Decoupling MIL Transformer-based Network for Weakly Supervised Polyp Detection.
2023 IEEE International Conference on Bioinformatics and Biomedicine (BIBM)(2023)
摘要
Colonoscopy has emerged as a crucial examination for early colorectal cancer (CRC) diagnosis. Early detection of polyps can significantly enhance the survival rate of colorectal cancer. Most recent weakly supervised methods for detecting polyps are based on multiple instance learning (MIL), which employs labeled training data at the video-level (bag-level) to identify polyps at the frame-level (instance-level). However, existing methods often use the same features without considering the differences of video and snippet. Video classification usually focuses more on global features, while snippet classification relies more on leveraging multi-granularity detail information. This paper proposes decoupling the MIL network into the feature encoder and instance decoder. Furthermore, we introduce a novel Snippet-wise Cross Fusion Attention (SCA) that captures rich temporal context semantic features for instance classification. Additionally, our approach incorporates a parameter-efficient finetuning architecture called convolutional adapters, which aims to enhance the training process stability and improve the model's performance. Experimental results demonstrate consistent improvements over state-of-the-art methods on a newly introduced large-scale colonoscopy video dataset by a considerable 7.9% AUC and 1.16% AP. Our code and dataset will be made publicly available at: https://github.com/kanydao/Decoupling-MIL.
更多查看译文
关键词
Polyp Detection,Weakly-supervised Learning,Feature decoupling,Video Anomaly Detection
AI 理解论文
溯源树
样例
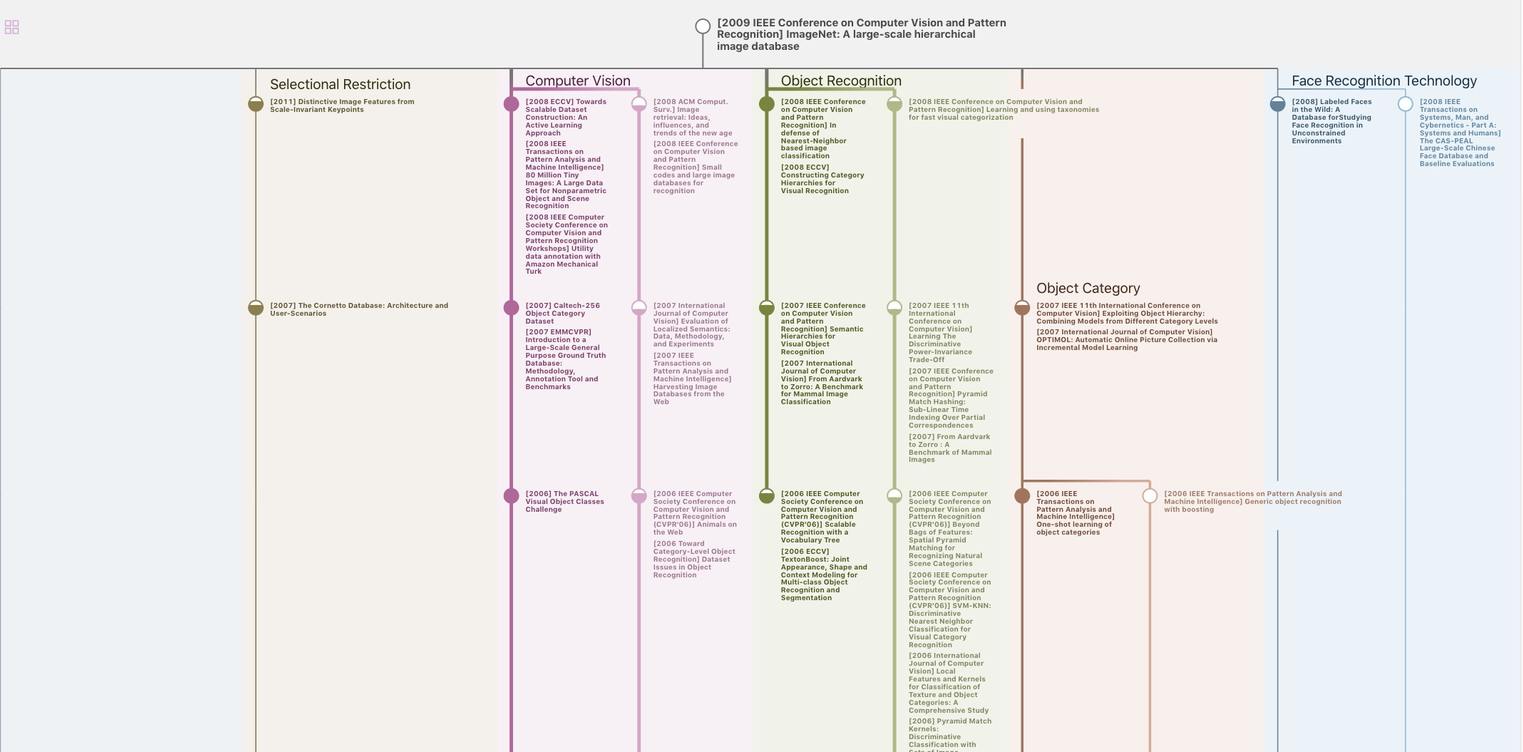
生成溯源树,研究论文发展脉络
Chat Paper
正在生成论文摘要