ConTNet: cross attention convolution and transformer for aneurysm image segmentation.
2023 IEEE International Conference on Bioinformatics and Biomedicine (BIBM)(2023)
Abstract
In recent years, Convolutional Neural Neural Networks (CNNs) and Transformer architectures have significantly advanced the field of medical image segmentation. Since CNNs can only obtain effective local feature representations, there is difficulty in establishing long-range dependencies. However, Transformer has gained extensive attention from researchers due to its powerful global context modeling capability. Therefore, to integrate the advantages of the two architectures, we propose a network of ConTNet that can combine local and global information, consisting of two parallel encoders, namely, the Transformer and the CNN encoder. The CNN encoder is a stack of deep convolution and Criss-cross attention module (CCAM), which aims to acquire local features while strengthening the connection with the surrounding pixel points. In addition, two different forms of features are fused and fed into the encoder to ensure semantic consistency. Extensive experiments on the aneurysm and polyp segmentation datasets demonstrate that ConTNet performs better due to other state-of-the-art methods.
MoreTranslated text
Key words
CNN,Transformer,Criss-cross attention module,Medical image segmentation
AI Read Science
Must-Reading Tree
Example
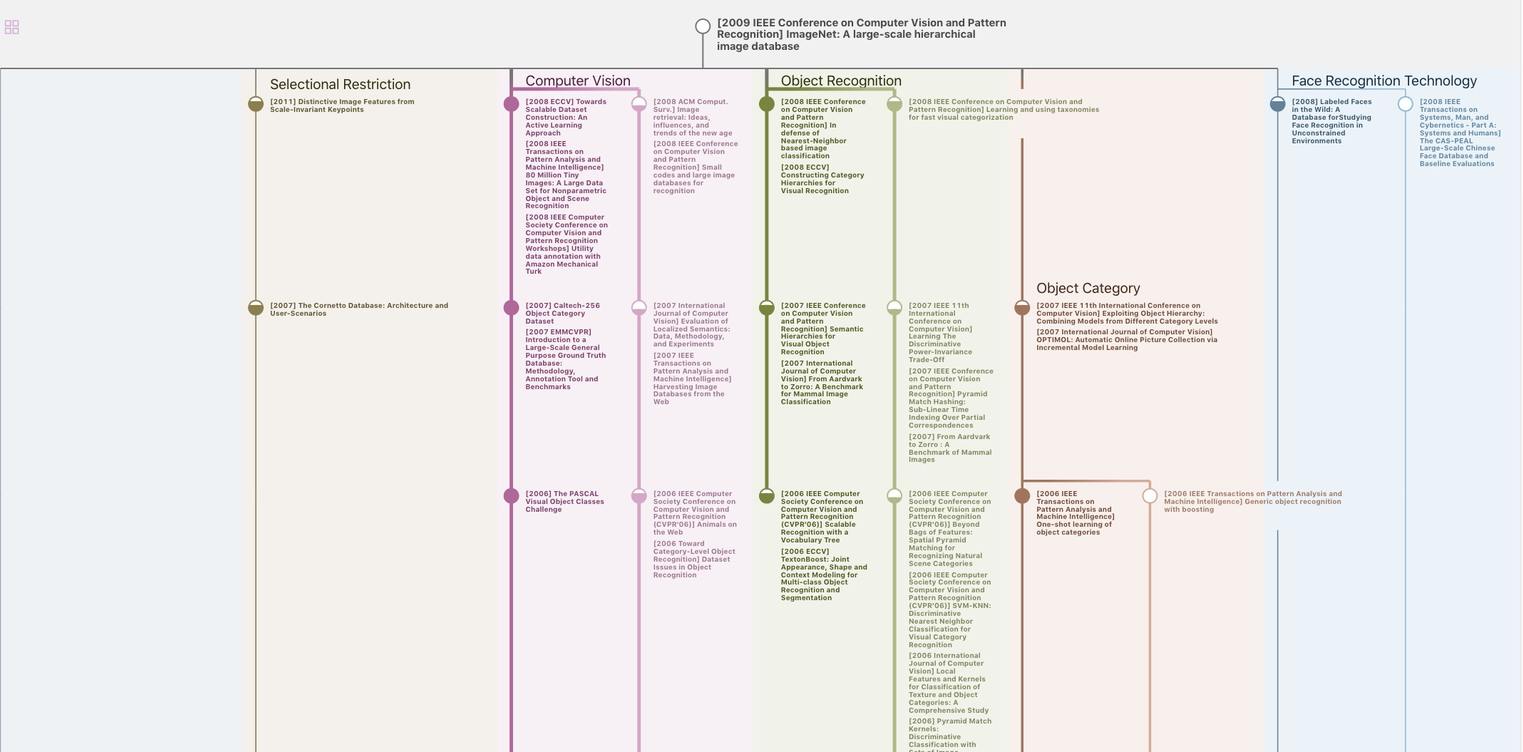
Generate MRT to find the research sequence of this paper
Chat Paper
Summary is being generated by the instructions you defined