PLFormer: Prompt Learning for Early Warning of Unplanned Extubation in ICU.
2023 IEEE International Conference on Bioinformatics and Biomedicine (BIBM)(2023)
摘要
Patients’ Unplanned Extubation (UEX) behaviors in ICU have adverse effects on their postoperative recovery. Therefore, it is necessary to design a early warning systems to detect UEX tendency. However, the fineness and rapidity of UEX behaviors, coupled with the complexity of the ICU environment, renders the utilization of RGB monitory videos for early warning extremely challenging. To address the aforementioned challenges, we propose a PLFormer to make early warning of UEX behaviors in ICU by using the prompt learning approach. Specifically, we provide click prompts to the Track Anything model (TAM) with the ability to segment and track patient regions, producing a mask sequence. Then we introduce the Prompt-Guided Adaptive Fusion (PAF) module, which utilizes mask prompts to guide the model’s attention towards the dynamically active region at both global and local levels. Subsequently, we incorporate the ST-Transformer to delve deeply into the spatial fine-grained representation and long-term temporal dependency properties of UEX behaviors. Experimental results demonstrate that our PLFormer achieves state-of-the-art performance on an ICU monitory dataset.
更多查看译文
关键词
Unplanned Extubation,Prompt Learning,Large Vision Model,Fine-grained,Transformer
AI 理解论文
溯源树
样例
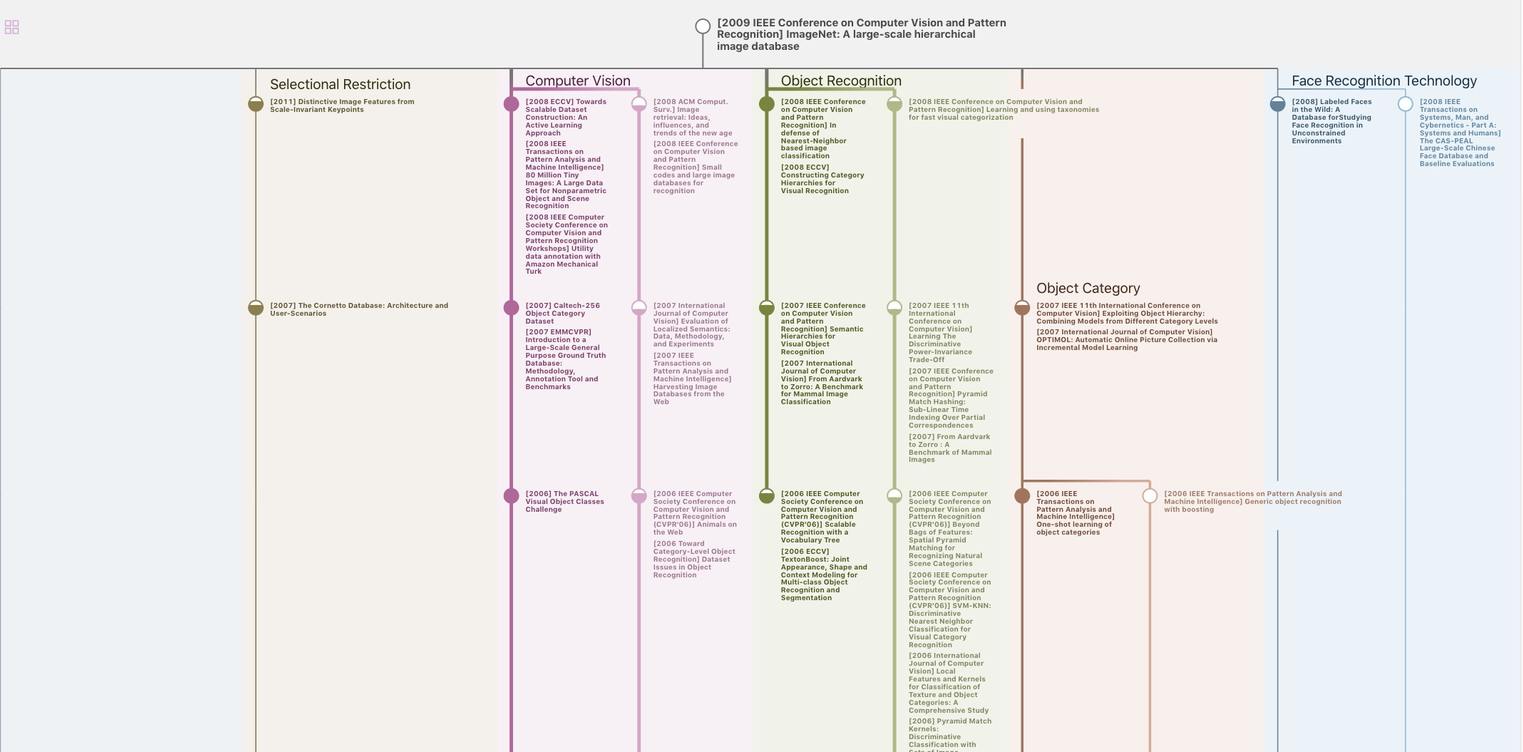
生成溯源树,研究论文发展脉络
Chat Paper
正在生成论文摘要