Identifying Disease-related Brain Imaging Quantitative Traits and Related Genetic Variations via A Bidirectional Association Learning Method.
2023 IEEE International Conference on Bioinformatics and Biomedicine (BIBM)(2023)
Abstract
Discovering critical genetic biomarkers of Alzheimer’s disease (AD) by detecting the complex associations between genotypes (i.e. single nucleotide polymorphism, SNP) and phenotypes (i.e. quantitative trait, QT) is a long-standing and beneficial task for the diagnosis and the follow-up treatment of patients. The function of genes and their relationships with phenotypes are extremely complex. A lot of imaging genetic methods have been designed to uncover the association between brain imaging QTs and SNPs. However, most of them are focused on the effect of a single SNP, which may have limited ability due to the oligogenic or polygenic characteristic of AD. In this paper, we propose a deep reconstruction bidirectional association with feature selection (DRBA-FS) method to explore the multi-SNPmulti-QT associations. In this method, the co-effect of multiple AD-related genetic variations is identified and aggregated, and their high-level genetic associations to brain imaging QTs are jointly modeled. Experiment results on real neuroimaging genetic data from Alzheimer’s Disease Neuroimaging Initiative (ADNI) show that the identified biomarkers are all related to AD. Interestingly, our method can learn the joint effect of multiple AD-related genetic variations across the genome, and thus has significant potential in understanding the genetic mechanism of AD.
MoreTranslated text
Key words
Brain imaging genetics,co-effect of genetic variations,feature selection,Alzheimer’s disease
AI Read Science
Must-Reading Tree
Example
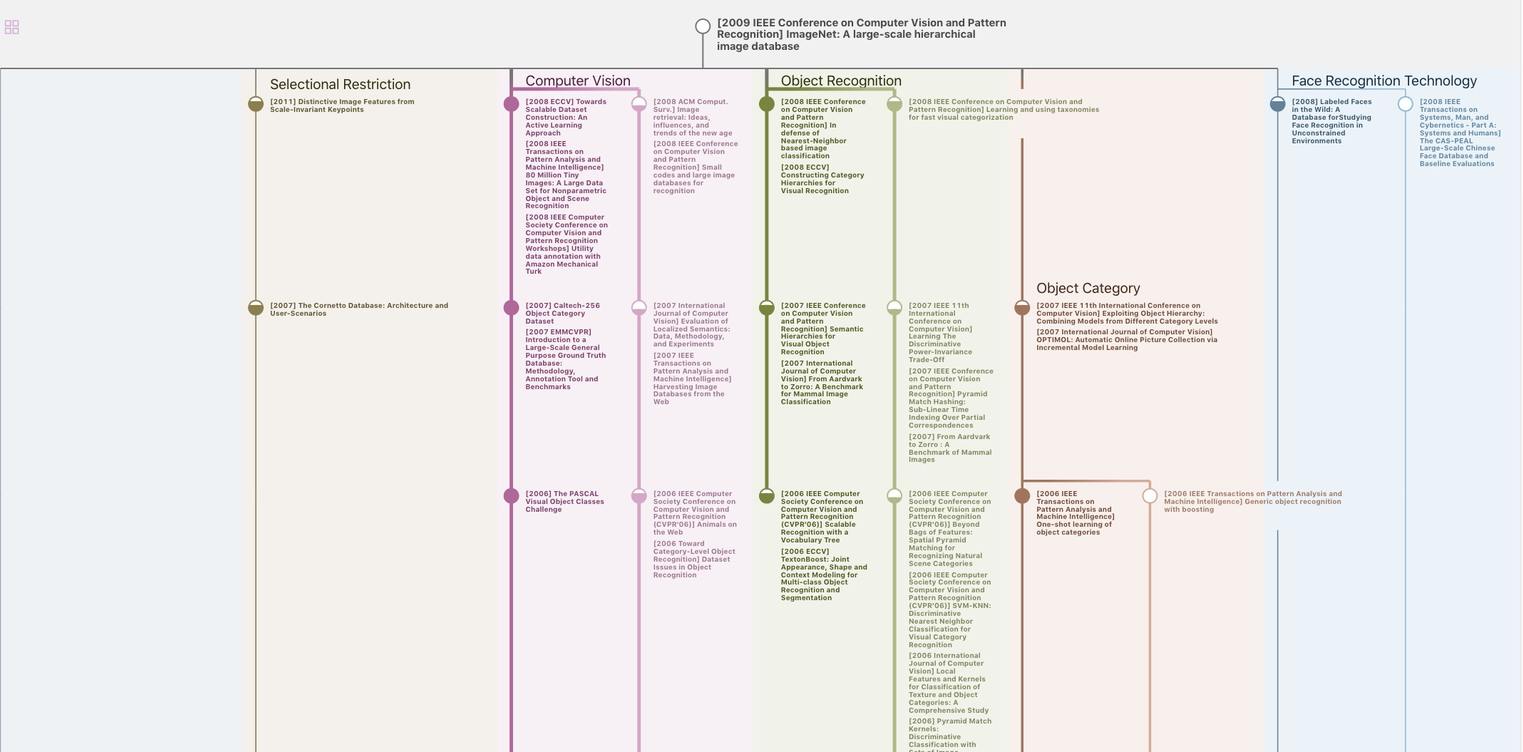
Generate MRT to find the research sequence of this paper
Chat Paper
Summary is being generated by the instructions you defined