A joint CNN-GNN framework for early diagnosis of AD using multi-source multi-modal data.
2023 IEEE International Conference on Bioinformatics and Biomedicine (BIBM)(2023)
Abstract
With the abundance of medical data, computer-aided AD diagnosis using multi-source and multi-modal data is a hotspot and trend in research, which brings more possibilities for the realization of accurate assessment of cognitive impairment diseases. Currently, the AD diagnosis based on convolutional neural network (CNN) is still the main method. But clinically, part of data exists in non-imaging form, which makes CNNs have a lot of challenges in fusing imaging and non-imaging. Graph neural network (GNN), which extends classical CNN to non-Euclidean space by using graph topology, affords better flexibility for multi-modal data integration. In order to realize AD diagnosis based on multi-source and multi-modality data, this work takes the advantage of CNN in acquiring image features, and further combines image features and non-imaging information via GNN, proposed a joint CNN-GNN diagnostic framework. Through ablation experiments, we further analyzed the effects of MMSE score, and Apoe4 genotype on AD diagnosis on the basis of image and could provide clinical reference. In addition, our proposed method achieved further improvement in diagnostic performance.
MoreTranslated text
Key words
AD diagnosis,CNN-GNN framework,multisource multi-modal data
AI Read Science
Must-Reading Tree
Example
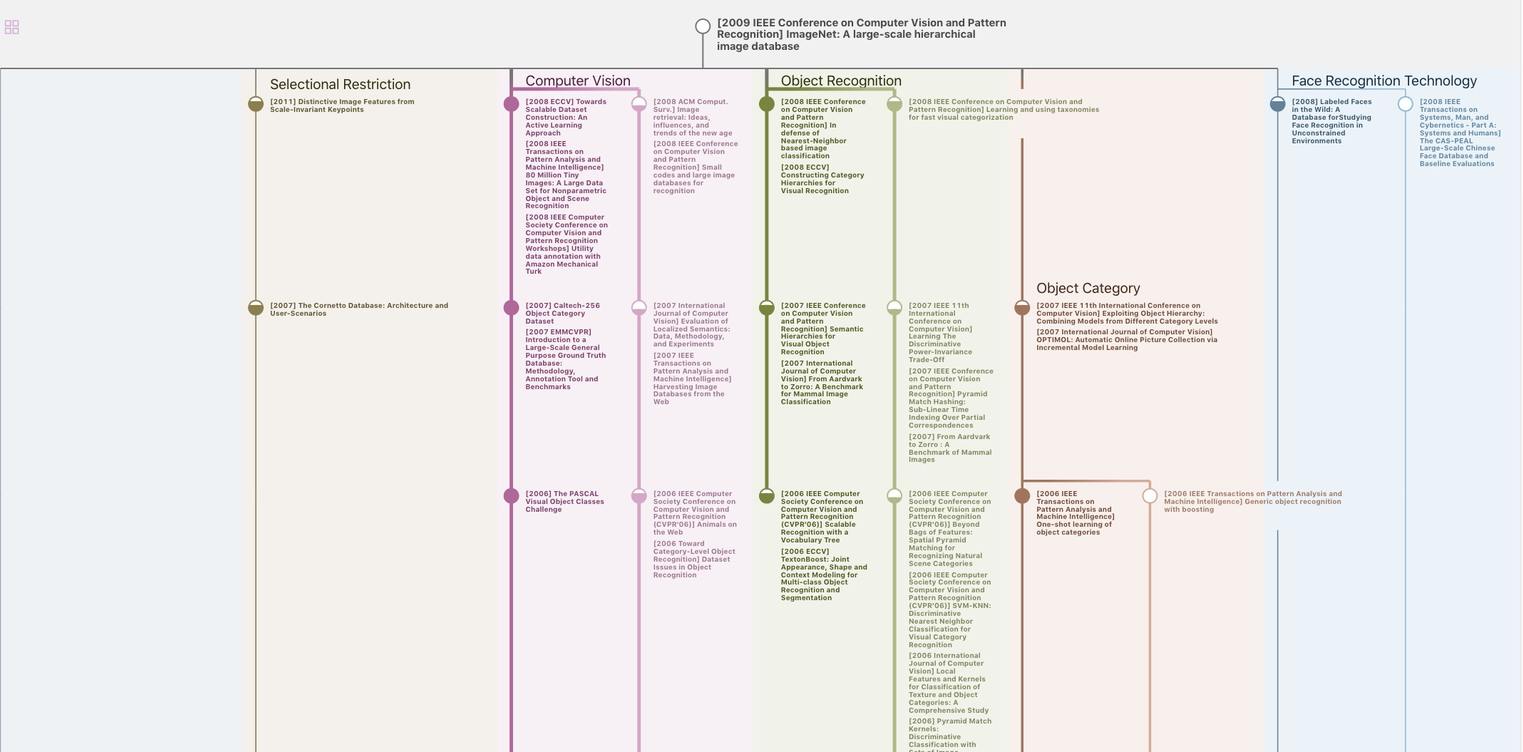
Generate MRT to find the research sequence of this paper
Chat Paper
Summary is being generated by the instructions you defined