Prediction of Drug Targets based on In Vitro Activity Profiles Toward Drug Repurposing for Rare Diseases.
2023 IEEE International Conference on Bioinformatics and Biomedicine (BIBM)(2023)
摘要
Over 300 million people are suffering from rare diseases, most of which have limited treatment options. Therefore, discovering new treatments for rare diseases is imperative. Drug repurposing, which identifies new uses for approved drugs, is considered one of the viable and risk-managed strategies for disease treatments. To promote the drug repurposing process, we introduced a prediction model to uncover novel relationships between gene targets and chemical compounds. In our previous study, we identified enriched genes for compounds from the Toxicology in the 21st Century program (Tox21) 10K library, to extend that study for enriched gene target prediction, we developed machine learning (ML) models including Support Vector Machine; K-Nearest Neighbors; Random Forest; and extreme gradient boosting (XGBoost), by using Tox21 bioassay screening data. All four models perform well with f1_score over 0.7, and XGBoost has the best performance with four different multi-label prediction embedding algorithms, including Binary Relevance; Label Powerset; Classifier Chain; Multi-Output Classifier. Our study explored a reliable method to predict potential gene targets from in vitro activity profile data toward drug repurposing.
更多查看译文
关键词
Tox21,Machine Learning,Gene targets,Drug repurposing
AI 理解论文
溯源树
样例
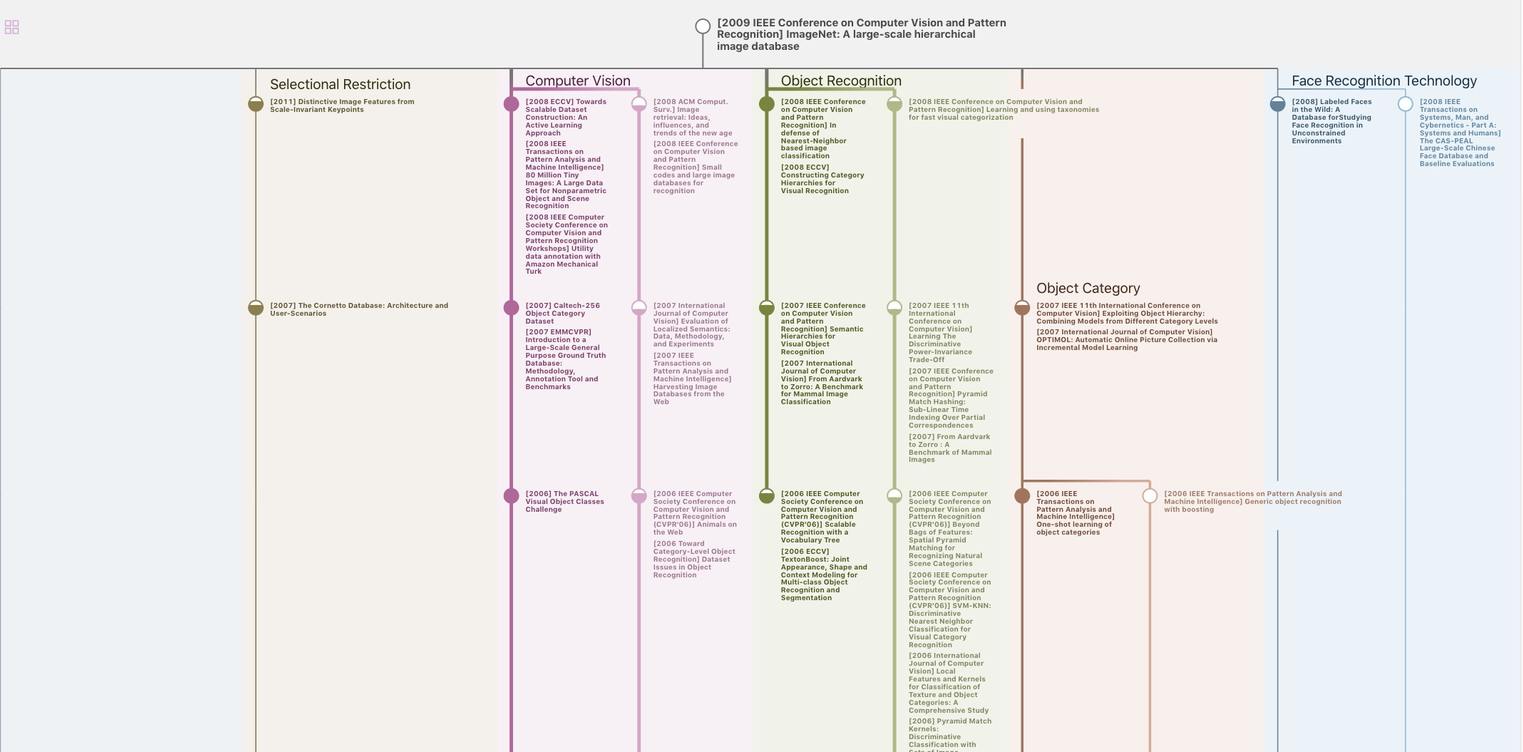
生成溯源树,研究论文发展脉络
Chat Paper
正在生成论文摘要