets via deep feature concatenation. We investigate 14 different combinations of rule-based and data-driven fingerprinting methods to identify the optimal representation of candidate drug molecules. Extensive experiments on docking scores of 270,000 molecules across 18 different SARS-CoV-2 protein targets demonstrate the effectiveness of the proposed surrogate models. In addition to unseen drugs, we further investigate the generalization of the proposed framework for unseen protein targets. This study may provide an instrumental and generalizable framework for exploring ligand-protein interaction, serving as a useful tool to facilitate rapid drug pre-screening during emerging public health crises.
更多查看译文
关键词
drug target,interaction prediction,neural networks,feature concatenation,explainable artificial intelligence,COVID-19
AI 理解论文
溯源树
样例
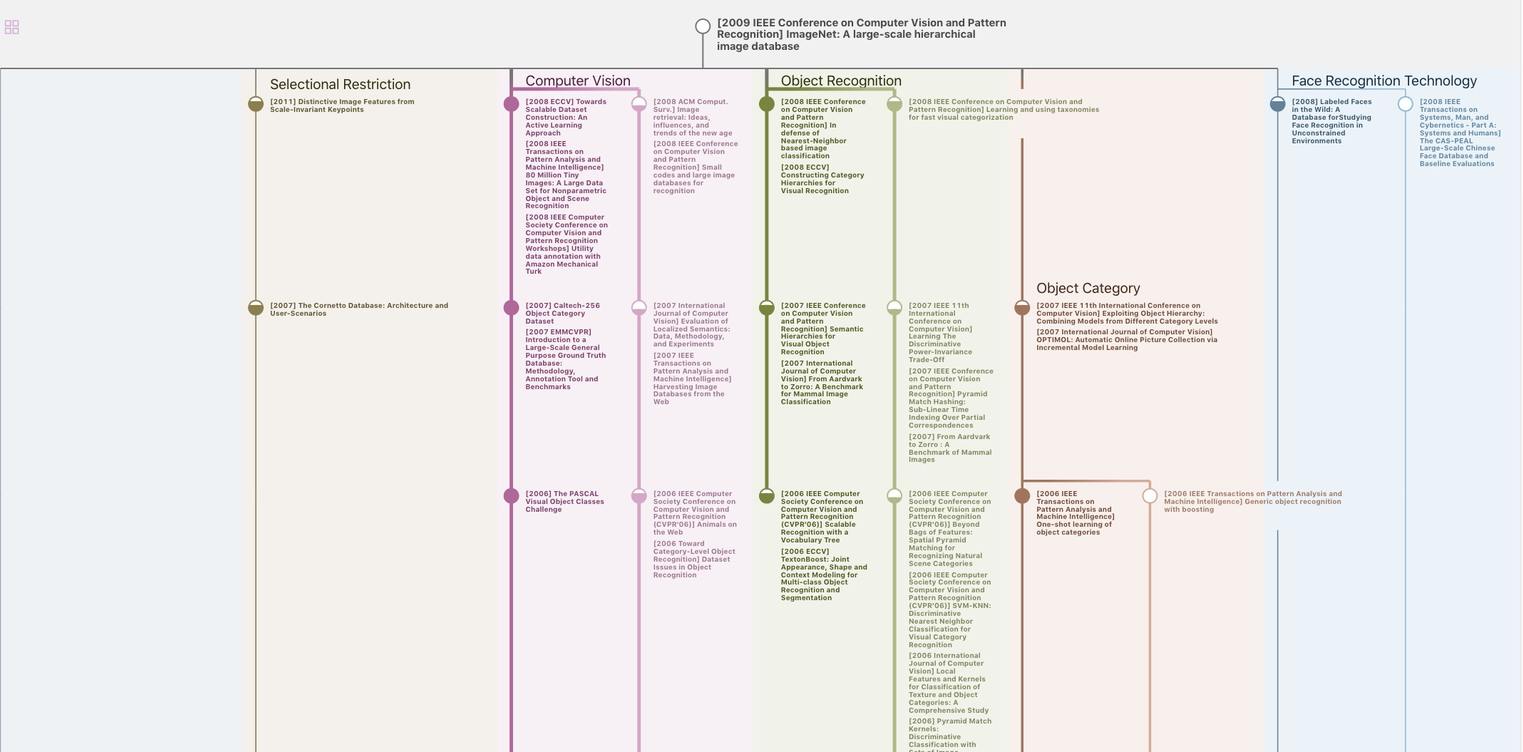
生成溯源树,研究论文发展脉络
Chat Paper
正在生成论文摘要