Towards Trustworthy Collaborative Healthcare Data Sharing.
2023 IEEE International Conference on Bioinformatics and Biomedicine (BIBM)(2023)
摘要
The integration of healthcare and data-driven technologies offers remarkable opportunities for medical research and patient care. However, it is crucial to adhere to the ethical responsibility of protecting patient data and maintaining robust privacy standards as the primary concern. Federated learning (FL) has been recognized as a promising technique to address this issue. FL allows multiple healthcare providers or institutions to collaboratively train machine learning models without the necessity of directly exchanging sensitive patient data. Nevertheless, using conventional FL methods, which rely extensively on centralized aggregators, poses significant challenges within healthcare, including privacy vulnerabilities, regulatory compliance, and the potential for malicious exploits. In order to address the above challenges, this paper presents the Cross-Silo Federated Learning Framework with Blockchain and Differential Privacy (CSFL-BDP), a framework solution designed to enhance the privacy and security of data in healthcare systems. The CSFL-BDP framework addresses these concerns by enabling collaborative model training across healthcare institutions while obviating the need for centralized aggregation. By leveraging blockchain technology, the CSFL-BDP framework ensures data integrity, immutability, decentralized control, and patient records protection. Furthermore, it integrates differential privacy techniques to protect sensitive medical information during collaborative model training. This framework has the potential to transform healthcare analytics in practical settings, enabling institutions to collaboratively enhance medical knowledge while maintaining patient data security and privacy.
更多查看译文
关键词
federated learning,blockchain,differential privacy,privacy-preserving,healthcare
AI 理解论文
溯源树
样例
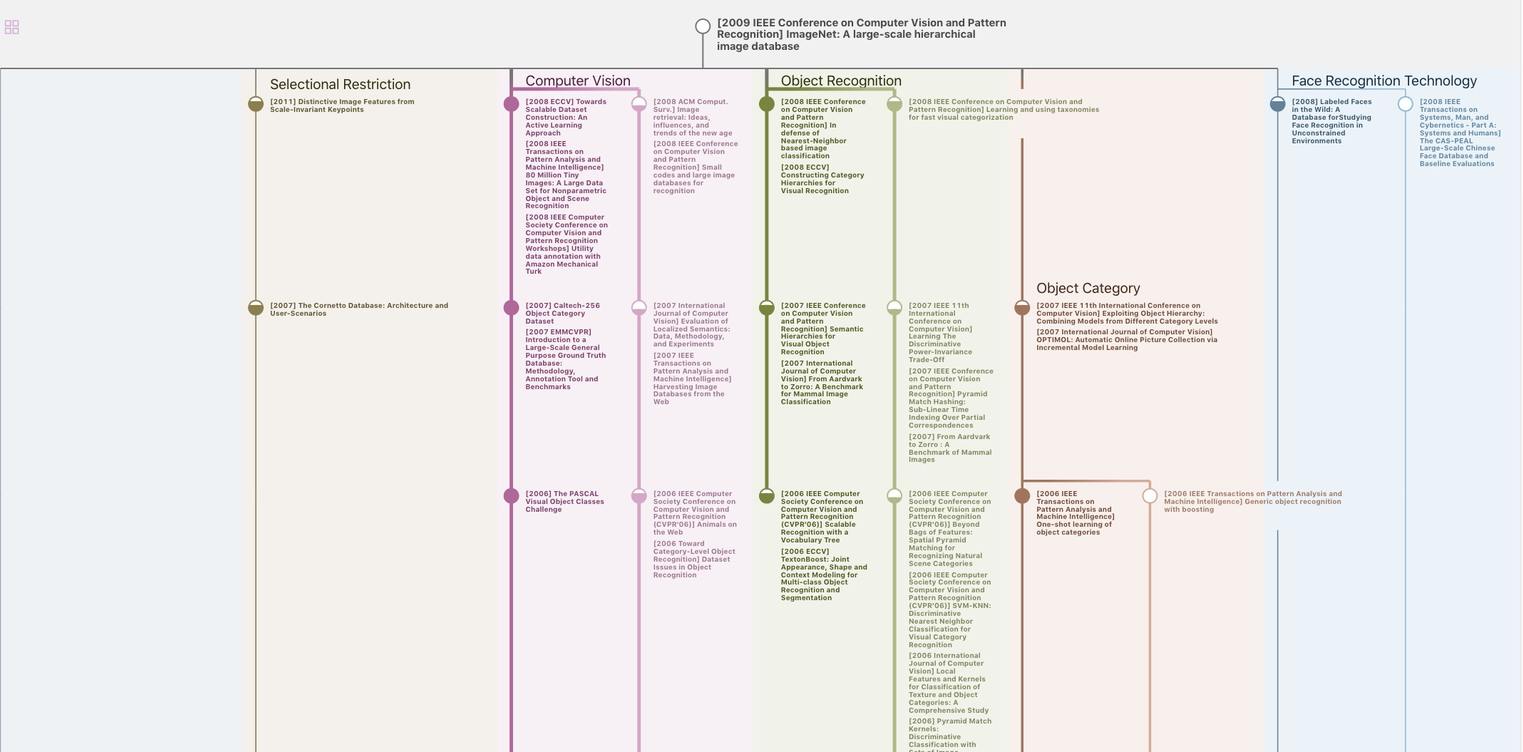
生成溯源树,研究论文发展脉络
Chat Paper
正在生成论文摘要