Joint Learning-based Multiple Documents Heterogeneous Graph Inference for Biomedical Entity Linking.
2023 IEEE International Conference on Bioinformatics and Biomedicine (BIBM)(2023)
摘要
Biomedical Entity Linking(BEL) is the task of linking biomedical mentions in natural language corpora such as diseases and drugs to standard entities in a given knowledge base. The same biomedical entity can have multiple mentions, including synonyms, morphological variations and names with different word order. Thus, making predictions for each mention with an insufficient context in a single biomedical document is challenging, especially when mentions or linked entities are unseen during training. This paper proposes an inference method for biomedical entity linking based on the heterogeneous graph constructed on multiple documents. We first design a joint representation learning method and compute mention-mention and mention-entity similarity in a unified semantic space. Based on them, we utilize the mutual nearest neighbors relationship between mentions and the relationship between mention and entity to construct a heterogeneous graph. Finally, we apply a clustering-based method to make linking predictions, which associates each mention in the documents with a unique entity. Extensive experiments on three biomedical entity linking benchmarks (MedMentions, BC5CDR and NCBI) demonstrate that our method outperforms other state-of-the-art entity linking models.
更多查看译文
关键词
Biomedical Entity linking,Heterogeneous Graph,Iterative training,Minimum Spanning Forest
AI 理解论文
溯源树
样例
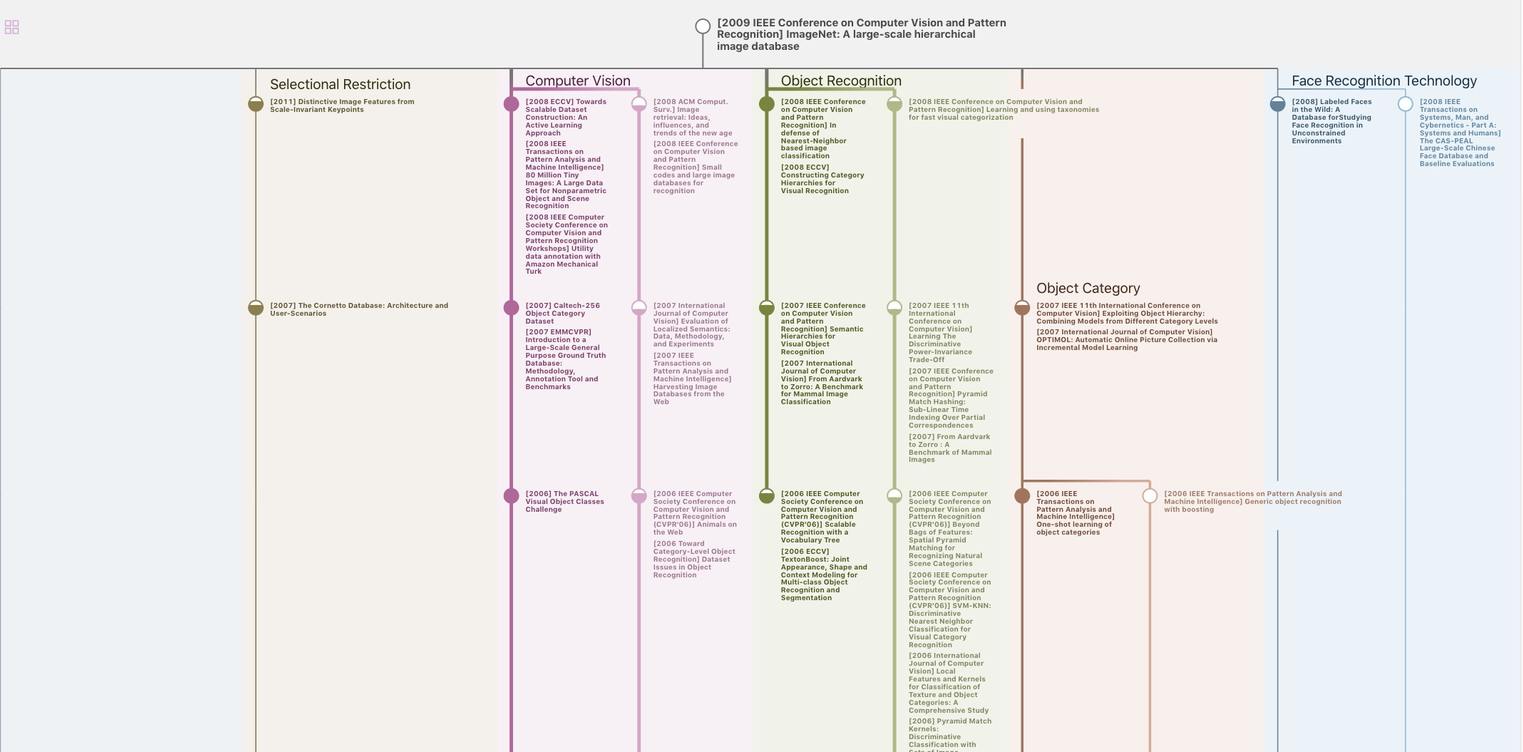
生成溯源树,研究论文发展脉络
Chat Paper
正在生成论文摘要