Advancing Pancreas Segmentation through the Patch-Adjust Fusion Framework.
2023 IEEE International Conference on Bioinformatics and Biomedicine (BIBM)(2023)
摘要
Pancreas segmentation is pivotal for the timely diagnosis of pancreatic diseases, but it remains challenging due to organ’s small size, large spatial variations, and unclear boundaries. To enhance segmentation accuracy, researchers have explored various multi-modal coarse-to-fine (MMCF) methods, integrating distinct data-related factors such as stage, view, scale, and patch size. However, these methods often ask for multiple trained models, leading to significant storage and computational expenses. Moreover, these methods frequently treat different data-related factors separately, overlooking their potential relationships and constraining the feature representation capability of convolutional neural networks. To tackle these issues, we propose a data-efficient framework known as Patch-Adjust Fusion (PAF). PAF framework addresses these factors concurrently through dynamic patch flow adjustments, seamlessly integrating diverse elements while accounting for their interactions and dependencies. Consequently, the PAF framework demonstrates superior segmentation performance, diminished storage requisites, and expedited inference speeds compared to the MMCF framework. This is exemplified across two public CT pancreas datasets (NIH and MSD) and a private MR pancreas dataset (MR300). These results underscore its suitability for clinical applications, positioning the PAF framework as a promising solution for accurate and efficient pancreas segmentation in medical settings.
更多查看译文
关键词
multi-model coarse-to-fine,patch-adjust fusion,pancreas segmentation
AI 理解论文
溯源树
样例
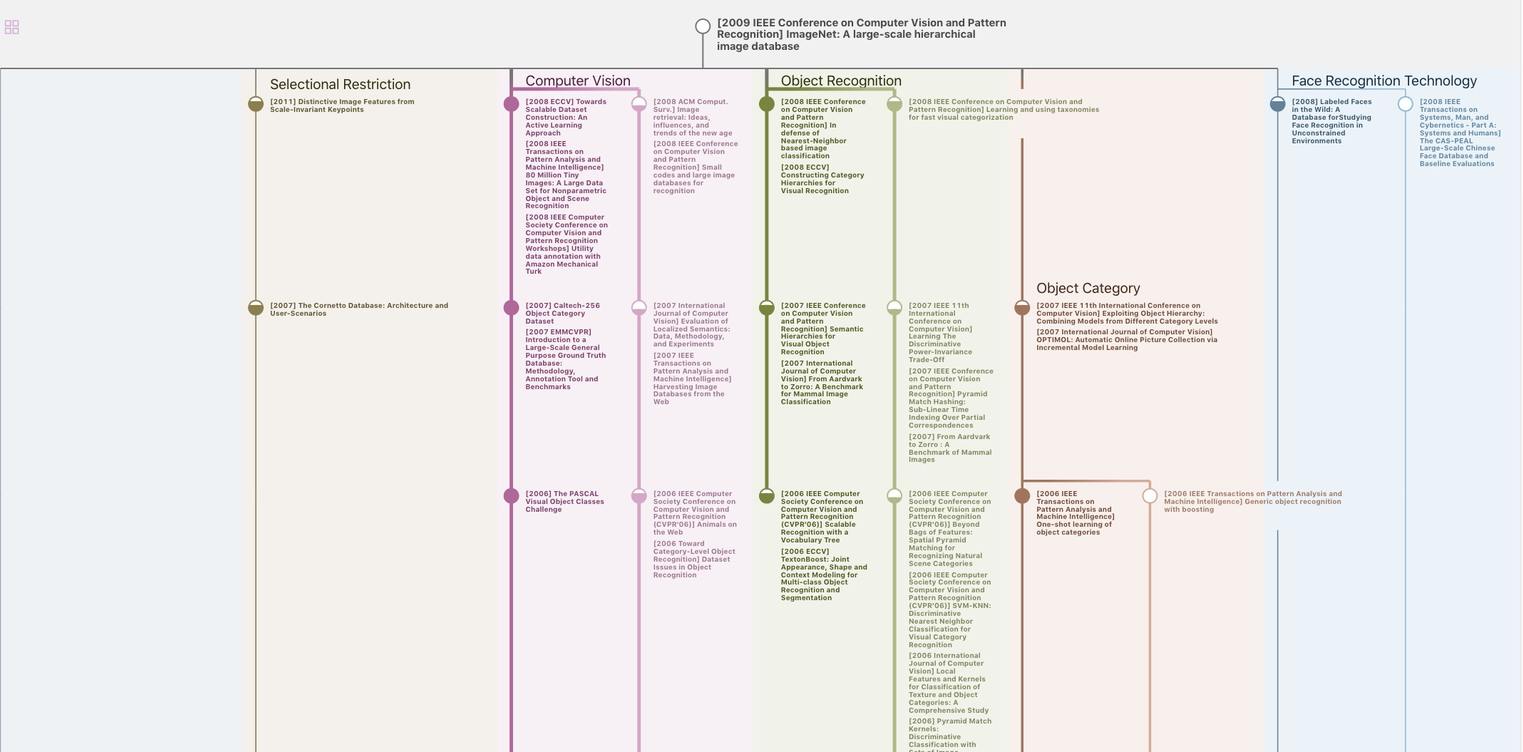
生成溯源树,研究论文发展脉络
Chat Paper
正在生成论文摘要