Graph Convolutional Network with Neural Inductive Matrix Completion for Predicting Disease-Related LncRNA Genes.
2023 IEEE International Conference on Bioinformatics and Biomedicine (BIBM)(2023)
摘要
Numerous researches emphasized that long non-coding RNA (lncRNA) plays a vital factor in various biological processes, and its mismatched expression and dysfunction are tightly linked with the occurrence of human diseases. Thus, computational models were designed to identify lncRNA-disease interactions by merging heterogeneous biological data. However, most of them neglected the intrinsic structure of multi-source information, which limits the performance for potential lncRNA-disease association prediction. Here, GCN-NIMC is introduced to alleviate the dilemma for disease-associated lncRNA genes identification based on the graph convolutional network with neural inductive matrix. This method builds a feature matrix with multi-source heterogeneous data and then learn the various information contained in the feature matrix for the sake of acquiring better feature expressions of the lncRNA-disease interactions. Experimental results on 10-repeated 5-fold cross-validation demonstrated that our proposed GCN-NIMC is superior to existing cutting-edge methods for identifying disease-related lncRNA genes. Furthermore, case studies confirmed our computational method as a practical tool with clinical benefits to develop the therapeutic schedule at lncRNA-level.
更多查看译文
关键词
graph convolutional network,inductive matrix completion,lncRNA-disease association,gene identification
AI 理解论文
溯源树
样例
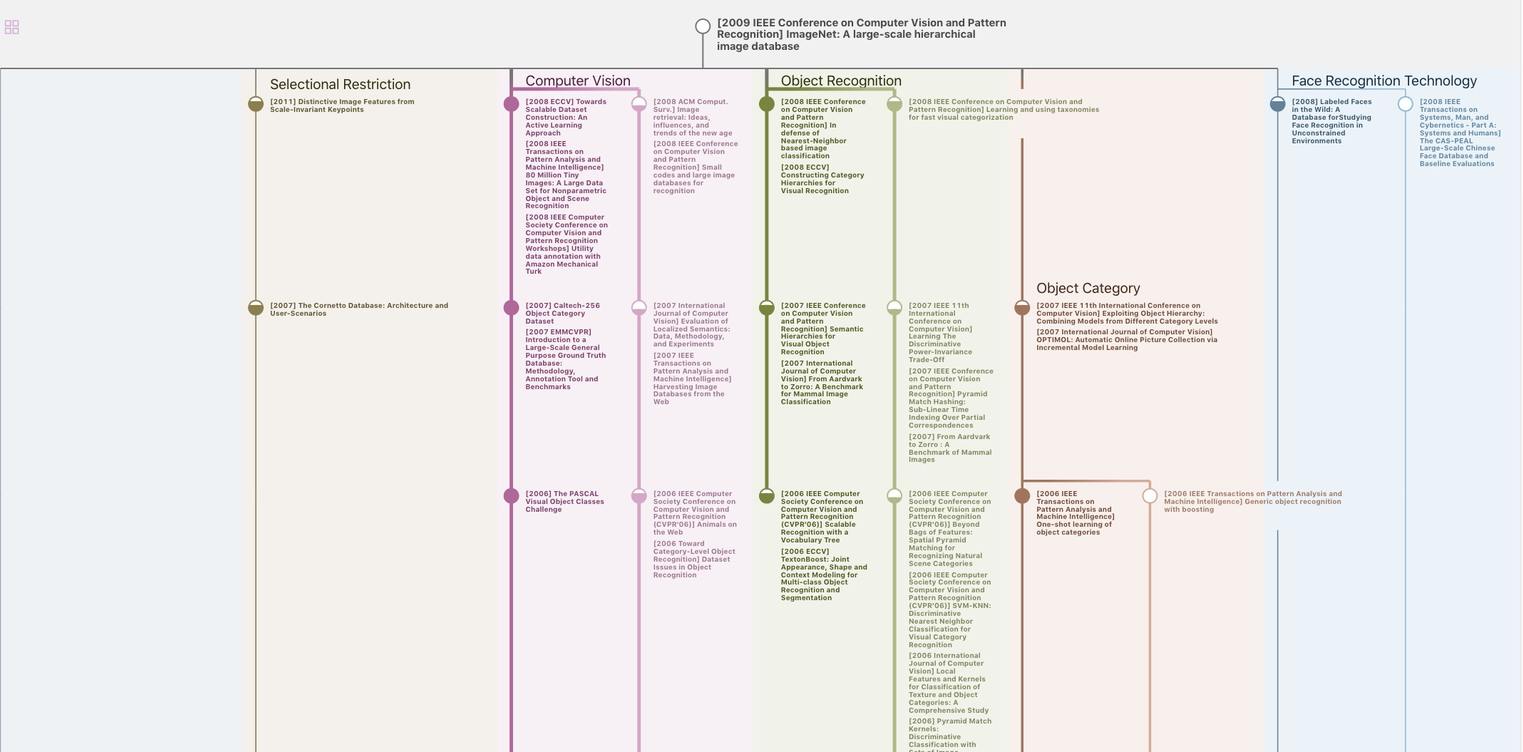
生成溯源树,研究论文发展脉络
Chat Paper
正在生成论文摘要